The sharp rise of TV viewing, especially OTT, during the pandemic brought a huge opportunity for marketers to reach new and incremental audiences. Unfortunately, the ability to measure and optimize OTT campaigns had not quite caught up. TV viewership across screens and channels continues to fragment and shift, making it a challenge to deliver cost-effective planning and activation across linear, OTT, and digital platforms.
To solve for this, TV viewership data, from automatic content recognition (ACR) technology in more than 35 million U.S. TV sets that captures every DMA and detects content across sources, demographically representative and IP connected, was explored using Databricks and pyspark.
Watch this session to learn how the Advanced TV solution enables users to get all benefits of programmatic data applied to the most engaging medium. The TV intelligence tool provides multiple insights to enable users to connect data, discover insights, and activate campaigns with efficiency.
Transcript:
Ardeshir: So we're gonna go ahead and jump into our next session now, we have with us today, Bitanshu Das and Likhitha Jagadeesh of MiQ. And there'll be talking about data engineering and advanced TV, tapping into rich deterministic viewership and cross device data. Bitanshu, he is the lead engineer at MiQ managing the data engineering team and responsible for building scalable big data solutions in pragmatic media buying an ad tech space. He loves exploring the data building up optimization of data pipelines, and building data pipelines from scratch. Likhitha has been working as a data engineer at MiQ digital India since 2019. Bitanshu and Likhitha, welcome to zero gravity. And please take it away.
Bitanshu: Hey, everyone, this is Bitanshu. I'm working at the lead data engineer. Thanks for the intro. And with me, I have Likhitha who is a data engineer at MiQ together will present you an interesting title that is like deep data engineering and advanced TV, tapping into the rich deterministic viewing viewership across on cross device data. Yeah, so who are we? We are the marketing intelligence company with the right people and technology. We onboard transform connect a lot of data points, data sources, and we drive discover insights out of these data by a lot of advanced analytics and use these insights to power our marketing campaigns in case you are aware of the ad tech world, we usually come in the category of programmatic agencies. What do we do? We are experts in combining real data and digital data applying, applying marketing intelligence to give our customers the insights that helped him to win. We basically work in three major verticals, media, analytics and tech, we have an award winning programmatic ad campaign management system.
Apart from that, we also provide custom analytic solution and can provide customized scalable solution, unlocking the value of the data that we have at our data lake architecture. Going deep, can we move to the next slide? Yeah, going deep down in our verticals, let's have like a problem statement that we try to solve. So in our media vertical, we basically deal with the programmatic campaign management or taking a basic example, our advertising agency may be launching a new product, and they want to increase the engagement of the product by X Factor within one month. The media vertical at MiQ would start launching the ad campaigns for this, this, then the analytical vertical will leverage this data built solution optimal solution on top of this data, these solutions can be customized or generalized. We provide insights prediction to drive the AD team, ad campaigns, and then finally increasing the engagement as a whole.
A team of business artists and data scientists and data engineers are responsible for maintaining this analytic's vertical, the third vertical comes under technology that is on the Tech, which is technology vertical. It builds and maintain infrastructure platforms solutions, onboard data for both in-house and clients. Moving on to the next slide. So the media vertical that's responsible for driving the ad campaigns. This leverages our award winning programmatic media products and services. Some of these products that you can see on the screen that we have at MiQ are also our USP. These are movements, motion, select predict and finally cast. Cast is the product that we'll be looking at the data engineering architecture, we'll be looking at the detail, and few minutes. All these products that we have developed a unique solutions, they belong to different spaces. They are built with the help of both data engineering and data science solutions as well as analytics, for example, like motion is basically with respect to tracking activities around a location or trying to target users.
Predict is a performance tool, which is basically creating innovative solutions around the technologies that exist, and so that we build the right products and services, and we can target the right customers. Moving to the next slide. So this is a daily scale of data that we handle at MiQ. We ingest almost 33,34 terabytes of data daily. We have been in this field for the last 10 years. In these 10 years, we have served 90 billion plus ad impressions. We have around 750 million users who we target using our targeting platforms, we have developed over 7000 customer acquisition strategies.We daily serve around 2000 Plus live campaigns at any point of time. And if you're belonging to the ad tech industry, then you must be knowing the term like CPU per minutes we are targeting like 1 million CPU per minutes, which is currently at around 900,000 CPUs per minute
Moving on to the next. So I guess you have already listened the line repeated to the death. But it's true. Even it holds in the ad tech industry that data is the new oil. To have our ad campaigns optimized and rightly targeted, we onboard a lot of datasets. Some of these datasets are like bought, some of these are nurtured and built over the time within our organization. We have data sets for like multiple categories that you can see on screen, we have a quality device tracking that has IP management data, there is ISP provider data, there is location data or contextual audience profiling. As I mentioned, some of these data sets are bought through data vendors. Some of these are built over time.
Then there's action based data we have like macro data, which is like weather feed or twitter feed. Overall, at our MiQ, we have around 70 to 75 data sets which we process. We connect these datasets together and build solution. So that all these interconnected data sets are processed together and made sure that we maximize the amount of insight that we can generate out of this. One of the important data sets that we have at MIQ is like PCR data sets, which we'll dig deep into in the further slides. Moving on. So digging deeper into TV, like I guess most of us have already have some kind of subscription. And even if we didn't have it before 2020, most of us have taken some kind of Netflix, Amazon Prime subscription during the pandemic. So OTT was one of the major gainers in the pandemic year with the user base expanding multiple times, like. Like OTT has actually changed the view TV viewership, how we view TV.
And according along with the change in viewership of the TV, the ad, there has been also a change in viewing pattern of the ads also. In case of traditional data or traditional TV, what you had is like one ad would be running for everyone, but with the rise of OTT like more personalized ads can be repeated. So for example, you are watching a football match and you are watching some Lay's chips, but you can't you don't find like Lay's taste. Tasty maybe. So when you open the phone, you can see some we had browsed some other healthier options and keto diet options. And you found find that keto diet that you had searched for in a phone via an ad, and that's like how connected devices work. So even in 2020, like we started, even in 2020, like at the beginning of pandemic, the OTT ad tech market was around $8.88 billion, but utilizing the budget correctly was a challenge.
So these are the challenges that you're seeing on the screen. Similarly, there were concerns regarding the performance tracking and attribution of these ad campaigns. 27% of marketers didn't know how to track their ad campaigns. 24% of the marketers are unaware of what was the right strategy for OTT based targeting and the OTT adtech space was relatively untapped. To overcome all these challenges, we built our custom TV solution. Moving to next. Our TV solution is data driven, its partner agnostic and curated supply. So what does this mean? Our advanced TV solution is actually heavily dependent on data science and data engineering solutions. These solutions includes connecting multiple datasets with millions of TV viewership data, deriving the most out of these insights out of these connected datasets. Our partner agnostic here refers to like ACR data vendors. ACR means automatic content recognition.
This is the data type that we deal with and we'll have a much detailed look into in our coming slides. We do detailed analysis of data and then we decide to onboard the correct data partners. Some of the partners includes like Vizio, LG, samba. So depending on the requirements of the markets, depending on the geo where the data is, which data part platform is data sources more beneficial for us. We make onboard these kinds of data and hence we are partner agnostic, we are not related to any one single partner, we are always open to onboard new partners.
The third is like curated supply. Curated supply actually refers to the different customized solution for our clients, we don't only provide the generic standard solution, We also provide customized solution that can be both insights and activation as per the needs. The customer solution can also vary on Geo based data and demographics data also. Moving on to the next slide. So these on the screen you can see the five core offerings of our verticals, this means like connect, discover, activate, optimize, and measure. So connect actually what here means that we are able to connect multiple ECR datasets together, within and across multiple Geos to get the maximum reach and representation of our data set. We have built in pipelines to smoothly process connect multiple data source, multiple ECR data feeds, both batch and real time processing. In discover, we actually have an in-house planning and Insights tool to create like customized solution, standardized solution based on our different onboarded clients.
Activation offering in the TVs industry is actually a unique solution. It's very new. We tapped this market on two years back. And now we can process and activate both batch and real time data across multiple DSPs like we have Xandr DV 360 DtD in Yahoo. Optimize over here refers to the performance of DSP optimization like there is a dedicated team for TV ad campaign optimization. This team looks into private verification, inventory management being an and we are an outlier in terms of our Espeon flexibility, the clients have the ability to set up an automated campaign just with a click of a button. So how automated our solution is. Coming to the final vertical, that is measure. In ad tech industry, like measuring and tracking of our metrics is very critical. Because attribution is one thing that proper attribution of your tools is very important and we use various attribution tools in the market, we combine these data from these tools and to calculate the metrics like reach, frequency.
Moving on to the next slide. So this actually shows the reach of our ACR data sets we have like ACR data set across 35 million USD visit, we cover like every DMA, we are able to detect content from any soul that is, could be it cable TV, be it OTT, be it gaming console, we represent every demographic that data sources, which covers every demographic, and the diseases can be matched both to first party and third party data sets via IP. Here, you can also see a brief view of the tools that we have in the screen. Next slide. So the ACR data actually is like automatic content recognition, it can identify the content played on a smart TV. So that's kind of like pixel mapping technology that is being used, where you can map the pixels and find out exactly what content is being played on the TV.
So MIQ actually buys the data from the data provider, the data provider that we have already mentioned before, like Vizio, samba, LG, we ingest into our Lakers architecture. And then this ACL data is processed and used to build our solution. We ingest 250 GB plus GB data daily. These data like can be that can be content related data, that can be the advertisement shown on the TV, that kind of data or that can be a audience profiling or demographic data. Next slide. So what was the goals to offer advanced TV solution. So first was like we want to process this ACR data and make sure that they are connected with the other datasets already available to derive more insights out of this.
Another challenge that we wanted to solve was that to fetch touch YouTube Insights from DV 360. One challenge that was associated with YouTube Insights was that YouTube is a closed, like provide to bring the data out of their infrastructure, so we had to rely on both GCP and our in-house data as well. The third is like, we wanted to target the relevant audience, because activation is not very common across the industry right now. And hence, the targeting was an issue that we want to wanted to solve. So moving on, we'll have a more like more detailed understanding of the data pipeline architecture and the process associated with it. So yeah, handing over to Likhitha.
Likhitha: We store the data in Amazon s3 in lakehouse architecture. So we ingest all the DSP data that is the Tradedesk and AppNexus Data in s3 locations, and also we ingest the ACR data that is a third party data and also in the s3 location. So once the data ingestion is completed, we move on to the data processing step where we you know, process the data on data bricks, which is built on top of Apache Spark. Before starting the ETL operations, we do have data check and alert the step where we are. In the first step we are checking if the data for a particular day has arrived or not. So once this condition is satisfied, we move on to the second step where we are checking the data health, so, if we will be checking if the current date data size is at least average of at least 70% of the average of last week's data. So, if these conditions are not satisfied, we will be sending out an alert to the respective teams who are using this data.
And in the next step we will be generating the aggregated data and storing the data in s3 location and this is used for generating both insights as well as analytics. In insights we will be developing dashboards and in analytics we will be targeting the audiences. Coming to the data processing part. So, this step usually involves joining an aggregation of multiple or you know, data sets, whether it's a ACR data with the demographics data in order to generate, you know, all the demographic insights like ethnicity, gender, and income and everything and also joining the ACR data with the DSP data to find out the users in order for targeting purposes. So, coming to the YouTube Insights, one of the challenging step that we face here was you know, as Bitanshu already mentioned, so, the YouTube data which is provided by DV 360 is available only on GCP and not on any other cloud platform and also a one more challenges the cost of data transfer from GCP to s3 or s3 to GCP is very high.
So, in order to overcome these two challenges, what we are doing is first in the first step, what we are doing is we are joining our ACR data with the DSP data and that we will be joining only the data for which the users have requested. So, in the first step only like we are filtering the data for the requested brands and this aggregated data we will be moving this to moving this from s3 to GCP. So, once we move this data to GCP, we will be joining this same with YouTube Insights, which is available on GCP. And once we join these data, we will move back the data from GCP to s3. So, by this way, one, one part of the data processing is done on GCP and other part is done on AWS. So, this way, like there is less data being transferred and also the YouTube Insights are now available on s3. So, there are several aggregated cards, which are you know, generated using multiple metrics and dimensions. And all of these data will be powering the insights which are which is available on Tableau dashboard. Going on to the next slide of TV intelligence insights.
So for the TV intelligence insights, we are using ACR data and joining it with the demo data and the admitted data. So from the demo data, we can get ethnicity, age, gender, you know, and income related information. And from ad data, we can check, you know, the performance of the ads when the ad was fostered when the ad was last year, and also the video links as well as other details with respect to the ads. So, once we you know join all these data sets and then create the aggregated data this will be powering the TVI dashboard. So, this is one of the examples as you can see here like we can see the split between you know linear TV and the OTT part. And we can also see you know, what are the top ads which will performing better and then which serve multiple, you know, impressions and how many households the ads reached. And we can also see the demographics as well as the geographic data along with you know, the top shows and channels for linear TV as well as the top apps and devices for OTT.
So, coming to the activation part. So we have two types of activation as Bitanshu already mentioned. So one is the real time activation and another one is the batch activation. So here we will be using IP addresses of the devices and cookies of the websites in order to target the audiences. So for batch activation, we use the ACR batch data and process this data on data bricks. So I'm for our real time activation we stream the ACR data directly on to Amazon EC2 and run the pipeline on a sequel. So we chose EC2 because of the size of the because the data size of RTA is like very less and we usually need like you know 24/7 running cluster for the real time activation.
So that's why we chose EC2 and in last step, whatever the IP addresses and cookies that we got, so, we will be using them and hitting the API's of these DSPs that is theTradeDesk, App Nexus and Yahoo. So, we hit these API's with the respective you know IP addresses and website cookies in order to target the audiences. Okay, coming to the data preparation challenges that we faced. So, initially, when you know the number of brands or the number of requests that we got from the users were like, very low, we had not seen any issues in the pipeline, this was because like, you know, most of the data was filtered out during the first step itself, but as a number of brands request like increased exponentially, we started facing you know, many scaling problems and high runtime and also had multiple you know, pipeline failures. And due to this, we had to do you know, multiple back fillings as well. And due to the processing of massive data, it also resulted in you know, cause spike and also in high runtime initially the pipeline you should take around three to fours, but then once the brands started increasing rapidly, the runtime went upto 9 to 10 hours.
So, coming to the big data optimization steps that we took, so, the first and foremost step is to you know, get the basics right, which means, whether it's data set, we have to you know, include we included a pre processing step whenever we are using you know, large data sets. So, we filtered for the required columns and brands only and stored this pre processed data on an s3 location. And whenever we are whenever we were joining, we made sure that the other table has only you know, unique values for a given key level, otherwise, it will result in a large data set. And one more option, one more thing we did was using broadcast join. So, whenever we were joining any lookups we used broadcast join, and this resulted in like less server time and selection of instance type of the cluster is also very important, because, initially since the data size was like very huge, we thought of going with memory based cluster, but when we debug the logs, we found out that most of the memory was not utilized and instead of memory based, we needed to have you know, the compute based cluster.
So, we shifted from memory base to compute based and that resulted in like lesser run time. And, one more thing is we also truncated a long DAGs. As you know, it was very difficult to pinpoint, or debug ways the issue is happening. So, we started storing the intermediate data on s3 location, so that we have a lesser or like shorter DAGs and it's very easier to debug. Coming to tweaking the shuffle partition size, it's like, one of the most important thing sometimes if the data size is more and if the sometimes if the shuffle partition size is more it results in like more number of smaller files, and like, there will be more files, but with the small smaller size and also, maybe some of the smaller, some of the, like, few files with huge datasets. So we tweak the partition as per our requirements, and that resulted in like, you know, 35% of cost improvement, and also 20% of performance improvement.
As we are dealing with huge sets of data from several so resources, it's our utmost importance to you know, to be GDPR compliant. So, whatever the data that we are using for insights generation purpose, we are not using any PI data there. So, we hash all the PI columns using hashing algorithms like SHA or MD4 we store this data in ours3 locations. So, for all the insights condition purpose, we will be using this process data like the hash data. And since the clients and the other users have access only to these insights dashboard, we are not sharing any of the PI data with them as well. And for activation purpose, as you saw, like we are using cookies and then you know, device IP addresses. So since those are like raw data we are using our activation purpose in like only in a restricted environment.
So the data which is used for that is also in the restricted environment. And the pipelines, which are getting executed are also in a restricted workspace, where the users will not be having any access to read the PI data. But we can also be, but we can just see, you know, the count of data. So, yeah, with this, we conclude our session. Thanks for the opportunity. And now we can want to the Q&A.
Ardeshir: Oh, fantastic presentation, both of you. Very, very insightful. Let's take some questions that came in through the chat. First question is for organizations that are looking at your architecture and also want to automate their data pipelines? What are the first three steps that they should take?
Bitanshu: I will take this one. So basically, first is identify the right data source. So as I mentioned, is like we do a POC on the data source before onboarding them. It's. So as we have seen that the effectiveness of datas will also vary from Geo-Geo. And hence, it's most important to identify the correct data source, then we have to identify like, what is the processing system that we want to? So like? Is it going to be a spark engine? Is it going to the hive engine? What are the benefits associated? And how we'd like to save the output? What are the output columns that we want? What is the output file systems? Would you go for a text file, saving to parchive file? How would you like to partition this data? And what is the timeframe that we are looking for? Like, do we go for a more cost optimized processing? Do we go for a more internal like time based optimized processing of data that so I think the first three that we decide.
Bitanshu: I mentioned time optimized data. So what is, you know, when it comes to media analytics, what level of data latency is typically found to be acceptable? And I guess that may be use case dependent?
Ardeshir: Yeah. So there are two types of data latency that like we receive the data as batch ingestion also. We receive the data as real time ingestion also. Also, batch ingestion is like one day delay. Some of the datasets can be actually three to five days delays, because as I mentioned, there is an adtech industry, there is a lot of challenges regarding with that how to attribute the correct data provider. And like sometimes, the data provider actually verifies which is the correct Are you attributing them perfectly so that's why the delay can be up to three to five days. So in case of batch processing, it's like a 24 hour delay before users is seen and targeted in time. And see, like critical cases where real time is more important, for example, live soccer match, you want to target audience. In those cases, it's like the target audience can be target within a few seconds or a few minutes.
Ardeshir: The next question here is we only have about two minutes left here. So maybe time for one or two more questions. We saw that you're pulling data from ACR TradeDesk and AppNexus, are there other external sources or other types of data sources that you're pulling in or ingesting from, and combining that together in a single environment?
Bitanshu: Yeah, like we saw that in that slide. Like, we have like 70 to 75 different data sources. So for example, like, if as customs based solution approaches that, like, apart from like, for example, if you have some summer vacations going on for kids, you're seeing some kind of OTT shows on TV, and you also want to maybe target some location where the weather is sunny and you want to promote your maybe waterpark theme adventure or something like that. In such cases, you have you can you have like the weather data, you have that ACR data, you can combine them to bring out the insights.
Similarly, you can if you want to relate it with something else, for example, if you want to see what is the like, what's the footfall of a particular store after you have broadcasted an ad or something then we can we have to get the motion data we get to you need to have that location hyperlocal data and then we have to associate with the our ACR data or whatever data that you want to connect with and then you can make sense out of it. So it depends on the use cases we have to onboard multiple data sources.
Ardeshir: Fantastic. Great. Thank you for answering that. Bitanshu Das and Likhitha Jagadeesh of MIQ, thank you for both of you for being here today and zero gravity. We are going to we are going to move on to our next session right away. Glen Shuurman, senior platform engineer at Quintor will talk about how you can scale up Apache Airflow at enterprise level and how you can avoid mistakes in that journey. Glen has a great catchphrase. You say it's impossible. I say challenge accepted. And is always looking for ways to make and optimize make life easier using optimized technology Glenn thank you for being here today at zero gravity and the stage is yours.
Speakers:
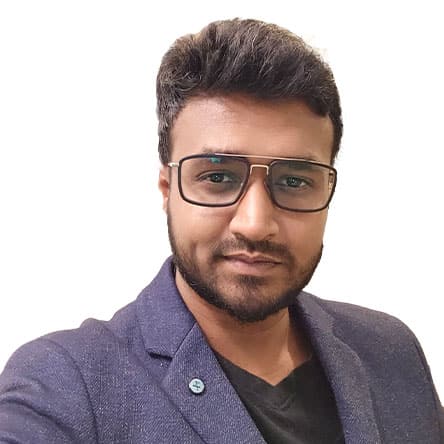
Bitanshu Das
Lead Data Engineer
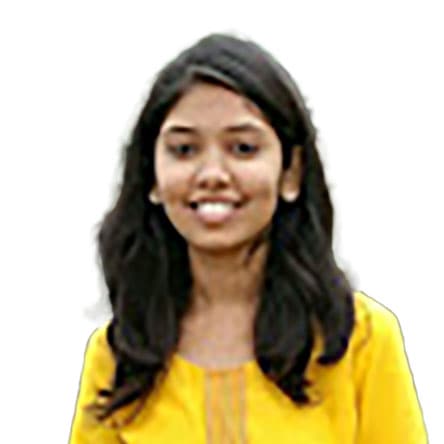
Likhitha Jagadeesh
Data Engineer II