For most organizations, building a data platform is no longer a nice-to-have, but a necessity.
Companies distinguish themselves based on their ability to glean actionable insights from their data to improve the customer experience, increase revenue, or even define their brand.
In this lighting talk, we cover the six must-have layers you need to include in your data platform and the order in which many of the best teams choose to implement them.
Transcript:
In this quick tutorial, we will walk you through why you need a data platform to six must have layers for your data platform, and why you should prioritize data quality when building your data platform. But before I dive in to the rest of the talk, I want to quickly introduce the team here at Monte Carlo. For those of you unfamiliar, Monte Carlo is the creators of the data observability category, founded in 2019, by Barr Moses and Lior Gavish. Before founding Monte Carlo, Barr was the VP of the data platform over at Gainsight and Lior co founded a security company that was acquired by Barracuda Networks. And Monte Carlo, we work with hundreds of data teams across various industries to accelerate the adoption of data by increasing trust in their data platforms through end to end data observability.
We are currently writing the first O'Reilly book on data quality. And the first three chapters are available for free on our website, so go check it out. At its core, data platforms are a central repository for an organization's data. A data platform should handle the collection, cleansing, transformation and application of data to generate business insights. Building the data platform is no longer a nice to have for most data teams. It's in necessity, organizations are distinguishing themselves from their competitors based on their ability to glean actionable insights from their data, whether to improve the customer experience, increase revenue, or even define their brand. When building a data platform. One thing to keep in mind is that there is no one size fits all approach, the right data stack will look vastly different for a 5000 person FinTech company, than it would for a 200 person startup company in the eCommerce Industry. Still, despite the size of your organization, there are six must have layers, every data platform should incorporate in one way or another.
Of course, how you choose to build your platform, and which tools you decide to go with are entirely up to you. Now the list of tools you will see is not every tool that we could possibly list, but these are the ones that are most popular amongst our customers. First, you need an ingestion layer. You can't process store, transform or analyze data unless it enters your data ecosystem. Some popular tools in this space for this are listed on the slide. After building out your ingestion layer, you need a place to store and process your data. The emergence of cloud native data warehouses, lakes and Lake houses have taken over to market offering accessible and affordable options for businesses to store data. Some top some popular tools in this space are listed on the slide. A crucial layer to the modern data platform is the transformation and modeling layer. A few common tools that data engineers utilize to either transform or model their data are listed on the slide here.
The data transformation and modeling layer turns data into something a little more useful, readying it for the next stage in this journey the analytics layer. Some popular BI and analytic solutions for data teams are listed on the slide here. The other two layers which we'll zoom in on shortly our data observability and Data discovery. Data observability is an organization's ability to fully understand the health of the data in their system. It works by applying DevOps observability best practices to eliminate data downtime, with automated monitoring, alerting and triaging to identify and evaluate data quality, and discoverability issues. Data observability leads to help your data pipelines, more productive data teams, and most importantly, happier data consumers.
While we might be bias here, your data observability layers should seamlessly connect to your existing data stack and automatically monitor and alert for the five pillars of data observability, which are freshness, distribution, volume, schema and lineage. Data discovery empowers data teams to trust that their assumptions about data match reality, enabling dynamic discovery and a high degree of reliability across your data infrastructure regardless of which domain. These two layers are the newest layers of the data platform and one set core respond most directly to data quality. While you should invest in both, we find that most data teams choose to prioritize observability first, after all, you don't know what you need to manage before you can measure it. A data discovery approach requires data observability, which can be enforced through automated table and field level lineage to map upstream and downstream dependencies between data assets. Data discovery without severability will prevent teams from achieving a much needed bird's eye view of their data assets across distributed domains.
Speaker:
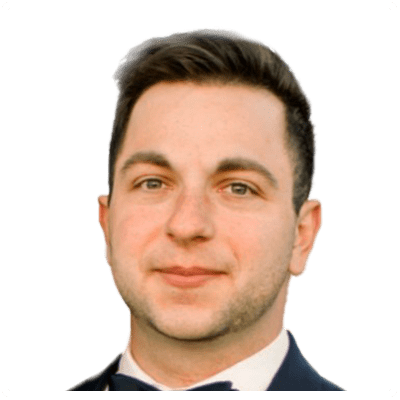
Brandon Gubitosa
Founding Team Member | Monte Carlo