Transcript:
Kaila: Next up, we are going straight into our lightning talks. So thank you, Ryan again. And we are now officially in three rounds of five minute lightning sessions. Up Now is Craig Colangelo, a Senior Solutions Architect at PM square. I'll spare the length of the introductions and ensure Craig has enough time to deliver this session. So Craig, welcome.
Craig: I'll tell you that PM Square doesn't know if data mesh can help fix your data mass. Data mesh is a new concept. It's not a new technology stack or some exacting architecture. It's more like design ideas around the new data management philosophy, where conventional analytic solutions are just nodes on the mesh. The main driving ideas to treat domain focused data products is first class concerns, and the tooling and pipelines is Second Class concerns. It shifts the current approach really from a centralized one to a distributed ecosystem of data products that play nicely together. In other words, a mesh. Now data mesh is still very young, but it'll gain real traction over the upcoming years. And PM square really intrigued by this new thinking and potential paradigm shift that could yield better data management practices.
Our hope is that maybe it can help your organization too. So why isn't conventional analytic data management working perfectly for many of us, some of us are simply living with the constraints of yesteryear, Kimball and Inmon revolutionized data warehousing around three decades ago. Technological advances since then, like cheap memory, commodity servers and cloud everywhere have all enabled a new age of analytic and data management possibilities. The conventional bedrocks are still just that bedrocks. But we have PM square think there's an opportunity to do better. A data warehouse is easy to query and understand, but it depends largely on preconceived questions. Data lakes are easy to load but challenging to use and often turn data into swamps. The lake house combines the two and gets us closer to a better technological solution. But fundamental problems still exist.
I would argue that the complex business problems and culture and factors themselves might need to be at the very center of our thinking. Consider this Legos first product was a wooden duck. IKEA started off by making pens and DuPont way back when started off by making gunpowder. Now considering what those organizations do now, is it enough to presuppose the business conditions or current world problems and narrowly build to that? Probably not. Maybe we need to change our perspective, even in the world of analytics from technology first to more of a product and process first approach. And with that, really think of our data as the valuable product that it is then treated as such. Remember that this is all still a work in progress. Zhamak Dehghani, founder of data mesh just finished her first book on the subject. Data mesh begins with a change in philosophy, culture and organizational structure, but it also prescribes key functionality that should be present. The idea of implementation is not associated with any particular vendor. In fact, data mesh remains technology agnostic. So data mesh is an intentionally designed distributed architecture under centralized governance and standardization for interoperability enabled by a shared and harmonized self serve data infrastructure.
It aims to move the traditionally distinct analytic plane closer to the operational plane, while still respecting their differences by establishing domain driven design as a key tenant. There's a lots of data mesh, but the four main principles are domain oriented, decentralized data ownership, data is a product, self serve data infrastructure as a platform and federated computational governance. With these principles, come new language. Instead of ingesting you serve instead of extracting and loading you discover and use. Instead of flowing data around via centralized pipelines, you publish events to streams, and instead of a centralized monolithic data platform, you have an ecosystem of data products.
Considering all this PM square believes that the Incorta unified data and analytics platform is a natural fit as a note on the mesh, if operational plane data as represented by third normal form source system data is indeed domain oriented then Incorta is uniquely positioned. Incorta loves third normal form data, and often doesn't require any sort of reshaping or refactoring of the source data due to its direct data mapping engine. So the naturally domain oriented data can remain just that with Incorta and it can be enhanced to suit the individual data consumers need. Please join us during the week of June 6 for a deeper dive into data mesh and to discuss how Incorta might be used to help take advantage of this new way of Thinking about data management.
Speaker:
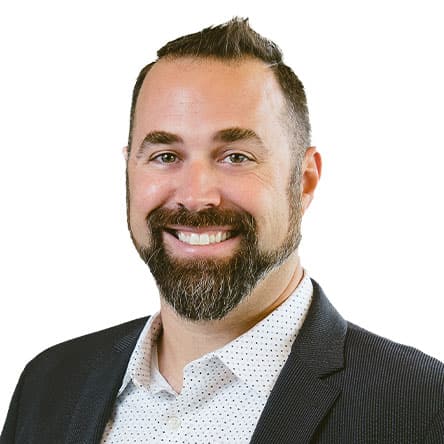
Craig Colangelo
Sr Solutions Architect, PMsquare