With 2022 right around the corner, it’s that time of year again: predictions season!
Will technology solve the global supply chain crisis? Will data engineers start using AI-based tools in their day-to-day work? Will “citizen data analysts” become the most influential people who work with data? Will retailers nail down product profitability at the SKU level?
Watch this on-demand webinar with Incorta co-founder and EVP of Product Matthew Halliday, Broadcom CIO Alan Davidson, and Ventana Research VP Matt Aslett for a fun, forward-looking conversation about big data, analytics, and cloud technology and business in 2022 and beyond, hosted by Eric Kavanagh, CEO of The Bloor Group. In addition to top predictions, we discuss strategies for navigating uncertainty in the market today – and tomorrow.
Transcript:
Eric: Ladies and gentlemen, hello, and welcome to our very special webinar today predictions 2022 can you believe it.
Eric: For data analytics trends, you should know your host here Eric cabinet of the blog group and DM radio fame.
Eric: i'm going to give you my predictions for about 10 minutes here that will have a nice lively Roundtable discussion with our experts and we'll hear from Matthew holiday of incorta, at the very end.
Eric: But we want to hear from you, too, so don't be shy ask us some questions at any time, we do have a technical expert on the call to answer any of your deep dark technical questions so let's dive right in like I said for analytics trends, you should know i'll talk about.
Eric: That for a minute.
Eric: we're gonna hear about the quarter product roadmap after that.
Eric: But really the expert Roundtable discussion is going to be a lot of fun, but talking to these gentlemen.
Eric: And they all have some pretty interesting views on the world and where things are going so let's dive right in.
Eric: Predictions where are things going folks, of course, you have to understand the past to know the trajectory of where you're going in the future right and so atl.
Eric: Obviously, has been a huge mainstay of the data space for many, many years really data pipelines and what we talked about today and data engineers right data engineer, is one of the new.
Eric: Really hot roles in the world of data management it's a really important and critical role, and so one of the predictions i'm gonna throw out here is that Ai technologies.
Eric: That are typically associated with data science are going to be used increasingly by data engineers, so why do I have a picture of cars here and cars being made by robots well let's think about this we've had robots making cars now for decades.
Eric: Now granted cars are very complicated machines are very complex but we've not had human beings doing the rivets does.
Eric: That like the old days in a long, long time, so my question is what's taking so long for Ai to impact data pipelines.
Eric: And I can tell you about it was about 27 years ago that yours truly had to do an assignment I had recently launched my company moebius media way back.
Eric: In that day in the last millennium and I had to do a mail out of several hundred names for a promotion.
Eric: And I recall that I wanted to personalize them dear john deere Fred etc, but the data that I had had the full name in the field.
Eric: And I was not going to sit around and type 500 or how many ever names, it was just to get all that correct, I thought to myself there's got to be a better way, and so what I did is I.
Eric: I did like scrape the data from the database I pasted it into a simple text kind of application.
Eric: Then I use the command F key to find and replace and I found all the spaces and I replaced them with commerce.
Eric: Because I learned, you can import a document into excel and if it's saw those commas in that structure even way back in the 1990s.
Eric: It would say Oh, maybe this is a data file you're trying to load do you want to use fixed width or their delivers etc, I said yes let's use the limiters of a comma and.
Eric: Then I opened it up and it's like I said open it up, and there I go I had my first name separated from my last name.
Eric: There are some times when you had to be careful because you would have middle names involved and i'm score what does that do that creates another column.
Eric: Right, so you didn't just have first name column black same column sometimes you'd have first class and then the third column.
Eric: Because you had the middle names in the last name calm Well, this is not an uncommon problem for people in the data space and again we're talking 27 years ago.
Eric: So I had to manually go through, and just find those exceptions and go ahead and type them back in that's a long time ago that's technically ETA out, I was doing the tea part by.
Eric: Replacing the spaces, with the commas it's a fairly simple script, if you will, and you could write a script to do that, even way back when.
Eric: So the question becomes, why is it taking so long for this critical process of data engineering to start adopting artificial intelligence well, there are a number of different reasons, one is because you know frankly.
Eric: We didn't quite have the computational power back then that we have today, and so today we can do that much more easily.
Eric: And I think you're going to see this really take off because folks Ai is really good at tackling the mundane the tedious and guess what it turns out, those are the tasks that no one really wants to do anyway.
Eric: One of my big mantras in general for this industry and for business and life, quite frankly, is that morale is the most important metric in any company.
Eric: In any life, quite frankly, because when your morale is high, you get a lot of stuff done if your morale is low, you don't get a lot of stuff done, you can have all the time, money, resources expertise experts.
Eric: In the world and you're not going to do very well if morale is low well what causes morale to go down.
Eric: tedium no one likes to do tedious tasks, so I think that's one of the main drivers in this major prediction that Ai is really going to much more impact.
Eric: The whole data pipeline space and that's going to be good for everyone, because that's the boring stuff anyway right when you do all the work to get the data prepared.
Eric: You can learn about the data, it is a discovery process, so there is some benefit to.
Eric: That outside of the benefit of getting to the point where you can do the analysis, but the analysis is the fun stuff that's the interesting stuff.
Eric: that's the stuff that's going to change your business and help you navigate out of what are some pretty strange times that we're in right now, today.
Eric: So prediction number one Ai is going to play a much bigger role in data preparation and data pipelines it's going to help data engineers who I promise you are overworked if not underpaid right now and they're going to be very happy and you're going to be happy.
Eric: So let's talk about citizen analysts, of course, citizen kane is a famous film it's regarded as one of the best ever made technically loosely about Howard Hughes a great media magnate.
Eric: And there are lots of similarities between reporting reporting in the world of data management and reporting in the world of journalism right, because when you report.
Eric: And what you're supposed to do is report what happened right, so I think citizen analysts are finally going to play a very significant role.
Eric: At scale in organizations for a whole number of reasons, you can see, the one listed at the top, there is that the tech is much easier now.
Eric: It used to be that you had to understand some pretty unwieldy technology.
Eric: To be able to do these things data science teams, for example, data scientists, which I think we all know, are in high demand right now well that's a rare skill set to be a data scientist who also understands the business.
Eric: who understands the mathematical components of data, science and it can actually interact with people and run a team that's a big deal and it's pretty rare.
Eric: But the deal is now because the technology is pretty straightforward, because we have really matured in lots of different ways in the data management industry.
Eric: I think you're going to see many more of these so called citizen analysts doing really good work and finding the insights.
Eric: And remember they're also on the front lines, so the further you get from the front line.
Eric: The harder, it is to see there's always in attenuation from the top of an organization, down to the front lines now granted really good leaders are able to motivate people on the front lines, so you can get a sort of bottom up.
Eric: reggae songs and that's what I think we're seeing now with the citizen analysts and, of course, people in the front lines know the processes best I mean it.
Eric: should go without saying, but I think it does bear repeating that if you don't change a process and actual business process that's in motion.
Eric: With the insights you glean from these technologies, then you really haven't done much at all right it's not good enough.
Eric: That you have an idea in your head that you think is correct, you need to make some change so those people know the processes and let's be honest changing a process.
Eric: Is kind of a scary thing right the operations folks your chief operating officer we don't talk about that person too much one thing which I think is kind of odd.
Eric: We talked about chief financial officers chief analytics officer chief information officer chief strategy officer chief technology officers right, but instead see oh who's actually responsible for all those processes and those folks do not like change very much.
Eric: They do like it when it optimizes something we'll talk about supply chains in a second here.
Eric: But they do like that, but they want to be very, very careful about it, so this is a very important component.
Eric: Now math skills data scientists, of course, should understand heuristics they should understand the algorithms that are being used my favorite is K means, for whatever reason, I think it's a fascinating algorithm.
Eric: to separate to classify by disparity, which is pretty interesting stuff but that's optional, I mean it's going to help if you have that skill set you're going to help your organization.
Eric: Please kids out there anyone who's listening, if your parents are watching this and you're in the background study math it's really important math is great, because there are actual right answers in math and that's fun.
Eric: Now the other big prediction here is I predict that the age old business it divide is really going to finish it's crumbling over the next couple of years, so as we go into.
Eric: I think that's going to be a big trend line and that's very good news for everyone, because the business finding with it is never been productive it's not productive now, it will never be productive.
Eric: Now there are turf battles, of course, there are other things that come into play politics are real politics cannot be avoided.
Eric: In this world, so there is something to be said for that, but I would say that.
Eric: Because we have such good technology, these days, and because the cloud also really bakes in a lot of governance, all the metadata about who touched what when who did what.
Eric: it's wonderful that all that stuff is just baked into the solutions that we use today that's good for audit trails it's there for good for governance.
Eric: For control points as we talked about in the risk management world a control point is a point at which someone can do something to change something right.
Eric: So that's so one of my big productions and trust, I think, is really going to be the cornerstone of success and I think that.
Eric: One of the sort of unrealized or under appreciated aspects of the world we live in the data management world.
Eric: Is there's a lot of mistrust, which has just grown over the years, because people see the data and they just don't believe it and that that will unravel any programs so trust I think it's going to come roaring back and it's going to be very valuable for everyone who uses these technologies.
Eric: let's talk about supply chains wow what a remarkable scenario we have in the world today with ships off the ports in Los Angeles, and around the world.
Eric: they've gone far out to see I think they're like 60 or 70 of them outside of Los Angeles, right now, what does that do.
Eric: That is a huge factor it's incredibly disruptive for all kinds of industries right, not just for retail, but for supplies of all kinds of industries food products.
Eric: materials, I actually wrote an article, but a year ago, or so about the cycling industry because I love to ride my bicycle and the cycling industry got hit hard with the territory.
Eric: So the interesting thing about all of this fun stuff that we're seeing with code impacting supply chains and so forth.
Eric: We had some early warnings, we had early warnings two and a half, even three years ago with all these terrorists, because the tariff sent the cycling industry sideways when you just couldn't get parts.
Eric: Even now, to this day in the motor industry lots of parts are hard to get because they're all over the world and it's hard to track down.
Eric: So what's going to happen, I think necessity is always the mother of invention so broken supply chains will absolutely positively spur innovation, they already have.
Eric: So one of the things I think we're seeing is this whole justin time analytics stuff.
Eric: it's really important to know where your products are especially for manufacturing where you have lots of different little parts that need to come together.
Eric: that's so the world of supply chain, right in the world of procurement is that you have lots and lots hundreds of thousands, quite frankly, if not millions.
Eric: of little parts, some of them are screws or rivets or Whatever the case may be, and if you can't get those then your whole.
Eric: supply chain is disrupted and your business is disrupted, I spent years in the print production business long time ago and I learned from experience that within that industry, the folks who are.
Eric: doing the actual printing press they're actually running that what my printer told me he goes look if that printer is not running.
Eric: we're not making money so when that stuff when that machinery goes down or doesn't have the materials, it needs it's extremely expensive and very painful, so I think justin time analytics on supply chain is going to be a huge issue.
Eric: And last but not least, let's talk about retail I think retailers are going to figure out and have already figured out.
Eric: That they need to know where their profit comes from, so I think they're going to have to understand profitability at the skew level in order to remain competitive.
Eric: And these for anyone who doesn't have kids these are fidgets and one of my point here is that unless someone on the call here is in the business of manufacturing marketing or selling fidgets we're all in the wrong line of business.
Eric: Because budgets are everywhere kids absolutely love these things there go crazy over them and they're crazy expensive.
Eric: That one in the top right hand corner that little was like an acorn it looks like a corn fidget it's probably 789 bucks.
Eric: And they cost probably 50 cents to make so fidgets are a high profit line item companies that figure out how to get to the bottom of all this, and really understand and it's difficult thing.
Eric: we've talked about tcf forever total cost of ownership we've talked about Roi return on investment, these are very difficult.
Eric: metrics to ascertain for all kinds of reasons but we're going to hear and one of the predictions we're going to hear, which I agree with 100% is that observe ability.
Eric: is going to be a really big deal we have much better technology for observe ability of data and pipelines of data in information systems.
Eric: There are terms like dark data, people are talking about or data downtime lots of really interesting concepts that show where pipelines are broken.
Eric: And i'm talking data pipelines that's really good for the people who have to generate the reports and do the analysis so observe ability is going to be big and i'm telling you this whole thing about retailers.
Eric: Really needing to know where they're making their money that's going to be a key success factor and so folks I would now like to introduce our speakers so i'm going to stop sharing here for just a moment.
Eric: We got several experts on the call today i'm very pleased i'll first introduce matt athletes.
Eric: He is he leads the expertise and digital technology covering applications and technology that improved the readiness and resilience of business and it operations this focus areas.
Eric: of expertise and market coverage include analytics and data Ai machine learning blockchain cloud computing he's got a lot on his plate matt as look welcome to the show today.
Matt: Thanks yeah no good to be here and yeah after 14 years in the industry, two months into work and ventana This is my debut on the.
Matt: webinar for ventana so pleased to be it.
Eric: hey well good for mark Smith, I know mark very well Ben Tom is a fantastic outfit we're going to get your predictions on what's happening in the world today.
Eric: And next up Alan Davidson he is the chief information officer of the global technology organization at broadcom.
Eric: In this role he's responsible for the continuous evolution of the technology landscape, a business application landscape, landscape and hardware and software product hosting so yeah just a few things going on.
Eric: And Alan is old school man he's so old school that his version of social media.
Eric: is writing Christmas cards and mailing them to people, which is the original atl the extracted the ideas from his hand he transformed them onto a paper with a pen.
Eric: And then they actually loaded it into the omelet and sent it i'm very impressed by that Alan tell us a couple of quick things about yourself and what you're working on.
Alan: Thank you, I appreciate you having me on here but yes i'm very old.
Alan: physically and virtually So yes, I mean we we are in a very interesting time and certainly in our industry and broadcom is, if you don't know is.
Alan: One of the major manufacturers of semiconductors and chips, which is also a hot topic so as well as supply chain issues.
Alan: and physically getting logistics around the world, we are obviously in a supply a physical supply chain issue, making the making the products in the first place so.
Alan: LIFE has definitely been interesting over the past 18 to 24 months so having access to to real time data in a in a streaming fashion, is highly critical default, we are trying to do.
Alan: So in my professional world i'm very online and make private world I like to keep it private so it's.
Eric: good for you and I just want to comment real quick.
Eric: Because i've studied this industry fairly extensively over the years and i'm always very impressed at the patience and the vision and the.
Eric: let's call it the endurance that you need to have to succeed in that industry because product design life cycles are very long.
Eric: Like if you think about when chips are manufactured, I mean there is at least a decade long cycle from design to production to implementation to.
Eric: understanding what they're doing and talk about observe ability that's really impacting your industry in very positive ways that I think that, even though the difficult situation have gone with.
Alan: You probably have a lot of really keen experience in.
Eric: The criticality of having that analytics and knowing what you can decide upon what you can't right real quick out.
Alan: Yes, no, I mean it's a you know, in the average lead time to from from inception to even just tape out and.
Alan: The manufacturing process is at least 18 to 24 months, even just to design and.
Alan: and get them to the manufacturing process, regardless of even shopping the good So yes, I mean it is a is a and a long time, and a very capex intensive.
Alan: Industry that we're in so you need to make some fairly substantial bets on and technology that doesn't exist in the world, so.
Alan: it's a fairly risky risky business but we've been at it for a few decades, so we're getting pretty good at it, but again it's it's having that having that capability, because the the the.
Alan: The nanometre and the size of these checks the the space between the transistors is getting so smallest assented and low single digit nanometers which is is not even you know hundreds of a human here.
Alan: So the the complex, the amount of data that's generated to build these these chapters is exponentially growing.
Alan: Even from from from know from two years ago, the amount of data, the amount of compute power to generate these steps is doing for X.
Alan: So I checked it, it takes for exit the compute power in the detail, acquired and even just two years ago and required to do that so it's exponentially expanding and it's not slowing down it's scary enough, I was just in a meeting the other be no one no talking about some sub nanometre.
Alan: chips so would already on to the next there the next iteration and that's about to hit and 2025 26 or something so.
Eric: And you know real quick something just popped into my head here the manufacturing industry you folks have been doing.
Eric: Working with sensors for a long, long time, so what is now relatively new to the rest of the world Internet of Things iot sensors.
Eric: That kind of thing you guys have been doing that for decades right, you have sensors on all these machines that you can then track.
Eric: I know Trenitalia just rolled out the first fully equipped train with sensors a few years ago was actually there in Rome for that, and you can learn so much about the manufacturing process and the material science to right real quick.
Alan: yeah I mean we we we make a significant number of components that feed into a lot of these databases.
Alan: And the amount of data that's generated from from these is is in the terabytes and petabytes know so because there's the volume of the volume is is immense, we are getting hundreds of thousands, millions of sensors that are all streaming data.
Alan: Through automobiles planes through buildings buildings themselves January and environmental data and there is.
Alan: No becoming even more and more relevant, because we know need to monitor air quality, we need to monitor not just is the temperature hot and cold, you need to monitor your quality unit is more than just the fire suppression and the.
Alan: A.
Alan: Security alarms you really need to understand the environment tools in the building, which also generate that but it's more.
Alan: There was a good report from the World Economic Forum about in 2020 or 2021 where you know 50% of the population is online.
Alan: In 2020 million people are coming online every single day, and what do they do the January data and the Facebook and instagram the one you know snapchat whatever the go on with exceptional myself, so I do a nicely.
Alan: What is it you know really what that means is is the amount of data that's getting generated both from two main sources those human beings.
Alan: That are coming online and Janet a thing, and people are loving online it's only been exacerbated over the past 18 months two years because.
Alan: People have to exist online people function, no more online than they've ever done before, so the Meta data gets generated from the human beings but also is 10 times more data gets generated from iot devices, but if you look at it from a.
Alan: historical perspective, again, you are, you are on a significant exponential curve, so when we have a fairly interesting view because we make.
Alan: A lot of components that go into stories the basis, so we supply store these devices to Dale and and a neta touchy and you know all the major storage device manufacturer, so the the amount of physical stories that gets shipped to hyper scale as like Amazon or Facebook or.
Alan: 10 cent alibaba that those types of machines it's no you're you're calculating it nine petabytes and zettabytes in terms of the volume is immense so.
Alan: to your point on your your excel thing to go through your your list of names you're beyond the point human beings can can understand the Meta data.
Alan: So you know just it's it's not our it's not a nice to have any more Ai ml is requirement, because you just can't process them in detail it's not physically.
Alan: feasible to do that we've gone past that point and we probably past that point about four or five years ago.
Alan: And this is not getting any any better so it's more than a competitive, we have to your prediction robots have been building cars absolutely robots have to analyze data it's not it's not it's not.
Alan: it's not a nice to have.
Alan: yeah additive, because otherwise you wouldn't understand.
Alan: You right.
Eric: yeah that's a pity statement right there folks, by the way, robots have to analyze data they've been building cars we need them to analyze data at scale.
Eric: And that feeds into several of our predictions, so this is going to be fun and, last but not least, Matthew holiday is a veteran software engineer and data analytics.
Eric: Expert with over 20 years of.
Eric: Experience developing products and taking them to market after more than 15 years at Oracle and several years managing engineers at Microsoft he co founded incortaand, of course, he also is.
Eric: is quite the race truck driver, you can see the truck in the background, there, which is very impressive, I have to say.
Eric: And like a lot of people there are a couple companies where lots of folks worked at some point in time and Oracle is one of them.
Eric: Think about 30% of the software executives around the world, at one point in time, worked at Oracle so Matthew welcome to the show and tell us a bit about what you see happening.
Matthew: yeah thanks it's good to be here yeah definitely interesting times, I think.
Matthew: What the best way that I can describe what I think is happening is very much when I think about analytics It reminds me about what was happening, when I first got into computers so back in like the 80s.
Matthew: When I started, you know getting computers lives, you had an older brother and he would put computers together and being into computers meant you were into.
Matthew: Knowing about driver settings jumper settings.
Matthew: interrupts and connecting and trying to figure out how to actually get the computer to boot up right, you have to get all the components largely underscores you bought a MAC but I couldn't afford a MAC in those days so.
Matthew: What I think we've hit now is that people don't do that today right when we talk about being into computers.
Matthew: People use computers in their daily life it's become part of how they create music it's become part of how they create art.
Matthew: we're just seeing that huge transformation take place and really the exciting things that people are using computers for today, I think, are coming from those groups of individuals, and I think the same things happened with analytics.
Matthew: analytics used to be, you were doing analytics it meant you were into all of the components, all of the pieces.
Matthew: And really it wasn't so much about what what was the business outcomes, it was more about you were into the technology, you were into just understanding the tech behind it, which.
Matthew: As a technologist yeah that is exciting, it is interesting, but I think where it gets really interesting is enough Friday night, you know I don't want to look at.
Matthew: The technology necessarily behind a movie I want to watch the movie I want to see what people were able to do the technology and the technology goes to the side, and then the story comes to the forefront right and so.
Matthew: that's why I think it's going to get interesting with analytics as we move further into 2022 and beyond, is that we're going to see this big shift where the exciting stories we hear are going to be around what people have been able to achieve with analytics.
Eric: yeah that's great stuff and you know it's just typing a message here to our panelists.
Eric: I noticed the auto correct kick on inside zoom so it recollected the Roundtable word for me so you've got automation everywhere, these days, what's kind of dive into our discussion, I want to throw the first.
Eric: prediction over to matt as let this whole concept about Ai being infused into the data pipeline, the data engineering process, what do you think about all that man.
Matt: yeah no I think it's it's really going to be touched on some of this already I think the the comparison you made to sort of you know, computers manufacturing cars and island talking about the manufacturing process is is really.
Matt: critical if you think about those kind of environments, you know that the fully instrumented and monitored, and you know, to ensure that everything's been done to the correct quality, and you know the efficiency and and everything's you know the correct validity and.
Matt: Why do we Why have we not been doing that, with processes and and and and analytics processes, and I think you know it's interesting you start to see the change, where people started to talk about data pipelines.
Matt: And it becomes much more visible in industrial perspective on it, which I think is is is a good thing, and I think what we've seen you mentioned observe ability, a couple of times.
Matt: You know the emergence in the last year of data observe ability platforms and we certainly see this is going to continue to focus from next year, and actually through 2025.
Matt: You know it's going to be a priority, I think, for vendors as to deliver more automated approaches to data engineering.
Matt: To improve trust in an enterprise data and, as you said, particularly to sort of automate some of the some of the more mundane important but mundane things particularly quality, I mean data quality software it's clearly you know, been around for decades, but it's historically.
Matt: being used by you know to to manually check and correct data quality issues, much as you were describing.
Matt: Earlier, and with the volumes of data, in particular, obviously the volumes that is talking about clearly you can't do that anymore, so you need to to apply.
Matt: machine learning automation technologies to that and also you know data OPS practices as well to to automate and collaborate.
Matt: And you know automate the monitoring of data user analytics projects to ensure that the required quality and validity, which also you know freeze data engineers up to focus on more.
Matt: In vertical is high value things so yeah absolutely I think you know we've seen this building momentum and that definitely will continue into next year.
Eric: that's a really good point I think you're spot on about observe ability and I think it's very exciting.
Eric: Because you know hit There too we just had to guess we didn't know, and I think there were so many occasions where people just or whatever just move on to the next thing because they didn't feel like they had the capacity to solve the problem.
Eric: But Matthew holiday i'll throw this over to you once you once you see once you can see what's happening once you get that observer ability that's a game changer and you can't go back you can't go back to working in the dark right, what do you think Matthew.
Matthew: yeah as I think this all started about when historically Mr see data engineers and data, scientists have separate tool sets.
Matthew: right if you're a data engineer, you were using you know, like clover or informatica or set of tools that.
Matthew: were specifically for your job right and your role was to transform data put it into a usable shape know create star schemas and do all those kind of things do you normalize the data so that analytical crews could run against it.
Matthew: And then, if you're a data scientist, you were talking about notebooks you were talking about pie spark you're talking about scala.
Matthew: you're in the thick of those kind of technologies.
Matthew: And what we're seeing now is just this huge shift and change where data engineers are now in place back data engineers are figuring out how can I use data science technologies to do my job right and so.
Matthew: Far first part of that is how do I get rid of the mundane, and so what I think super exciting in this is that when you get rid of you know, the 80% of the work that people are doing in D normalizing data.
Matthew: And you provide a capability and a platform like incorta others way you can bring data in.
Matthew: As is and then be able to run those technologies against it and be able to you know get into these new areas I think that's that's where we start to see.
Matthew: That transformation take place, because obviously we don't have enough data scientists honestly we don't have enough data engineers.
Matthew: We need to one I think just get rid of the steps that are unnecessary some of those steps, I would have came kind of stupid and honestly, even if you automate stupid things you know what it's still stupid.
Matthew: and the first thing I think is always remove those things remove the complexities simplify the process get it to a point that then when you focus on bringing Ai and you focus on bringing.
Matthew: How can I bring more insight and visibility and all the things you know that the challenges that Alan spoke about is just you know the data and how do you surface meaningful insights.
Matthew: When you have that kind of freeing up your time to not spend it all doing the very thing you shouldn't be doing.
Matthew: You know just moving data and transforming it for you can do anything with it.
Matthew: And you know a lot of reports have shown about 80% of even data scientists are spending their time doing data engineering task, just to get the data before they can.
Matthew: You know, feed it into something they're not spending all that time you know figuring out, you know the best algorithms to fit.
Matthew: To a problem they're trying to solve right and fix some of these like NP hard.
Matthew: slash NP complete problems which started to we never had anything to be able to fix them, and now we have these these algorithms that kind of you know, can get us like very close and approximate very close.
Matthew: close enough that we can actually do them in that kind of time frame.
Matthew: So with those things, taking place and those transformations and changes in the technology and having a modern tech stack and that's probably one of the most crucial things is.
Matthew: If you're sitting around again with kind of the antiquated approach that we were doing in the in the 90s, which largely a lot of these organizations, even the ones we mentioned today.
Matthew: It wasn't until recently a lot of them started changing, even though that they were instrumental in probably processing some of the largest data sets on Planet Earth.
Matthew: When it came to some areas of their business, they were still leveraging the technologies from the 90 because there was nothing.
Matthew: In that right if you're doing business application analytics what was at your disposal, like you, didn't have those tools and technologies.
Matthew: That could actually work on those particular data sets because they're very different problems, then the iot data sets that are generated so thinking of data as just being just one.
Matthew: I think is is just kind of thinking humanities, like all people are exactly the same know we're we're very different we have different experiences.
Matthew: And data is this is as nuanced as that as well, and so I think you know we've kind of done a disservice to how we even classify data and how we think about data, we need to think about it and it's the attributes and characteristics of the data itself.
Eric: And that's a really good point and I think it actually plays right into our second prediction here, which is citizen analyst so maybe i'll start with you Matthew holiday since you're kind of on a roll there.
Eric: It to me the maturation of technologies in the space is a big part of why we can get there now right it's you know it's like you, you don't i'm.
Eric: Not many people work on their own cars anymore and it's partially because they're so specialized right, you have to if you're a mechanic.
Eric: For for Mazda, for example, you have lots of special tools that you need to work on that stuff so people just don't do that anymore someone else does that.
Eric: But it's become very simple in in a certain contexts, but in the data world, it seems to me, these technologies now are such that you don't have to know.
Eric: Data science in order to come up with good insights, and so I think that the maturation of the technologies is shaping a future where citizen analysts can be very productive, but what do you think Matthew.
Matthew: yeah I think that's where it gets exciting, because at the end of the day, I think it comes down to curiosity and imagination.
Matthew: And the people who are gonna have the most curiosity imagination, are going to be the people who actually do move the levers and push the button, so if you're in a business at the end of the day.
Matthew: As matt mentioned earlier it's you've got to know exactly like what can you change right if you don't change a behavior or something, then it what, what are we doing so.
Matthew: In reality, like the creativity side of it is going to be with the business users, those who are closer to the decisions being made, they will see the opportunities they will see the art of the possible.
Matthew: Now, in historically that's always been, then you pass it on you kind of have to put that onto paper and pass it on to you know.
Matthew: Some technical expert who's going to then implement it for you.
Matthew: And you know they're asked you do you really need to know this question like How important is this question to.
Matthew: Like Is this a 3040 $50,000 question because most questions end up being though that kind of cost when you think about how long it takes to answer them, so it doesn't really feed into the.
Matthew: possible, and so we think about applications that being built on like the iPhone that ecosystem has now gone so far that.
Matthew: It really opened it up so almost anybody can build an eye, you know their business idea onto an iPhone APP and get it out it's not like the bar of entry was so high previously.
Matthew: it's kind of like I think we're entering this point where.
Matthew: Now for the citizen analysts like talking about the analytics tools, on your resume and listing them out is kind of been as dumb as listing out i've got word PowerPoint and excel.
Matthew: Like on my resume people just look at you like, what are you talking about it's just this is part of what you do right, this is part of.
Matthew: Being in the analytics, and so I would encourage everyone it's like you need to get more involved, like to think that this is the subject matter of.
Matthew: A few technical people in my company, and I will just ask some primitive questions you will fail as an organization, you need to be.
Matthew: involved in it right, it cannot be a separate like Oh, we just got a few set of questions and if you're not part of the process, you will not help shape the outcome.
Matthew: Like it's too late to just say I want this and not be involved in how it's made and produced so I think that's.
Matthew: One of the things I think it's really exciting and that's what we'll get some the most exciting stories and honestly quite frankly that's where i've heard some of the most interesting stories.
Matthew: In my day to day job with the customers I meet with has been from people who've never built analytics before this has been their first analytics project they might be technical.
Matthew: But they've never done analytics before and what they've been able to do it, or even business users, has been truly remarkable and it's really you know blown me away because they have the creativity and they know their business and they know what they do, on a day to day.
Eric: You know what's really interesting here is, and I was a philosophy major 1000 years ago in school English philosophy.
Eric: But i'm bias and we'll get to bias here in a second with with Alan.
Eric: But people who are in the industry and have been building these technologies and using the technologies for the last 1015 years.
Eric: They have their own set of biases and it's hard to kind of strip those away and realize that the world has changed i'll throw that over to matt haslett.
Eric: to comment on, because I think that's part of the challenge now is just changing your mindset about what's possible what needs to be done and what does not need to be done, but what do you think.
Matt: yeah I think it's it's I think the question was posed in the in the context of transformational analytics use cases and the role of.
Matt: citizen analysts in those I think that you touched on that there's you strip away from that some of that bias.
Matt: You know that.
Matt: And I think you know what we see is absolutely is the business users who know the business they've got the domain knowledge, a lot more decision making roles and more power to influence change they they can drive more transformational change.
Matt: Obviously, working in collaboration with with data analysis and data scientists, but they're you know they're the ones that can actually implement change so.
Matt: I think you know what we see is organizations that have been successful in that those that have built a you know, a data culture across the organization they've invested in.
Matt: Data literacy and I think it's almost a fallacy some some organizations fall into the trap of thinking that means you need to train everyone to be a data scientist, or to be a data analyst and and you don't wait on some people.
Matt: There is a benefit not for others, we need to do is just on them with information.
Matt: The outputs of these analytic processes.
Matt: You know there's still a place for reports and dashboards but you know we've seen the rise of decision intelligence that enables the delivery of information and insight and recommendations.
Matt: You know, into the business users chosen applications and tools and the part of their daily workflow I mean that could be an enterprise application, it could be something like slack it could be email or mobile.
Matt: But you know they don't have to go looking for it, and certainly don't have to ask for it and wait for a month for a report to be delivered, it should be part of the whole you know the the lifeblood of the organization.
Eric: yeah that's a good point and i'll bring Alan back into the conversation here we're going to.
Eric: Talk about.
Eric: supply chains and justin time but I know you did have a comment on bias and.
Alan: yeah I mean I think it's, you know as as it's been pointed out, and the session i'm very old and so i've been around for.
Alan: A number of years for for going through your pain and suffering of getting access to data, but I think.
Alan: In general, you know you see it in multiple areas, knowing just the data analytics piece, but there's a the law code no code and genres obviously.
Alan: proliferating through so people's ability to get access to data is, and I would see an all time high, so the ability for.
Alan: Easier access to data, so the challenge you then come into is efficacy of that data how real is that data will bite to the facts and data, because if you look at most.
Alan: medium or large companies, they have significant amounts of technical debt they've got a significant amounts of pockets of data we're nobodies there's a lot of dark spaces and companies will never really been able to analyze a lot of information and you then get into.
Alan: A I.
Alan: You know how is this data steel is this data activism data a correlated to two different things, so you have to be that I would I would then caution.
Alan: A the proliferation and free access or of data analytics because the technology is so powerful to do to give people and easy access, you have to then also because of this, maybe some beta that.
Alan: From a company perspective and again, as you can tell from the accent, I have some European roots in May, so the European regulation and authorities out there for gdpr international data transfer ebi daughter The list goes on for a it's a you need to be cautious about this significant.
Alan: volumes of data they're.
Alan: The easy access to data there, but who, who should really have access to what type of data is also important and quote from an observer ability from a buyer's perspective.
Alan: or influences, are you taken from from data can can have a positive and negative outcomes for for what you need to do so, you just need to be careful on.
Alan: As you are promoting and delivering these tool sets and the capabilities, because trust me the technology is there today, both from.
Alan: An infrastructure, as well as an application perspective, so you know the incorta stuff is an excellent tool for for giving you visibility and the capability to access, but you really need to then.
Alan: Have people understanding the quality of the data understanding the relevance of the data to make sure that people who are observing and accessing it understand the meaning and the quality of what that with ice is so.
Alan: You know, and obviously there's a significant amount of regulation know coming where you need to be very careful you into data privacy you into the personal.
Alan: Data regulations that you need to make sure that as you're deploying this, you have a framework and a process that allows people to have access to stuff that they should have access to based on the job type and the.
Alan: Profiling so you do get into more interesting areas and job profiles access to information and quality of information so it's it's a.
Alan: There is a land rush, and those are an exuberance and to matt's point on on on a capability to get access to data, we just need to be make sure that, from an efficacy, as well as a corporate governance perspective you're careful as to how that gets gets deployed in your in your organization's.
Eric: yeah that those are excellent points folks and we do have a good question from the audience all about analytic frameworks we'll get to those in just a second.
Eric: But maybe matt i'll throw it over to you matt as look to comment on observe ability Alan was just referencing what you were talking about.
Eric: Being able to see, and of course in manufacturing, you need observe ability did this rivet going properly or not a is being used for that very capacity now and a lot of environments.
Eric: But observe ability is huge and it affects our fourth big prediction, which we can kind of blend into now about.
Eric: How retailers really need to know at the skew level what is profitable and what is not it's a pretty difficult thing to pretty difficult nut to crack but real quick, what do you think man.
Matt: yeah absolutely I think you know the the level of.
Matt: Data that that's required, as you said, for organizations to to not just look at you know across.
Matt: The entire company or even within a particular region about profitability, you know we've gone way beyond that, in terms of understanding efficiency.
Matt: And particularly you know we talked about supply chain as well, knowing that you know your products or or even components, not just all products are in the right place for you to actually deliver to your customers and and support those.
Matt: Those customers yeah it needs to be done to finite level.
Eric: Matthew holiday i'll throw this over to for you to comment on as well, because the skew level awareness there's a whole lot that's required around that to get to that point, but you have to start moving in that direction if you're going to remain viable in this marketplace right.
Matthew: I think absolutely and I think it's not just.
Matthew: about the skew level.
Matthew: Right, you might notice you level at an annual basis across your entire organization I think it's.
Matthew: Profitability all the way down to by location and by individuals day.
Matthew: So, be able to know exactly in which locations which products are moving because there's a lot of decisions to be made now if you if you've got thousands of skews.
Matthew: Being able to do that as there's no easy task of being able to you know slice and dice that to really understand where Should I be putting products, how should I be thinking about negotiating deals with my vendors, how do I know.
Matthew: exactly where the profits are coming from if i'm not able to meet something if there's.
Matthew: Shipping challenges, what are the best, most profitable products for me to ship to a location if there's a truck that's going, and then you know we know there's a shortage of trucks like a truck drivers in the UK.
Matthew: Well there's a truck going from one store from one distribution Center to the stores what products should be on that truck.
Matthew: Right it's used to be just a logistics problem of like how to maximize the shipping of how much product can I get into that truck you can do it that way, but.
Matthew: Really what's the profitability and to build a look at that holistically to make decisions about your supply chain but understand exactly the profitability of the product and.
Matthew: that the very end is really key and that's why we see you know customers doing some really amazing things i've seen.
Matthew: Honestly, large corporations fortune 100 corporations, where they have no visibility into the skew level profitability, they might know general categories.
Matthew: they'll know regions but they won't understand how a store in San Francisco can is performing vs San Jose.
Matthew: And, should they where should they be moving those products individual stores will end up shifting and calling up saying hey I think i've got do you have some of this product like.
Matthew: you'll find all of those things happening on a local basis, but if you can get the data right, you can you know, improve the customer experience.
Matthew: And then, some of those industries, especially if you're in the consumer.
Matthew: kind of packaged products which have a shelf life if you know if you go to buy a cup of coffee or you want to buy something that goes along with it and they don't have it, you don't come back the next day and say well you know I didn't get my coffee yesterday i'll buy two coffees today.
Matthew: To make up for yesterday, right.
Matthew: Maybe if it's a computer you might wake, a few days, but most likely, what do you do.
Matthew: You know, a product go and find someone else who has it.
Matthew: And you move on and go that direction, so those kind of things being able to understand that, to make the right business decisions is going to be key because if you're not doing it guaranteed your your you know your competitors will.
Matthew: and your competitors will outperform you because they are using data to really understand their business and it all comes down to that visibility down to every single level in the organization.
Eric: yeah those are excellent points i'm going to use that that example if I didn't get my copy yesterday I get two copies tomorrow, even though I have about three copies a day.
Eric: But let's I know you wanted to share some.
Eric: Some slides here and talk about your product roadmap so i'm gonna go ahead and share my screen, we have some good questions coming in from the audience folks will get to those in just a second.
Eric: So let me go ahead and share my screen and bring this up here use slideshow and just kind of dive right in so incorta product highlights go ahead, take it away Matthew holiday.
Matthew: yeah so I just wanted to highlight just a couple of things that we kind of focus on here and and quota that I think do tie into the conversation that we have today.
Matthew: Of course there's many things that we're doing, but one of the overarching principles that we believe in one of the objectives that we say, these are kind of guiding principles is.
Matthew: We really want to simplify and reduce the time to your first insight and I think this speaks to a lot of what we spoke them today right.
Matthew: If we can make it easy if we can make it low no code and we can make projects that normally take maybe 16 weeks for you to get new data into your system before you can start to analyze it and reduce that down to.
Matthew: A few minutes or hours at most and not be measured in weeks and months or years and the worst cases that's that's a huge thing The second thing.
Matthew: That we use is really talking about empowering the citizen analysts, we believe in this, we believe that the bar of entry, has been too high.
Matthew: And, and the more that we can do to reduce this and.
Matthew: And kind of make it accessible to the masses, the better, and then the third one is really harnessing the power of many this is around communities, not just having locked in systems where.
Matthew: The power of your organization and the manpower or the person power that you can throw the problem is how you will survive or how you will move forward.
Matthew: This is about how do we leverage partners, how do we leverage the Community, how do you leverage.
Matthew: People like yourself and other organizations to better understand exactly how to engage with your data so.
Matthew: i'm just a couple of cases or examples and how we do that so simply find time to first inside.
Matthew: And incorta, we have something called our data wizard, which is a really great way for customers to be able to get data into the platform with a no code oriented data prep experience it's very fast it's very easy.
Matthew: Being able to define schemas without being pulled into the Co be able to load the data and to build a vision, you know visual management into that whole process.
Matthew: is really powerful where we're moving now is in the explore data phase so whether you want to use tableau or you want using quarter visualization or you want to use excel.
Matthew: You can go ahead and engage with that data right away, and so we've got a lot of exciting things with helping in that order augmented data prep experience.
Matthew: To help people any few clicks experiences them for the South for themselves, and so we have customers who are.
Matthew: kind of going through that but that's a big area of investment we're going to be a lot of exciting things.
Matthew: Being able to deploy what we refer to as blueprint applications where you can actually have the analytical APP for.
Matthew: All the components, you would need So what are the tables I need to get from salesforce or what are the tables or need from Oracle EBS.
Matthew: And how do I get those in how can I then interact with those data and engage with it so.
Matthew: A lot of exciting work going on there and there's more to be done, and we have some fantastic ideas that we're going to be rolling out so excited about that.
Matthew: For move on the second one, and so this is empowering the you know the citizen analyst and I think.
Matthew: You know there's been a long conversation about trying to kill off excel and and it's completely in my mind the wrong conversation, because at the end of the day I view it is how do we empower people and it's it's it's very much like.
Matthew: With our email clients probably every one of us might well I would guess have a gmail account it's probably very likely.
Matthew: However, i'm sure there's a myriad of us using different devices to engage with our email whether it's.
Matthew: you're using the MAC mail whether you're using the client near the Web browser whether you're using an android device whether using Microsoft outlook like there's many different options and ways to get at that data at your email.
Matthew: And it's the same for data it's like, how do we empower people to use that tool of choice, so that they feel comfortable to be engaged with that data again it's just a tool, now there are some limitations with excel you can only you know.
Matthew: process of a million records which actually I would say, you need a pretty hefty computer for that not to be a super painful experience but.
Matthew: One of the five got billions of records and I want to be able to go against that so.
Matthew: we've created ways for users to build us a really gauging experience of feels like building pivot tables in excel but be able to do that again billions of records of data with all of the.
Matthew: nuances of your applications behind the scenes, you can get access to all of the data behind it.
Matthew: And then being able to even put them into templates so create a financial statement have it, the way you want to look and and make it very easy for people to see that.
Matthew: So we're embracing that and we're investing heavily in the excel add in space.
Matthew: Because we think it's going to make a bit of resurgence, a lot of people trying to move all the way to dashboards but we see a lot of users just want access to the data.
Matthew: and getting access to the data and be able to synthesize it that way, they want join it with other data pieces of data is super important to them.
Matthew: The third one is promising the power of money so we've been really busy we've released parts of these things like a marketplace and our component SDK we just recently released.
Matthew: This is where we want you know we can never even called was the only way or the only company that could create the visualizations for you to experience would be behind.
Matthew: We would never build catch up, and so what we're trying to do a without component SDK is to allow the Community to be able to build so you can think about all the rich D3 visualizations that are on the market.
Matthew: Being able to drop those into our framework, so you can use a Meta data driven experience to be able to build them out package them up and then other people be able to use them.
Matthew: same with excel templates being able to build those and share those again how to get people very quickly to where they need to be.
Matthew: same with the SDK is for the connectors again we have hundreds of connectors, but you can build your own, and you can you know potentially share it with others as well.
Matthew: The analytical application marketplace talked a little bit about these blueprints, but this is where.
Matthew: I want to use a very commonly used business application, whether it's a CRM and hcm a supply chain application or if naturals application.
Matthew: How can I get that up and running today right if all of these projects are going to be it's going to take me a year or i'm so heavily invested in my current ecosystem.
Matthew: That I can never get out of that that's because you're approaching it with the same mindset of i've sunk in thousands of hours or days of work or effort into these projects.
Matthew: And the thought of moving to something else seems too much well it's kind of the wrong way to think about it it's like, if I can give you increased insight today.
Matthew: Is that a value to you, if I can answer help you answer questions today is that a value in blueprints really make that a distinct.
Matthew: kind of possibility for customers to now do and then analytical suggestions, so we have a suggestion algorithm.
Matthew: that people can interact with, and it can help them get to understand what should they be looking at we've talked about.
Matthew: Alan talked about there's just so much data that you can't know what you need to look at and so guiding people in helping surface those things.
Matthew: is really, really important and so with our analytical suggestions, whether it's suggesting well, if you look at revenue.
Matthew: 95% of people like you, who look at revenue also look at it, grouped by stage of a set of sales cycle.
Matthew: You know, things like that, and then say Oh, and they also filter it by look by geographical location.
Matthew: Helping you drive to where you need to get to, and I cannot be just within your organization, but even across all of those customers so there's just a few things that we're working on to kind of help move in this direction.
Eric: Okay, good stuff folks we got a few more minutes here, we may go a couple minutes long so a lot of good questions are coming in now.
Eric: The first one, I think, is fantastic and maybe i'll throw it over to Alan.
Eric: Just to give them a nice curveball but an attendee is asking as we take this leap forward.
Eric: Who designed the analytical frameworks, so that you can get a framework that pulls analytics together on multiple levels to design business strategies that will take the company forward in a competitive environment Alan, what do you think about that.
Alan: So I think it's a you know from a company perspective if you're trying to determine, you should environment your data, you really need to get.
Alan: You know, is the point of collaborating between business and it it very good at giving you a technical solution and zero knowledge about what the data actually means so.
Alan: So, but, but on the flip side everybody says, but I just want to add up these numbers and and that's why I went to get the revenue number so really you know, forgetting it really you have to break that not body, a bone you have to get.
Alan: And so it's an interesting skill set to have and the you know the problem is a skill shortage in terms of.
Alan: People really been able to take your business problems in it with a technical solution so that that business analyst with a technical band is really the way to do that the way we have addressed that within broadcom we do have people that.
Alan: A we you have to simplify your process so comes back to if you get simplified process you then get to where is your source of truth.
Alan: As you go through all these all these multitude of technologies that allow you to transform data and move things from point A to Point B to point C so.
Alan: At some point is like the Chinese response kind of campaign for a year, your intent of the source doesn't get to the end point in what you've what you've really mean and so it's really around.
Alan: How are you can you get access to your source of truth and the and do you have people that understand for that, for that is unclear, that is within your company so.
Alan: Creating that that business analyst role with with a an understanding of the technology and the data source getting to the source of truth is super key to then and you're not having to.
Alan: translate that into something something else so from From that perspective, simplify your process understand, for your source of truth is and and how people that can.
Alan: understand the context of what you're trying to do, because the technology is moving out the way that eventually is moving in the.
Alan: butt and then least different problems So be careful what you know buyer beware, be careful what you wish for because you'll get access to all the data, do you really understand what you're doing with that probably night.
Alan: So you really need that that context the people with the context to understand that.
Eric: yeah i'm a big fan of cliches and one that popped into my head right now is a little bit of knowledge is a dangerous.
Eric: thing right.
Eric: Now matt as that I have a very easy question for you the attendee asks what is the modern tech stack for data.
Matt: Well, it depends, where you're starting from and what yeah yeah there's so many various components clearly things like a data warehouse even atl person system go away and what analytics tools will be says the modernization of.
Matt: Everything that exists within you know new versions new, modern modern architectures also as as Matthew and spoken about are leading to that, so the collapsing, of what were previously considered you know individual.
Matt: Product.
Matt: categories collapsing into single data platform so increasingly we see you know where they call that.
Matt: They like or what yeah however you describe it, the collapsing of integration and data management data processing and analytics and Ai ml into a single environment, so I think.
Matt: that's what I would probably think we're talking about a modern tech stack and then you know various pieces of components that that hang off that.
Eric: that's a good answer um I took some notes there like that the modernization of everything and you're also right the legacy doesn't go away, I mean all the old stuff.
Eric: That we've talked about that's going to continue to happen, probably until almost the end of time, at least, for you know decades, I would presume.
Eric: But there is this is a very long tail to legacy processes, but the the vanguard.
Eric: On this is my impression the vanguard is getting further and further away from the Pack and if you're not embracing these technologies, you are being distanced from the leaders in the space.
Eric: there's a couple of good questions around careers, maybe matt how to throw this one over to you.
Eric: Someone is asking if there were an ideal path to take to get yourself good future job security in the data and analytics world what would be a good starting point, do you just dive into Ai or is there a better foundation to learn prior to Ai, what do you think about that Matthew.
Matthew: yeah I think you're great question something i'm discussing with my son, right now, as he's thinking about Korea futures and I think the question we set the comments we had up front is just.
Matthew: The importance of math and it's scary how much math I have forgotten honestly from from my schooling and.
Matthew: But it does come back so i'd say that definitely understanding ultimately just the math principles is going to really help.
Matthew: But That being said, I also think that we're going to start to see commoditization of those as well, to a certain degree.
Matthew: Like there will be a certain group of individuals who are going to be maybe creating the algorithms and really kind of like in the weeds behind it and then there's going to be the application builders when I started coding.
Matthew: In software I literally was like writing every function, I would define every single function now when you see people writing code, they just using pre built functions and.
Matthew: procedures that they can leverage and then they kind of become constructors of those packages and functions to be able to kind of create what they want.
Matthew: And so now it's very, very easy if you want to get a package that will read you know.
Matthew: do some ocr like you don't have to write that right, you can just plug it in and say here's my file and give me out the text and then do what what you want, with it.
Matthew: So there's gonna be some some elements of that I think in Ai i'd say first of all, is just start getting in started like.
Matthew: You know brush up on Python start taking some classes on Python is probably a really great starting point.
Matthew: leverage, you know understand what data frames are pandas and very soon, this get familiar with how data processing happens inside of those up those platforms.
Matthew: You can spin up an encoder cloud instance and do all of these very things we've got sample data there you can spin up a notebook you can.
Matthew: Look through some examples, and you can learn exactly like how to start interacting with the data, so I think there's there's great opportunities there's classes, obviously, you can take for that online.
Matthew: But definitely going in and going through some of the data sets you know there's some.
Matthew: kind of data sets that we use like with the airline traffic control, for example, and flight information that are kind of classic data sets for.
Matthew: machine learning to find out predicting flight arrival times you can start to look at those kind of things and start to kind of get involved.
Matthew: And then just start you know just kind of taking small steps that's what i've seen people have moved from data engineers who've never done anything in the realm of data science.
Matthew: They start off with just implementing a business transformation using using some of this technology or maybe highlighting outliers and said how do I find outliers in my data set.
Matthew: So there's some basic things that if you kind of get experience with that things start to kind of fall into place and the more you do the more you'll you'll get experienced with it, and so I do think there's.
Matthew: A great opportunity but love to kind of just say hey data science is the only opportunity I think data engineering is is is mushrooming and going to be.
Matthew: super exciting area so don't just think I need to get out of data engineering data engineering, I think, is gonna say resurgence in terms of using these tools to make the data engineers job so much more effective.
Eric: yeah and a little bit low go ahead.
Alan: Sorry Eric I would suggest go take a statistics class trust me that that's that's gonna be that's gonna help because physics and math number change technology changes physics and math math changes.
Eric: yeah that's a good one to statistics lies, damn lies and statistics right.
Matthew: We use.
Matthew: That to get back yeah most is just applied statistics at the end of the day for.
Matthew: Most people is Ai.
Matthew: really sorry yeah definitely agree with that on.
Eric: that's that's a good point, and that was like my cynical journalist side chiming in there, but we do have a couple of really good questions, one is asking this is kind of a fascinating question asking.
Eric: Is it fair to assume there is a job bubble accelerated by the data analytics and E commerce and supply chain management, how did these sustain over the next coming year say five years.
Eric: I don't think so, and there is actually one other comments I saw that i'll throw in and maybe i'll throw this to matt asked to comment on, but.
Eric: Someone wrote does building a small product using machine learning make me a good candidate for recruitment.
Eric: Yes, the answer is yes, if you go and actually build something and show what you built and explain how you built it Oh, my goodness, like people who hire are going to love to hear that, but what do you think about this.
Eric: because things are changing for sure, but there are so many new jobs, and I think the exciting jobs are always in like the nooks and crannies of industries that have been overlooked for years, but now can be optimized because of these technologies but matt as look what do you think.
Matt: yeah I mean I suppose it's a it's a fair question to be courses about whether whether there's a job bubble.
Matt: And if I you know we've been drinking from the Kool aid too much I would guess I would agree, I would agree with you, not because the you know these are to me seemed like they are sustainable.
Matt: careers, I mean we just to be talking about you know the historic data technologies don't you know don't go away, they could they could you know.
Matt: Organizations continue to use those, so I think you know the the the.
Matt: Jobs are wrapping up right now will will continue, or at least the you know the the skills that are that are required for those world will continue.
Matt: And just back to what we're talking about in a previous question yeah absolutely agree in terms of the statistics but also.
Matt: Engineering per se, interestingly, my my son's going through this thing about what he should do next, do you think about engineering what open minds, but that was.
Matt: How that big a transferable skill actually into data engineering and you know, an engineering.
Matt: Problem Solving which can be applied in multiple areas, including, obviously data and analytics so yeah I think when we get to the heart of it, these these are skills and roles that will will continue for.
Matt: Many years.
Eric: yeah and maybe just one last question for Alan and then Matthew and i'll throw it over to you because you do have so much experience.
Eric: In this industry and you've seen the fads come and go, I actually meant to talk about the Ai winter, at one point in our show and I forgot to, but there was an Ai winter.
Eric: When it was the trough of disillusionment is gartner refers to it, where there was so much excitement about what I could do that we just weren't ready yet it wasn't ready for prime time.
Eric: Whether to to the cost of the technologies, but you know to Matthew holidays point learning Python.
Eric: I heard a joke on a radio show a while ago that we did a guy said every coffee shop he walks into everyone's on their laptops doing Python and he lives in San Jose so it's everywhere, but it's important to know the long tail but really kind of focus more in the future right.
Alan: yeah I mean, I think you know bikes the point of data quality and be careful what you wish for sure you know I think we're Nova tipping point you're right the we had a point, a number of years ago where.
Alan: There was a promise of what is probably more viable today, so the technology just haven't caught up with what we were creating.
Alan: A man's quantities of data we just still couldn't get access to it, but I think there is no change from from today, both from.
Alan: A physical infrastructure perspective from a compute storage power, as well as the applications running on top of it, so the ability to get access to to significant quantitative Lisa is.
Alan: More highly available know than it's ever been and I think it's just the.
Alan: But like I say I think it's it's a buyer beware kind of thing, so you know, be careful what you wish for, but you will get all the information, what do you want to, how do you wanted template up.
Alan: How you want to use that information that comes up that becomes a whole webinar and so, in terms of what that would look like, but but yes, no, I definitely agree from a feature perspective my my cautionary tale is and we obviously want to make sure, and this highly an.
Alan: Interesting world of regulation and data privacy you do want to make sure that you are cognizant of, and as you get access as you get capabilities you build in some security frameworks you build in some.
Alan: efficacy of what you're what you're trying to display in ensuring that but it's definitely a brave new world, but every years a brave new one.
Alan: it's a it never stops, which is the great thing about the industry so it's you know.
Eric: yeah good point and final question i'll throw it over to Matthew holiday.
Eric: You know and Alan was kind of hinting at this knowing what to do next, knowing which opportunity to explore or to examine.
Eric: that's a big part of the challenge these days right it's just you have so much data there's so many things you can focus on how do you know which project should be your next project, what are your thoughts on that.
Matthew: I think, ultimately, that is a business problem, it has to be a business problem and not a technical problem that I see so many technologists.
Matthew: With the technology in search of a solution for us in search of a use case for it's like.
Matthew: hey got this great technology and they just randomly looking, I think that was kind of the disillusionment bad trough that you spoke about.
Matthew: Is that people got excited about the technology, not about what they can actually do for the business, if you can shift that excitement to be like this is what we could do for the business and you use technology to get there.
Matthew: that's where I think it's gonna be exciting I comment about you know what's on your resume is not all the technologies that you use, but the actual impact of the business that you were able to perform.
Matthew: So growing and really getting into like what are the business pain points, what are the business problems.
Matthew: And really that's where it ties down to like the analytics needs to prove that it actually was worthwhile.
Matthew: I think we're kind of like accountants that have the worst accounting, or worse, like financial practices for our own you know accounts it's like.
Matthew: We kind of espouse all of the benefits of data and analytics and then, when it comes to actually showing So did we use the analytics to show them what we did actually true value.
Matthew: know how many people are engaging with analytics we don't know but we put up the report they asked for it it's like this really rudimentary kind of like yeah we delivered on the ask.
Matthew: But the question should be, as you know, I asked my product team whenever they wanted a new feature, they want to build them like.
Matthew: How do we know it's successful what would be the business outcome that I can measure to prove that this actually was worthwhile and we'll we'll look at those features against that, and I think it needs to be the same.
Matthew: Every analytics project should be handled like a product management product, so if you're not familiar with how to run you know product management.
Matthew: Maybe take a project management class.
Matthew: Because that would probably set you up to kind of have the right mindset, to ask the whys behind the question why do you need this, what are we doing what are you doing before don't just take the surface level of.
Matthew: here's the requirement and then say i'll give a solution for it because you're so anxious to use some technology, maybe, if you truly understand the business requirement, you would use a different set of technologies, but you know yeah go ahead on.
Alan: So no but that that's exactly what sort of problem today is exactly that, in terms of we have.
Alan: We have because of the technology availability, people are generating reports because they have a good idea, but they haven't fully fully formed idea so then so we're know building up new technical debt of all these other reports that.
Alan: People have created without really thinking through the problem and trying to solve, which is why I was mentioning about you really need a.
Alan: Business analyst kind of fundamental you need to know your business your questions you're trying what problem you're trying to solve.
Alan: that's that's the true power of of under of getting the the access to the data it's not just getting access to data, you need to know the problem you're trying to address.
Alan: We then you'll be successful because people like to use that information.
Eric: yeah that's a fantastic point and folks I do want to encourage you to download your free trial big thanks to all the folks from incorta for allowing us to bring you this show today.
Eric: So you can see some information right there on your screen we do archive all these webinars for later listening big thanks to all of you for such fantastic questions big thanks to our panelists and incorta.
Eric: 2022 it's a brave new world every year, so says on it's a fantastic quote will talk to you next time folks thanks so much we'll catch you up in 2022.
Matthew: Thank you.
Hosted by:
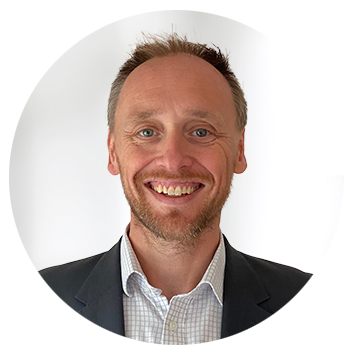
Matt Aslett
VP & Research Director at Ventana Research
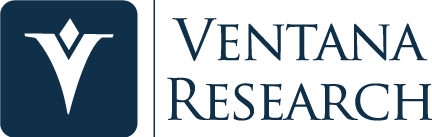
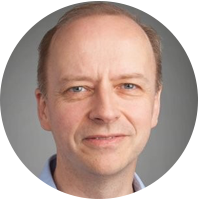
Alan Davidson
Chief Information Officer at Broadcom

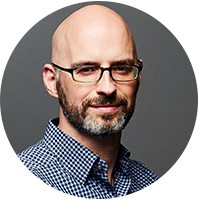
Matthew Halliday
Co-Founder & EVP of Product at Incorta
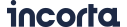
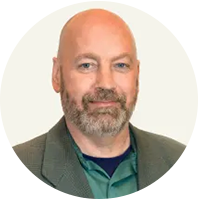
Eric Kavanagh
CEO at The Bloor Group
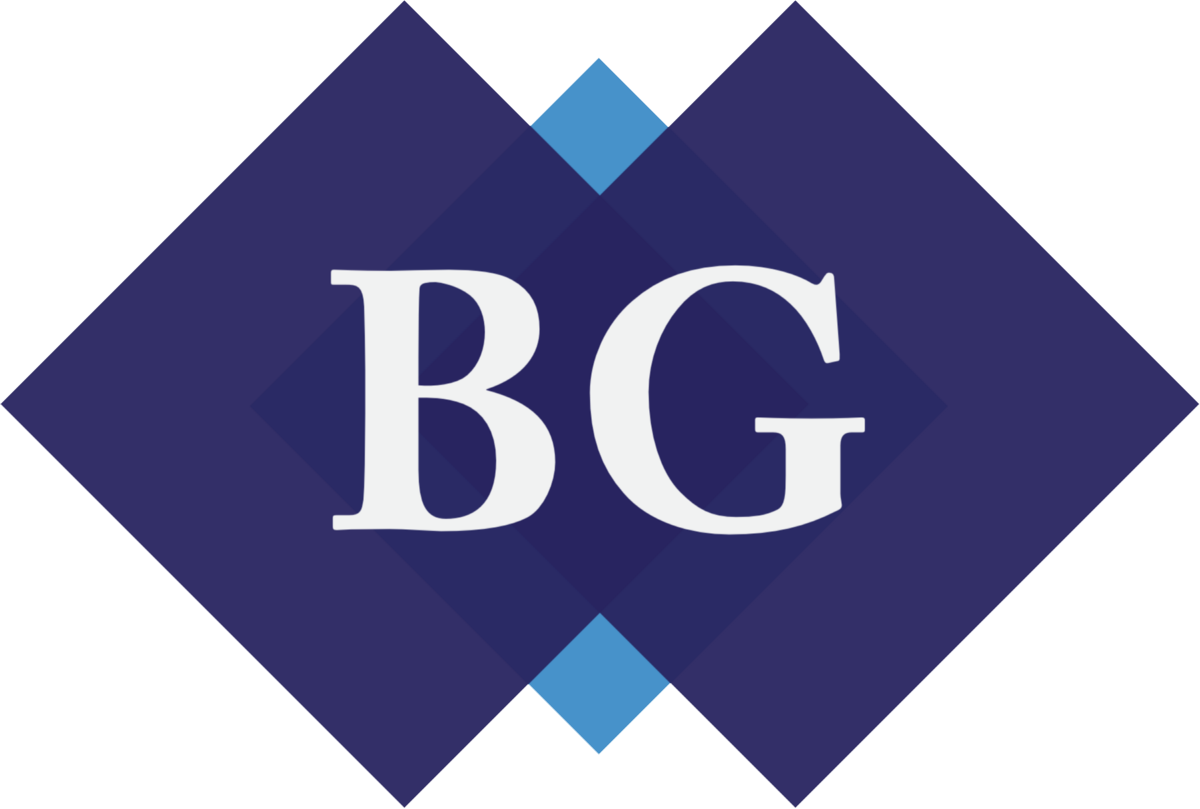