The growing value of data has been broadly known for some time, yet many organizations still struggle to build and evolve their capabilities, and services, to utilize it fully. These challenges are compounded by mounting business needs and expectations, and tighter competition within industry sectors.
Data operations (DataOps) is therefore becoming much more than just “lights on,” it is foundational to driving evergreen business value. Reimagining your DataOps strategy is a critical component of defining and delivering your organization's enterprise data strategy, and while companies have customized their individual operations approach, certain factors remain consistent across the most successful insight-driven organizations. Their approach to DataOps is driven by a clear and actionable mission, a value-oriented governance framework, and a comprehensive suite of enabling capabilities that drive high quality and high value execution.
Watch this session to hear how reimagined DataOps is a critical success factor to unlocking the full value of your data-driven business strategies, and how new technologies and shifting operating models are transforming the delivery of DataOps services.
Transcript:
Joe: Now we're going to cover, reimagining and optimizing data operations to drive evergreen business. Okay. This is going to be co presented by Dominic Rasini, Senior Manager of strategy and analytics at Deloitte and Prakul Sharma, Managing Director of DevOps and Practice Lead at Deloitte. Please welcome our presenters to stage.
Prakul: Hi, Joe, thank you so much. It's glad to be here. Dom and I are ready to go. Let's get started. Perfect, quick round of introductions from our side. Prakul Sharma, Managing Director at Deloitte and have worked across, you know, advise, implement and operate across data analytics platform for some of our fortune 500 clients, understood their strategy, their implementation requirements, and more importantly, their data ops requirements and help them solve various problems. I also lead the data ops practice for Deloitte. And our mission there is to really help our clients reimagine and reorient their data ops to the latest and greatest in how do you actually drive evergreen value from data. So we'll talk about that today. And I'll hand over to Dom to introduce himself. And we'll get started.
Dominic: Thank you Prakul. I'm Dominic Rasini, I have over 15 years of experience in data analytics transformation. And I focus on embedding both the functional and technical capabilities that allow organizations to become more insight driven by being data informed, and making decisions that optimize the use of data embedded within their operations and their strategy. So with that, I'll move on to kind of the crux of what we're going to talk about over the course of the next 25 minutes or so I think that it's widely understood that data is the new gold. It has been for a little while.
And by that I think, you know, all of the attendees on this presentation could probably agree that means that data should be viewed as an asset for any enterprise for any organization. It also means that there's an expected return from that asset that businesses can leverage by utilizing data to have better decision making as input into business operations and as a way of influencing strategy. So what does that actually mean? I think that these are some classic ways in which data has been used in the past and continue to be used just to anchor us on an expectation on how enterprises use their data.
So enterprise performance has been a classic one for many, many years. Enterprise engagement and the way that data is used in operations is just as important. We're starting to see and have been utilizing data more in terms of how you engage with customers, how you opt to optimize supply chain route through predictive modeling, and all those types of assets that drive predictability and increase likelihood and probabilistic scenarios that influence operations. And then finally, as a key input into business strategy, hypothesis driven enterprise and business unit strategy, not just as a way of influencing the strategy, but also as a way of evaluating whether or not those initial strategic decisions were effective and correct. And all of this is enabled by cohesive data operations. That's why Prakul and I are talking to you today.
That's why this is a data operation centric conversation. But there are challenges that exist in realizing that cohesive data operations and I think that for the audience on the call, it's probable that you have experienced one or all of these and including a plethora of others about how these challenges exist. And these challenges exist because of the fact that that cohesive data operations is probably not in place, or you're experiencing some speed bumps in trying to get it in place. What do we mean by cohesive, it needs to be integrated this matching of the business and technical sides of how data is managed and utilized within the organization. It has to be value driven, associated with business use cases aligned with something that the business feels is important and will drive future value. And then finally, thinking about intelligent operations. How do you use next gen technologies? How do you use innovative approaches to engineering to increase things like resiliency, availability and the trust that actually exists between the business and technology groups in utilizing data to inform all of these business activities? With that, I'll pass on to Prakul about what we're seeing in terms of how data operations is changing from prior day to what we're seeing in the future.
Prakul: Yeah. And then we'll lead into how do you actually think about going into this new world, we'll share some of our experiences from the ground, somewhat some of our successful clients have actually done. So hopefully, that's exciting and interesting for you. So maybe go to the next slide Dom. So what was historically data ops? Right? Data ops has been here for forever, since the time of the advent of databases and data warehouses. But really, what we've seen is it's been decentralized, a lot of the teams have been operating in a decentralized manner during their own policies, which has created silos, in the technology that we have built and leverage and focused on has been very much, you know, how do we process the data? How do we kind of, you know, scale the processing of the data, but rather, it hasn't focused on really how do you make the data much more integral? Like, how do you actually inject that resiliency into data, not just from a processing perspective, but also from a quality and the trustworthiness of that data? And ultimately, the talent? A lot of the data teams that we see in organizations are very much tech focus, right? How do you kind of evolve that team? So those are the trends we are seeing now into the future.
And the future that we are seeing is basically focused on three key things, right? One is learning how we're linking the data to the business value, how do you do that? You do it by, you know, defining the particular domains. In the last session, we talked about the data mesh and the idea of data as a product, right? Doing that, identifying the business metadata, linking that to technical metadata, and eventually defining ownerships around that and using that to drive business value. So that whole value chain helps you kind of link the data and its outcome to the business value, intelligent operations, that's what I was mentioning, a lot of technologies have evolved. Now, there are specific areas like data observability, you know, instrumentation of the various pipelines to collect the data, so that their operations become much more efficient. You might have heard of self healing data pipelines, those are examples of intelligent data operations. And ultimately, you know, it doesn't happen all automatically as much as we want.
So you do need operating models, service models that use the right talent. And this is where, you know, the DevOps and engagement of both business and technical folks have come into the place to really drive this evergreen value. Next slide, please. So there is absolutely a case for, you know, rethinking data of strategy, and in the light of how do you drive that evergreen business value? And these are just some of the examples, I'm sure, you know, there are more. But things like you know, if you're thinking about really, how do I link my data, and business, and you might have seen a lot of investments in the modern data platforms, migrations to cloud, but still facing the same kind of challenges. There is a need to rethink so data ops, because that's at the heart of how do you drive the value. Intelligent operations, I already mentioned, multiple tools, multiple approaches are there out there in the market that we can now leverage to not only work on the modernized platforms, which is more easier, relatively easier, but also some that actually can work on some of the legacy to actually help you drive some more automation into the whole data processing ecosystem.
And then from a service delivery perspective, DevOps. And another important trend that we are seeing is really the operationalization of the data governance principles, actually into the fabric of the data platform. This is by automating all those policies and procedures, so that you know, things actually start to govern and manage themselves versus us having an army of people doing data governance. Next slide, please. So how do we think about this? How do you pivot to this? The new way of thinking on data ops right. We tried to synthesize what we have seen across our clients and what they are particularly doing? Dom and I will walk through those things. But there is a need for a data ops strategy. And that strategy actually has three components based on our analysis and based on all the work that we have done in the market. The first one is obviously the mission. Second is enablement, which includes operating model and technology. And then ultimately, the governance side. We'll go through each of these, but I'll let Dom start with the mission and how does that relate today.
Dominic: Yeah, thanks Prakul. It's just like I come off mute. So as Prakul mentioned the data Ops strategy has been something that we're utilizing more and more in our conversations with our clients and the data Ops strategy is an integral part of the overall enterprise data strategy that our clients have been defining. I'll talk about the mission. And the mission is primarily broken up into three key sections that produce that cohesive, integrated model of approaching data as that asset as the new gold. The first one we'll talk about is priorities. And this is where if you are going to look for data as a value creating asset, identifying value and business use case alignment as the key reason behind success as the key driver behind successful data ops and investing in data ops, the rest kind of takes care of itself. IT departments as an enabler of business activity is an integral part of the thinking behind how you make that a priority. And IT departments become a key partner in business units in the overall enterprise, realizing their strategic initiatives and their strategic objectives. The second is, it's one thing to say that and declare that value is a priority.
It's another thing to actually have some models around how you would evaluate value creation on an ongoing basis. And there's a combination of leading and lagging indicators that would be useful to determine whether or not you're reaching your mandate and delivering against the business expectations. These include both financial and non financial metrics. I think, if we think through the sectors and the industries, there are some pretty clear non financial but highly quantitative metrics that are important to different sectors. And it's this blend of leading and lagging indicators that allow you to think through how your data operations approach is actually facilitating and delivering value to the organization. The final part is what are the guiding principles through which you're going to assemble and mobilize your data operations approach? I think that Prakul mentioned a few of them, embedding a DevOps mentality, embedding a product oriented approach to data instead of a more legacy, monolithic project type approach, our two classic ones that we see and discuss with our clients at the moment.
But there's also some behavioral adjustments in terms of guiding principles that are valuable. Incubating a concept of innovation across your organization, adopting a fail fast mentality, and building structures and service delivery models that allow for flexibility and scalability, rather than a very, very acute focus on one or two things that are inflexible and reduce the ability to react to changing business needs. The one thing that I actually wanted to spend a little bit more time on is the business priorities and how this ties to value. Typically, what we see in our clients is that strategic priorities are based on one of three things, an offensive strategy, a defensive strategy or a disruptive strategy. Your offensive strategy is typically related to revenue driving strategies, this could involve approaches to customer engagement, something that is ultimately going to drive changes to the way that your customers interact with your business, increase the number of transactions increase the average transaction size, these are offensive strategies and data as an integral part of meeting those strategies.
Defensive strategies are your classic. excuse me, I have a bit of a cough at the moment, defensive strategies are your classic cost centric strategies, a lot of supply chain manufacturing optimization strategies are anchored in this defensive strategy. And data has been an integral part of that for some time. But we're starting to see new use cases come up about how companies optimize and meet those strategic goals. And your disruptive strategy include ways in which data is starting to be utilized more as an asset for monetization purposes or you're ultimately using data for new product development, expansive opportunities. So aligning your data operations to how your organization mobilizes around offensive defensive or disruptive strategies is an integral part of how you'll move forward and allow data operations to be an enabler of that business execution.
Prakul: Perfect, thank you, Dom. And then as Dom mentioned, so mission defines that vision, that goal on how data ops should be used, but there are some underlying foundations that are needed to be in place. Enablement, being the first one, maybe go to the next slide, please.
So I'm sure, from an, you might have seen this picture or a version of this picture. I mean, it's nothing surprising. In any operating model that we've seen for a Data organization, there are usually two sides of the puzzle. One is obviously the core operations, all of the incident management to enhancements to, you know, infrastructure management, all of those pieces are there. But then you also have the core dev teams that are working through. However, what we are seeing is an evolution on how this model is actually mobilized in various organizations. Successful organizations are actually focused on driving this ecosystem across these five tenets that you see on the left side of the slide. This also links back to some of the latest and greatest thinking that is out there in the market, data mesh enabled data ecosystems is one.
First of all, it's really thinking of data as a product, rather than just data set, right? And that actually enforces not an organizational perspective to it, who owns it, how you actually enable the consumption of that data to the right people, all of those things come into the picture over here. The second is really, you know, as you're developing any of this data ecosystems are modernizing the platforms, how do you instill that culture of innovation and automation, you know, whether you're using metadata driven frameworks to orchestrate all of your pipelines, rather than developing each and every ETL yourself, and how those then actually instrument, the observability part of it, and how do you then use that observability to, you know, sell, feel and utilize those advanced approaches for data ops, management. Demand management, this is where the business and the data teams actually come together to make sure the demand is prioritized and driven in the right way.
And then a related aspect to it is the DevOps, which is, there are versions of it, there is pure DevOps for our software engineering folks. But then there's a variation of how data DevOps works, which has a lot of stress on how the data integrity and testing comes into the play. And ultimately, the governance by design, I made a comment earlier that, you know, governance needs to be injected into the actual fabric of your data platforms. And that's very essential, because I think the trust in the data is inherent in some of the governance and management processes that need to be there. Next slide, please. The other aspect of our enablement is also technology and closely related to that is the governance. The reason we are discussing those together is because they need to link together equally. What you see over here, I'll start with the right side of this slide are some of the feature sets that we are seeing when somebody is really thinking about an advanced data ecosystems from a data ops perspective.
So obviously, the very first thing is a complete control over your data ecosystem. So that you have you can control various aspects from orchestration to consumption, through an integrated approach, yes, there are going to be federated implementations of those, but there are certain standards that bring all these things together, Visibility, 360 visibility, which is not only at a technical level or an operational level, but also at a business level. So how does that data that that you are producing and delivering fulfill certain business requirements and the people who are actually at the end consuming who are really the business folks, how do they understand of understand certain events that are occurring in your ecosystems, and then that impact that end business outcomes?
A related one to that, as, you know, the transparency into data processing and execution, as well as also the end to end management of data governance and ecosystem that's where the concepts like trusted sources, management, data contracts, and how those are moving and how those are automated into the ecosystems come into the play. And ultimately, but last thing I'll put together is the business metadata. The business metadata is key to driving that business value as I was earlier mentioning and the linkage of that business maker to your technical and operational metadata is key.
We have seen clients actually develop certain modern ontologies or models that actually link these two different areas have metadata together and are able to then utilize not just to operate the data, but also to drive visibility from a business perspective. What do you see on the left side here says how some of those feature sets that earlier mentioned, get mobilized? So there are different sorts of capability areas that we have seen, that are instrumented or integrated into your core data ecosystems, which you see on the top and bottom of that data ecosystem pipeline. Let's move to the next slide. And maybe Dom, you can talk about how do you think about data strategy and what are the core components that really are required to mobilize that?
Dominic: Yeah, thanks Prakul. And I think that I hope the audience's that kind of understood the walkthrough of the conversations that Prakul and I are having with our clients at the moment in this important need to match your data operations with your overall business strategy. But as you think about that data operation strategy, we typically see two types of conversation unfolding. There's either a reimagining conversation around the strategy, or there's an optimization conversation around the data Ops strategy. The reimagining of the strategy often comes from our clients who are still yet to embark upon their modernization journey, they still have a lot of legacy technologies in place, either just through pure size, or they have been able to maintain that legacy architecture for quite some time.
And this reimagining is really coupled with as you start to move to more next gen technologies more scalable, elastic technologies, such as Cloud environments, such as Cloud workbenches, how do you need to reimagine that data operation strategy to make most effective use of those new technologies, and really leverage the innovative approaches that those technologies enable. The second type of conversation, though, is an optimization strategy. And you can probably guess that this normally comes from our clients who have more of a view of they're either underway with their modernization journey, or they have, you know, pretty much completed what they feel is their first couple of phases of modernization.
And this is where they already know that they need to start making some changes to their data Ops strategy, they've started to implement those introduced new service delivery models possibly scale up different skill sets and different capabilities. But there's still some opportunity to tighten things up, and really build an overall data Ops strategy that is more tightly coupled with business value. So I will leave it there in terms of the two types of conversations that we have, I think the audience can see on the right hand side that those conversations exist around these 10 components. And either through reimagining or through optimization, it's definitely important to consider all of those 10 components holistically, and identify what is right for you as an organization about how you move forward and make that connection between data operations, and business value.
Prakul: Thank you, I think now we can shift to any questions that the audience might have.
Joe: Perfect, so we do have a few questions for you. So the first of which is, and we've heard a lot of questions or discussions around the data mesh? What's the extensibility? Of kind of reimagining your data strategy to kind of fit with that paradigm? Or do you feel that just the principles apply, but it still needs to move a different direction? What kind of your stance?
Prakul: I mean, in fact, actually, we've seen some of this in action at some of our clients, on folks who are reimagining the data Ops strategy. You know, I talked about a few points around, you know, a core tenet into the operating model being, you know, data as a product, right. Data mesh is a way of enabling that data as a product, and how do you then enable that operating model to support? Mesh like architecture, on driving that integration in a consistent way is part and parcel of a data Ops strategy. Same goes for also some of the, the upside, right? Yes, you might have a little bit of federated underlying data ecosystems and architectures across, you know, business units and others. But how do you consistently exchange metadata, right? Whether that is for consistently sharing that metadata or for seeing how that metadata is related from an Ops perspective. Those principles are caught and parcel of the framework that we've seen, implement and be successful at our clients. Dom, anything to add?
Dominic: I think the prevailing point that I would say Prakul is that product mentality is a behavioral shift, and an attitudinal shift. And once organizations start to embrace that mentality, a lot of things start to fall into place. So I just keep indexing on that data as a product manager, as a product, viewed as a product mobilized around as a product is such an important part of successful ongoing operational execution.
Joe: That's a great thought, Dominic. And that was kind of one of my next questions is, what are the dominoes to fall? In what order? Do you usually see them fall in? Right? Usually that shift in mentality of like, okay, we want to go for data as a product. Right. But what's the next step that people need to be looking at? Are they taking some sort of an assessment of where their maturity is? Are they doing a hard look at their current data operations? Sorry, their data strategy? Are they asking that question? Am I looking at to reimagine or reinvent, right? What's that next step after they've made that shift that we want to go there?
Dominic: I think the thing that I will index on and there's multiple ways in which you could slice this in terms of actual activity. But it's also it's conceptual is embracing the understanding that mobilizing around data requires a cohesive, multidisciplinary approach. And this ties in, you know, the economic value that you can expect from data requires some sort of financial assessment. So bringing in financial analysts to say, well, like if we were to use this an anchor, business or strategic goal on this, this is the value that we would expect from that. Sometimes that's too distant from the technical side of things to really make those arguments.
The second side is what does the business actually need and how much of a partnering or able to partner with IT, to be able to gain access to the data that they need on a timely basis with the level of quality, the level of availability that is required. And then this starts to inform technical approaches around resiliency, high availability, quality management, so product as a way of treating data, but then the way that humans mobilize around that needs to be a cohesive, multidisciplinary approach. And all of the assessments are driven towards building that uniform view of data and how the service delivery model maintains that cohesion. Prakul, any thoughts from here?
Prakul: Know, I think you've covered. I think the important point is this is a multidisciplinary approach. Right? I think we always go by the people process technology. I think it's a different way of looking at that from a data ecosystem perspective. But they have to be looked at it and together and not in silos.
Joe: Perfect. We'll take one more question. As we have one more minute before our break here. What do you think are the main challenges for data ops in a transformation journey?
Prakul: Yeah. I mean, I could take this question. I think the transformation journey actually provides an opportunity to rethink data ops, as well as you know, all of the new architectures. Interestingly, what we have seen in the market is a lot of our clients have actually taken up the modernization journey, more like a move to cloud versus really rethinking the underlying architecture. And that has its downsides as well, the lift and shift approach only goes so far. The challenges we do see is, you know, like Dom mentioned, it's really a different way of thinking.
And I think a simple example would be when you're really thinking of, you know, thinking of data as a product. It's not only the technical owners of the product, it's also business owners of the product. So how do you bring business into the fold of taking the accountability and ownership of the data? And then how do you are providing the tools and the operating model to actually drive that kind of support structure. So, Joe, that's one of the challenges. There are many challenges we can kind of discuss in this area.
Joe: Perfect. I know there's a few more in chat, you might be able to answer them before we squeak out of this session here. But I do want to say that we are in overdue for a break now. We're gonna go take 15 minutes, and then we're actually going to join back to the keynote stage, where we'll hear from Rohan on a fireside chat. So I hope everyone sticks around and joins us. I want to thank you, Prakul and Dominic, for sharing your insights and expertise. And we'll see you all shortly.
Prakul: Thank you very much.
Dominic: Thank you.
Speakers:
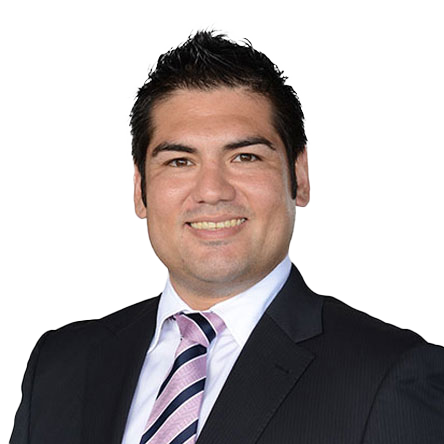
Dominic Rasini
Senior Manager, Strategy and Analytics
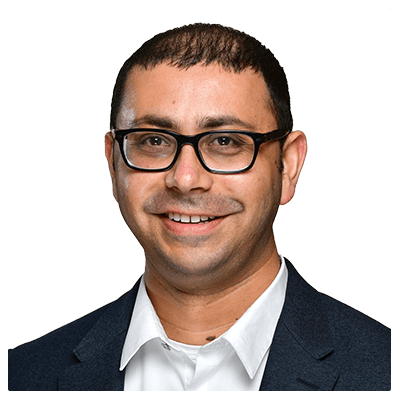
Prakul Sharma
Managing Director, DevOps Practice Lead
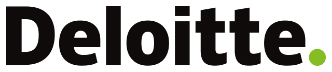