Unlock the full potential of business-critical Oracle data for operational insights in record time.
Creating and updating data models and reports using Oracle Business Intelligence Enterprise Edition (OBIEE) or Oracle Analytics Cloud is a lengthy and resource-intensive process. Requirements gathering, schema development, and data modeling can take weeks or even months. However, million-dollar operating decisions that rely upon dynamic enterprise data from Oracle EBS, Netsuite, and other sources can't be put on hold while data is made ready for analysis. It’s time for a new, modern approach to Oracle analytics.
Watch this webinar to learn how you can unlock the full potential of business-critical data in complex source systems to gain up-to-the-minute operational insights in record time.
You'll learn how:
- A low-code/no-code approach to data management can increase business agility and enable faster, more precise decision making.
- Incorta enables you to directly map to the data source, eliminating traditional transformation and aggregation steps to deliver all usable data to the business.
- Business users can easily build their own high-performance reports to answer complex business questions in seconds.
- Why a major fortune 500 company decided to migrate from OBIEE to Incorta and the instant benefits achieved.
Transcription
Mohamed Rabie: Let's give it two more minutes until everyone successfully joins.
Mohamed Rabie: I think we can start so first of all, allow me to thank you all for attending today's webinar and welcome you all in our webinar goodbye obiee and welcome the data liberation.
Mohamed Rabie: During today's webinar we're going to be focusing more on how Incorta can help you guys resolve the most complex business problems that you are currently facing within your organization and how we can utilize all the capabilities within the product to serve all of your needs.
Mohamed Rabie: So with you today in the webinar I am of course, and Amit Kothari our director of solutions engineering who has around 20 years plus experience in the IT domain in general and, specifically, in OBIEE and EBS and fusion reporting.
Mohamed Rabie: I mean comes with lots of experience in that area does can guide us and help us to understand more about what is the real problem that we are currently seeing and how we can easily resolve them using Incorta.
Mohamed Rabie: Also, with you today myself; Mohammed Rabie senior sales engineer for me quarter have around seven years of experience in the data analytics in general.
Mohamed Rabie: Coming from multiple products coming from multiple mentalities and whatsoever and i'm going to discuss more about how in court as product behaves and the main focus of our our architectures and also next slide please me.
Mohamed Rabie: Right, so what is incorta so incorta, basically, is a unified data analytics platform that has been built by Oracle application application technologist that has around Hundred Years loss of.
Mohamed Rabie: Oracle Apps experience we have Mr Osama El Kady our CTO, Mr Matthew halliday, Hichem salemi as well, the main aim or importance is to focus on solving the most challenging problems.
Mohamed Rabie: In the business domain, right now, which is how to take my data from point A to Point B in an easy and fast way without the need to go through all the hazards of the etfs building models and what's.
Mohamed Rabie: Our approach actually has been tagged and.
Mohamed Rabie: sponsored by multiple investors in well known ones for the white like Google ventures Microsoft throwing.
Mohamed Rabie: Let's give it two more minutes until everyone successfully joins.
Mohamed Rabie: web true ventures and.
Mohamed Rabie: So now, I believe I can drop the MIC to my colleague, so he can take us through a soft opener thanks.
Amit Kothari: Canyon area.
Amit Kothari: hi everyone, let me quickly dive into our topic for today so basically what we are.
Amit Kothari: What we wanted today's first understand what are some of the challenges associated with obe and to AC and how in quarter can help you.
Amit Kothari: solve that problem and quickly move to more real time reporting to some of the problems which we see associated with a bi is one of the biggest is you know it's very difficult to achieve timely insights so What it means is.
Amit Kothari: Having real time access to information is extremely challenging in this in the sense that, because we have all these etl processes.
Amit Kothari: Running where you have stars images and you have to populate the staging layer and the dimension and the facts, so when you are running all the detail that takes a lot of times over, and the project, which I have run which i've worked with customers, they only able to do to twice a.
Amit Kothari: Twice and load daily loads because they're incrementally tlc used to take like seven hours eight hours so it's very so it leads to higher latency.
Amit Kothari: That's so, so basically when customers I in the closing period and they want to have to.
Amit Kothari: Fast data refreshes are extremely hard to do, so, this is one of the biggest so many of our customers use us in quarter to close the books so that's one of the biggest.
Amit Kothari: advantages of using Incorta.
Amit Kothari: So that's one of the biggest problems.
Amit Kothari: Okay, the second one is data modeling in general, so it will be a confidence or admin tool and you know you have the Metadata layer, you have to.
Amit Kothari: You know, have all this do the physical modeling, the business modeling so and so.
Amit Kothari: So you need experienced consultants and expensive consultants to do all that modeling so it's not a very self service kind of model and more like.
Amit Kothari: kind of IT driven, Right.
Amit Kothari: There is basically.
Amit Kothari: You know you have to start just to basically what we had seen with other customers. If businesses want a couple of new fields added to their reports, it takes a long time for it to fall for the business before the basically there you know consultants to.
Amit Kothari: modify that PD then publish it to production so it's you lose agility that's one of the biggest problems we have seen.
Amit Kothari: The third one in the most other most important one is.
Amit Kothari: Extremely extremely sub optimal performance so basically what happened since everything all of the data is in a listen database.
Amit Kothari: Where is the data, growth happens.
Amit Kothari: The queries do not scale very well, so we are seeing with multiple customers were even the prompt refreshes take like one minute two minutes, and then the entire.
Amit Kothari: Entire report will take 30 minutes 40 minutes, just because they're all of the data growth has happened so much there the fact tables have like a 200 million rows field in billion rows and now you're joining with so many of other dimension tables, so those queries take a long time to refresh so that's one of the senior challenges we have seen with relational database in general that doesn't scale for high volume data and and what's happening is, we have seen across everywhere that the data growth is happening at a phenomenal rate.
Amit Kothari: Then let's talk about increasing the text that is also becoming very, very complex in the sense, you have so many different kinds of tools, one is, you have a at a layer, then a Meta data layer, then you have.
Amit Kothari: Storage complexities so All of this leads to you need your total to see your total cost of ownership increase in the sense now, you need especially people with specialized skills to do your ATM work to do your Meta data to do your admin work for your storage and all that so.
Amit Kothari: And it's very hard to optimize the performance so basically if you have an Obiee tool working on your relational database.
Amit Kothari: to optimize performance, you need specialized people with devious skills to create your indexes and here you have killed query by query so that leads to lot of bottlenecks in the reporting right and people when you add.
Amit Kothari: menu at the financial closes and all that you want you, you know you want your data to be so very quickly.
Amit Kothari: In a very quick question.
Amit Kothari: Let's go to the next slide.
Amit Kothari: So this slide talks about basically you know what's what the current landscape is. People have implemented databases like EBS applications and EBS and others, and they are part of the digital transformation project.
Amit Kothari: They want to move to more cloud based technologies so EBS many of the bs customers are moving some of the modules or their entire application to basically the cloud application so what's happening is.
Amit Kothari: So the first problem, that we have seen is, we have disparate technologies so now, you have existing pipelines from your implement databases to populate your.
Amit Kothari: populated data warehouses right now, all of this has to be rewired for your cloud era now and what's happening is, we have seen that the entire data structures are so different that it is like a huge project just in itself to do all this rewiring.
Amit Kothari: The other thing which we have seen is basically the customers only migrate only a portion of the data that implements data to the cloud so, for example.
Amit Kothari: They might just migrate open transactions, which is just like a subset of the huge historical trends and so they also have a need to also report on historical data, along with the.
Amit Kothari: historical data coming from an infamous application combines that with your, you know the new transactions, which are there in the cloud application So how do you do that, in the same in the same dashboard where you have.
Amit Kothari: Basically, depending on the date prompts you want so suppose if you know your cut over to cloud happened on first January So it's getting what you want this before for January you want data coming from your historical historical close on the historical context and coming from your demise combine that with the new one, so that itself modeling that itself a lot of problems.
Amit Kothari: And the other problem is how much data to migrate that itself is a bit so basically we have seen customers typically.
Amit Kothari: migrate only their master data and the transactions are all of the transactions are just.
Amit Kothari: Physical in the erp cloud application, they just want to do, new transactions so.
Amit Kothari: And then the other problem is.
Amit Kothari: A modeling challenge so basically if you look at.
Amit Kothari: The erp technologies, they like, for example, the cloud erp.
Amit Kothari: They don't give articles and don't give us access to their backend system, so we have to rely on flat files for that and I, which has a completely different structure, so this is some of the challenges with that.
Amit Kothari: Then, now the second thing which is the big problem is data visibility so Oracle doesn't give us bulk extracts both rest API expects to extract from Cloud, we we are given.
Amit Kothari: flat file extracts coming from UCLA so.
Amit Kothari: So what happens is to understand that and to model that requires a lot of deep skills in the cloud. Then basically the other thing is the data model itself has become so complex, now it is really hard to understand and model it in.
Amit Kothari: Your admin tool and all that so these are some of the big challenges associated with this.
Amit Kothari: Or that third one is self service so self service has always been a big problem it'll be a it's always managed reporting kind of scenario where.
Amit Kothari: You know developers will create the model for you the basically the details and musical prepare the report for you and you know users will just have to kind of go basically run those reports there's no so if a user wants to.
Amit Kothari: Basically edited and add new policies or remove some columns or have a different view of that, you have to go back to the.
Amit Kothari: To the developers and that takes a lot of time, but we have seen with multiple customers it typically takes one to two months, just to add one or two fields, because.
Amit Kothari: That is because there's only one team doing all this, so we want more agility where users are completely certain of the self-service model. We have metadata exposed easily when they can just drag and drop and create all these departments.clet's talk about.
Amit Kothari: There so.
Amit Kothari: So that's So if you look at the encoder platform, the input unit, the unified data platform, it was built ground up to solve all these problems, so one of the biggest thing which we have done is.
Amit Kothari: Direct data mapping So if you look at this slide So if you look, aside from the left hand side, so those are your sources.
Amit Kothari: And then, what we do is we bring data, as if we don't reshape the data in terms of so your source has a lot of.
Amit Kothari: A lot of the source tables are right, so what is typically with Obiee year to transform the data into a star schema with facts and dimensions, yet we don't do all this transformation, we just bring data as this.
Amit Kothari: We just joined them up, you know va and this direct data mapping is what leads to higher performance.
Amit Kothari: And agility in the sense that, because now you're not reshaping the data, you can have highly very, very low latency reports, you know our customers, many of our customers are refreshing data every 15 minutes some some even lower than that, so all of this, without.
Amit Kothari: Because what we're doing is we're doing incremental data extracts table by table.
Amit Kothari: And that's also parallel data loading so that eliminates all this huge transformation and we don't have any pre aggregated data, so all of the aggregation happens on the fly.
Amit Kothari: Because the Incorta performance is so huge that you don't need to pre aggregate data for performance so immediately the users can start.
Amit Kothari: You know, looking at Very, very latest, you know, as you know, they can have as many as they want and they can immediately start analyzing all the data.
Amit Kothari: So that's so then.
Amit Kothari: Maybe we'll talk more about you know, the more about architecture and all that how we do it, this is what at a higher level.
Amit Kothari: And now once once we.
Amit Kothari: ingest all the data into encoder right, then what we have is to have self service we have this business schema layer
Amit Kothari: So what happens is the back end if you look at it, the number of tables involved, they are huge and then the huge number of columns in there.
Amit Kothari: So this is like a very simplified presentation layer which we have right, and that has just a subset of the columns is the need to develop the self service reports.
Amit Kothari: So these are very business friendly views of the data so suppose you have data advanced people are or GL so we have this very, very small business schema so that they can immediately start recording of that and it's very easy to add more columns to that so it's totally self service.
Amit Kothari: So so basically.
Amit Kothari: So as part of talking more about the blue preset front, but we have seen all these business schemas by various areas, so you can immediately start creating reports of that.
Amit Kothari: One of the biggest advantages of this architecture is we don't need to create all these redundant data poppies. That's one of the biggest things we have seen. It'll be rare because to handle performance and all that you have to go on, you know the one aggregating.
Amit Kothari: You have different persistent tables just for this, for your aggregated data, so you need different different tables for different types of aggregation otherwise it doesn't scale so here that, when we extract data from table it only exists.
Amit Kothari: Only in one one place so there's so so basically you're not copying data so that reduces the storage cost your memory costs and also your.
Amit Kothari: Extraction cost right.
Amit Kothari: Then, basically, since we have all this.
Amit Kothari: Low latency extracts you know it leads to basically all the users working on the same data.
Amit Kothari: Then data governance is also.
Amit Kothari: very much supported here, so the data goal, and they can.
Amit Kothari: Basically, make sure that the.
Amit Kothari: You know, basically we have auditing of the data, and you know all the security and everything.
Amit Kothari: You know all of all of the constructs around the data governance piece is also supported here.
Amit Kothari: Let's go to the product comparison.
Amit Kothari: This lifestyle more more about how some for some of the various topics, you know various comparisons of in quarter versus or via usc.
Amit Kothari: The biggest insight, as I was talking about how do you build, how do you surface all this source data.
Amit Kothari: within a very short time that that is very, very extremely challenging to do with the va intimacy with our in quarter, I can just bring in the raw data growth raw data and tables, as is right, I just need to join them I don't need to reshape the data no ideas.
Amit Kothari: Then the biggest thing is performance, so I don't need to worry about creating indexes, because all of the data is in memory and all the joints have been rejoined and what we call the technology is called direct data mapping so that leads to.
Amit Kothari: Huge performance gains without reshaping the data.
Amit Kothari: Then we have these blueprints for many of the very popular earpieces like oracle's You know various technologies.
Amit Kothari: It's you know customers can quickly start reporting on the data.
Amit Kothari: Now the big thing is self service analytics so you know, going from.
Amit Kothari: Basically, from aggregated data to detailed data it is very, very simple because we don't have any pre pre aggregate data and then searches integrate so and then across the dashboard we search highly integrated.
Amit Kothari: In the sense it's very easy to locate data fields and also start reporting on.
Amit Kothari: I can go from one dashboard and then I can drill to another dashboard and it's very easy.
Amit Kothari: To do analysts across transactions so from and we have built around business processes so say procure to be so right from procurement I can drill to buy tables from people, so all of that is easily possible.
Amit Kothari: incorta is a data platform, so when we support hundreds of connectors.
Amit Kothari: So it's very simple to integrate new data, so I can have.
Amit Kothari: Your ABS Oracle EBS data implement swift cloud API can join them up, if you have other technologies like SAP salesforce netsuite flat files, you can easily bring them up join them up and in the same dashboard I can.
Amit Kothari: You know, have a consolidated view which gets all this data together.
Amit Kothari: So that's the steer that also is one of our biggest strengths is having reporting across multiple sources and.
Amit Kothari: Then our so we our tech our platform is we call it UDP unified data platform so it's really you can do analytics without, as I said right you don't need to pay the data rose.
Amit Kothari: Know details as is.
Amit Kothari: Because there's no etfs.
Amit Kothari: or this complex metadata layer like obe.
Amit Kothari: The maintenance is very easy.
Amit Kothari: So what we have seen is in many of the projects which are meaningful.
Amit Kothari: You know they had a team of like 5200 people just to develop all the ETLs and maintain them, but you know when importer comes in.
Amit Kothari: You know, with very few resources, you can do a lot of things so many of the resources could do much more than what they were able to do . The same resources, we are now able to deliver much more content than in the past.
Amit Kothari: Then, security, so we have security built into our system and it totally replicates the source security.
Amit Kothari: So whatever security, you have in your source, we can easily you know kind of achieve the same thing in incorta
Amit Kothari: Then we added machine learning technologies so that it's very easy to basically do all this prediction and forecasting directly within the context of your.
Amit Kothari: of your custom reporting it's very easy.
Amit Kothari: it's pretty tough about blueprints so this blueprints what we have done is it is basically kind of your start an application so once you once you are built around business processes like procure to pay order to cash.
Amit Kothari: GL and all that, and this will work with biggest customers and many of those dashboards what P, having a blueprint actually live with customers, so they have real real metrics which are very, very useful to customers.
Amit Kothari: And then, so we support all this drill to details.
Amit Kothari: You know, you know you can do snap shorting.
Amit Kothari: As slowly changing dimensions time series mlm so all of this is possible, as part of our platform and because we have this.
Amit Kothari: Is all this blueprint for a particular era, p.
Amit Kothari: It's very easy to start reporting right from day one, so we have seen 75 to 80% fit of our blueprints and then you just start adding your customizations.
Amit Kothari: And basically whatever dashboard you have you just start customizing that and adding the fields you want, so it gets it, this has been very successful with customers and i'll show you a DEMO of that.
Amit Kothari: So this is more.
Amit Kothari: Valuable to someone level the content, which we have so we have support from supply chain financials HR all all pieces of it and also and they're all integrated around business processes so.
Amit Kothari: It's very easy to add more stuff in it, so we have the same content across EBS vision, the rp we also have next week blueprint salesforce ASAP all of all of all of those applications available.
Amit Kothari: This slide captures.
Amit Kothari: at a high level in what I have been saying so far, so, if you look at your left hand side, you can have multiple sources.
Amit Kothari: source data ingested within encoder so APS yet peak loud, they can all stay within incorta, so you can have the detailed data of many festivals, we have seen just a hair and hybrid kind of approach only.
Amit Kothari: You know, with one model at a time to fuse to crowd the IP or they might just do that later in the year, the details sub ledger data isn't EBS so we can bring those into it, we can drill from ledger to the sub ledger.
Amit Kothari: So it's very really optimized for reporting.
Amit Kothari: The next two slides talk more about our particular use cases which we have done recently, so one of them is a fortune media campaign, a huge company, a huge presence everywhere in the US with with huge amount of data, so they are basically EBS Oracle EBS customer they had big problems they were facing were kind of the known problems, one of the problem was data refreshes.
Amit Kothari: They are only able to do two refreshes two times a day because of the huge amount of emails ahead, then there will be a performance that was extremely poor. Each of the reports was taking 30 to 50 minutes.
Amit Kothari: So we came in and plus the users but not happy because they were not able to get their family reports.
Amit Kothari: So we totally replaced their supply chain financial analytics within.
Amit Kothari: Just six weeks so basically within four weeks we deployed the blueprint within four weeks, all the customization was done and within the first six weeks, they started that testing.
Amit Kothari: Just within six weeks compared to a couple of years with the tech industry implementing a BA in data warehousing.
Amit Kothari: Then we were able to do 96 data refreshes a day that was one of the biggest when they are not able to close their.
Amit Kothari: There's no idea, this will be a totally self service kind of reporting.
Amit Kothari: So and and you know and getting a new report or a new field was just in a matter of hours because all of our business schema had all the fields, they wanted it to be very, very easy to start surfacing all this information to the users.
Amit Kothari: Another big story which we had was a big one of the world's biggest social media campaigns.
Amit Kothari: Where basically the other problem they had with the same or big and informatica ideas.
Amit Kothari: Extremely huge data set that huge data sets there will be a reporter very slow and they have it having a lot of problems closing the books on time, so the users wanted the business users wanted data refresh every 30 minutes, but with ETS, they could only do two or three times a day.
Amit Kothari: So just for the work or one of the initial ucs which we did just in one weekend we could just come in and complete their account receivable.
Amit Kothari: aging data that was one of the biggest things they use for closing that is just this one weekend it took or you know it just took for us to basically come in and finish their aging report and they could do this fast reporting.
Amit Kothari: Okay, so now let me show you one of our demos on an hour blueprint.
Amit Kothari: So this is incorta.
Amit Kothari: So let's directly edit our dashboards first.
Amit Kothari: See, if we look at our This is our EBS blueprint So this has a high level financial supply chain knowledge in manufacturing projects human resource so everything is supported here so let's go to financials we have all model supported year gap was receivable so let's.
Amit Kothari: Go to people's so and then I will show you how we have more in the data so let's talk about people's overview, so this one is kind of gives it a high level account payables.
Amit Kothari: Information So if you look at it, this is kind of the design pattern, we have is basically, you have the kpis on top.
Amit Kothari: All of the insight and then the detailed data year, so you can, so this is all of the boys detailed data, so you can quickly come up here and say so as soon as you say, basically Double Click on one.
Amit Kothari: Say on one supplier right he had one specular devices I just did all of your all of your insights will be refreshed by that see immediately within sub seconds all of the data what refresh, and this is all of all of this is millions of rows of data in our.
Amit Kothari: backend tables, so it is really easy to start navigating.
Amit Kothari: On these data sets, and this is company is very easy to customize right well, what are the categories, we spend most on anybody.
Amit Kothari: The top suppliers, all the standard questions which users asked for, then you can immediately now go to the holes data so see now all of this data is for this supplier.
Amit Kothari: So you can look at holds, then you can look at the payments, which has been issued to the supplier right, so all of this is.
Amit Kothari: At a lightning fast performance, you can do all this.
Amit Kothari: So this is my tables and then I can go to my receivables and I can do my aging details, so this is.
Amit Kothari: year the interesting thing is we're not you can go, we can do any as of data analysis of the aging, so we have something called as.
Amit Kothari: Far snapshot that we are not taking daily snapshot we just recreating it and the aging scenario as as on date by by just looking at the all the transactions, which is extremely hard to do.
Amit Kothari: You know, without doing this kind of a daily snapshot kind of architecture which will be it does.
Amit Kothari: Okay, so yeah so it's very simple to do almost all of these are actually live dashboard with our current customers.
Amit Kothari: Then we have the full GL reporting here, I can I can go from.
Amit Kothari: So let's talk let's let's look at this GL journalist, we are so I can go from my journal data directly and then this is my legit data data aggregated level with high level journals now I can from here, I can click to my er.
Amit Kothari: that's boots so from my leisure, I can go to my sub ledger see now I can go to my invoice details, this is one of the biggest thing which the one of the most popular thing with customers like is.
Amit Kothari: You can immediately go from legit or sub ledger and look at how my The sub ledger transactions affecting well ledger.
Amit Kothari: Let me talk about yeah so we have a lot of content here.
Amit Kothari: So we can go to otter management.
Amit Kothari: We have the full, as I said, this built around business processes, so we have looking back this one of the booking backlog building on order summary, so I can.
Amit Kothari: So this has the full business for assessment for fulfillment by category by backward items that backlog details I can go to my shipping.
Amit Kothari: stuff my returns my rma stuff my buildings and receipts, so all within this all of this building is is coming from financials so all within the same dashboard i'm able to now track the entire history of customer right to give you a gives you a 360 degree view of a particular customer.
Amit Kothari: So I think that's all I had i'll give i'll give.
Amit Kothari: i'll give it back to Rabie further explain his slides.
Mohamed Rabie: Thanks a lot great DEMO great content over there, if you allow me to share my screen.
Amit Kothari: yeah sure yeah.
Mohamed Rabie: Alright, so now let's tackle the second part that we're going to cover in today's webinar, which is the modern or actually the materialized approaches.
Mohamed Rabie: that most of the organizations, usually take in order to achieve that analytics go right so then all the organizations, whether they are Oracle shop organization or they have multiple solutions or even SAP or sequel and Microsoft stack.
Mohamed Rabie: organization, they would have multiple data sources within the source system right and within their systems where that was data coming from the RPS data coming from CRM even data coming from external entities and.
Mohamed Rabie: Local files and excel sheets right So the first thing most of organizations do is that the extract all of these data into a data lake nowadays right, so the map one to one of the data that they have over there.
Mohamed Rabie: After they move into the data lake be usually transform that data into a data warehouse so the stores to apply that transformation rules they start to put the data in a certain shape within the bigger House and also.
Mohamed Rabie: Once they build their own data warehouse they start to model, the data to make it more business relevant right, so they basically start to build their own stores schemas and snowflakes and.
Mohamed Rabie: Do their facts and dimensions linking all of them together in order to enable the business users at the end.
Mohamed Rabie: To start to do the reporting, so the problem we usually see in such cycle and basically our face this in all the organizations that I worked with here in the Emir region.
Mohamed Rabie: So they say it's really hard to push the data from the sources, up to the analytics because there is a lot of heavy.
Mohamed Rabie: Lifting that needs to be done to move the data from point A to Point B, because there is a lot of processes, I have to depend on multiple solutions.
Mohamed Rabie: I have to get my requirements across multiple and multiple of themes, so I have my own etfs team that needs to.
Mohamed Rabie: transform the data and I have my own modeling team that needs to understand the business behind my requirements and then put it in a certain.
Mohamed Rabie: analytical model and afterwards I have to go to my reporting team in order to make them be able to start and build my own dashboard.
Mohamed Rabie: But imagine that you would only own have only one solution that can be configured as your single point of truth that can directly connect to all of your business application, whether it was Oracle EBS or confusion as a B.
Mohamed Rabie: c or C or success factor ECC Hannah whatever business application that you have, in addition to the data warehouse in solutions that you have.
Mohamed Rabie: In just all of the data into this product and enable the business users to directly go in there and build their own dashboards in a.
Mohamed Rabie: Self service manner, without the need to rely back on it that's our goal as in court and that's what we're trying to achieve.
Mohamed Rabie: is to enable the business users to create their own dashboard in all on their own, without the need to revert back to it, and without the need to do any models in etfs and what.
Mohamed Rabie: So how do we do that, we do that, because of our beaten technology that is called the direct data mapping, on which the whole product has been built off.
Mohamed Rabie: So what happens within that edm is that when we load the data from your source system includes a smart enough to understand the path between every single point of data that we load.
Mohamed Rabie: And how does it relate to the other points of date all right, and then drew some sorts of a map, as you can see over here with them the meter rate of increase whenever you try to visualize your data, whether the quota or any third party solution.
Mohamed Rabie: and go encode that dramatically and our engineers mcafee start to throw.
Mohamed Rabie: A smart query routing between these data points, so we can understand the path between the data points ahead of time, so how did this help us so it helped us in when it comes to building star schemas when it comes to building snowflakes or comes to.
Mohamed Rabie: Doing or tracks and dimensions and whatsoever, because the need for that is now eliminated.
Mohamed Rabie: So imagine all the time you use to invest into building such a traditional star schema as, as you can see over here snowflakes and building your facts and dimension.
Mohamed Rabie: is now eliminated and all you gotta do is just to get your data and map it to then in quarter one one without the need to build these models so as you can see here in such a model.
Mohamed Rabie: We have the data within quarter flattened all right, this is the same format, in which the cool the data is residing within the source system.
Mohamed Rabie: We get it, as is from the source system into in quarter and our product is smart enough to understand the path between these data points.
Mohamed Rabie: To explain to explain more on this so, for example, i'm trying to build the analytics between the excel a module within my Oracle EBS and.
Mohamed Rabie: My F amp D, for example in kota automatically understands that in order to get the data from here to there, it has to go.
Mohamed Rabie: Through this path of data and to get you the insights that you need and that's all without any intervention from your site so that's the magic that includes that delivers and process.
Mohamed Rabie: So, how does that impact the business users, so instead of that the business has to wait weeks and a lot of time in order to get the reports.
Mohamed Rabie: They can easily have it within minutes right because all of the steps that you can see, above like about calling it preparing the data building the models.
Mohamed Rabie: Within the transformations that you need and whatsoever is now eliminated because, since we do not require any of these, and all of the data will reside with any quarter with symbol dragon drops and self service behaviors they can start to build and see their own reports right away.
Mohamed Rabie: So how do we do that, so we do that by ingesting all of your data alright using our product.
Mohamed Rabie: Within quarter we designed the data into our own data leak all right, and then we query the complex data and collect the data silos.
Mohamed Rabie: The different ones so say, for example, that you do not only have Oracle EBS or confusion, what if you have also.
Mohamed Rabie: within your organization SAP at salesforce and data coming from custom applications and whatsoever, we can easily interconnect all of these data together all right to then our own data lake and help you to analyze the data further and to start to build your own reports right away.
Mohamed Rabie: So let's dig deeper into the architecture of inquiry to understand more, how is that technically being done.
Mohamed Rabie: So, within in quarter, we have for logical components that data acquisition the shared storage data enrichment of data analytics.
Mohamed Rabie: Our data acquisition has around 200 plus connectors to the different data sources that can physically connect or whatever data source that you have within your organization.
Mohamed Rabie: Once we connect to your data source will start to apply parallel data loading and our mechanism and algorithm, which is the direct data mapping start to work to understand the past between this data.
Mohamed Rabie: right we store all of the information about our algorithm here into the Meta data.
Mohamed Rabie: That we have within our shared storage and all of the data that you're going to have from the different data sources that you adopt those any organization will reside over here in their data lake of unquote or data leak is based on Apache spark a data lake which is basically.
Mohamed Rabie: open a standard format known for the big data analytics really known for for the high compression ratio is a column or storage or so, you know the high performance of a web.
Mohamed Rabie: So whatever your data is coming from wherever it's coming from whether it's coming from sequel SAP Oracle it will always become a patchy for katy Perry get them, so we can easily interconnect these data.
Mohamed Rabie: Once we have the data over here we start to utilize our data enrichment components that basically relies on two parts, the first one is in court as engine itself.
Mohamed Rabie: That help you to do transformations on your data with your own logic build your own data flow with the self service matter or even by writing sequel scripts if you want to.
Mohamed Rabie: Also, we have under the hood of the quarter configured by default sport.
Mohamed Rabie: That allows you to write whatever script in language that you know whether it was a scholar our pricing by utilizing our notebooks that's very similar to Jupiter notebooks.
Mohamed Rabie: To translate whatever logic, they have into the script and apply it into the data that resides within our data right so once the logic is transformed the music will be written back over here into the Apache per cubic.
Mohamed Rabie: Finally, we have our data analytics component that mainly relies on our analytical engine or in memory engine which is one of a kind of number in the market and the reason behind that.
Mohamed Rabie: Is that, since we are using here column data storage or what engine is smart enough to only move the columns that you need within your data set.
Mohamed Rabie: From the party into the engine, and we also move it in a compressed way, so we do not have to decompress the data, so we only move the columns that you need within your reports and dashboards and we move it in a.
Mohamed Rabie: compressed way, and of course we rely back into the Informations we start healing the diary data mapping to understand the bathroom to in these data points.
Mohamed Rabie: Once we have the data, here it comes the second part of our designers component, which is the semantic layer that my colleague has been spoken off, which allows you to build your own views and make it speak more to the business people, you can easily have a semantic layer
Mohamed Rabie: built views by drag and drop, you can start to do your aggregations on the fly, you do not have to pre aggregate the data or anything.
Mohamed Rabie: And lastly, you can expose this view is either into inquisitors visualization which is available on the web application that we provide or the mobile application that equal to provide as well for the mobile application.
Mohamed Rabie: On ios on the android or even by exposing these data to third party tools and bi and visualization solutions to.
Mohamed Rabie: enable it to benefit from all of the the engine of a quarter and not being doing any transformations or anything and they become just a visualization hope for us so.
Mohamed Rabie: In short, like the benefit that we get out of this is basically two things first of all that, when I map the data from your source system into a quote, that I do not have to do any sorts of.
Mohamed Rabie: models, I do not have to build any star scheme as a snowflake or I do not have to pre aggregate the data to enhance the performance owner of that will be done automatically by the product.
Mohamed Rabie: So now let's jump into a quick DEMO that will show you basically how encode that can handle huge amount of data and make you be able to.
Mohamed Rabie: develop your own dashboard and an easy way without the need to revert back to any IP or anything alright.
Mohamed Rabie: So here, as you can see here is a quarters ui on the web browser so, by the way in kota is both cloud and on Prem solution.
Mohamed Rabie: You can deploy it basically anywhere, whether, if you have your own cloud, and you want to use equal that as a past platform as a solution, you can do this or, if you want to utilize our SAS offering tikun Buddhists, or if you have an on Prem.
Mohamed Rabie: environment, you can we can utilize any commodity hardware, that you have in order to deploy in quarter and use it for our analysis.
Mohamed Rabie: So our DEMO today we'll start by the regular flow that we usually take in order to achieve such a thing alright so let's start here with was the data tab I can see here on top, in which you will start to apply.
Mohamed Rabie: connectivity to whatever data source, you have so here, you have the list of all the connectors that you have the quarter, we have many more of them.
Mohamed Rabie: For the DEMO purpose, we can I use ready made Oracle application connectivity alright so here's the rp that we can connect to.
Mohamed Rabie: Once we establish that connection to your data source what you're going to do is that you can go to the schema layer that you have an equal right and the schema layer within a quarter is similar to any database that has.
Mohamed Rabie: Multiple tables within the relation between these tables and whatsoever for today's DEMO we're going to focus more on accounts receivable schema that we've built on top of.
Mohamed Rabie: Oracle EBS that we have so as you can see here the volume of data we're talking about 2 billion records of data right, and when we can build the dashboards we can focus more.
Mohamed Rabie: into the customer transaction lines all which has all the invoices information from Oracle EBS that has around have a billion record on its own right, if we go here to the diagram to look on how.
Mohamed Rabie: These TVs are related, you can see that there is no model has been built in here right, so you can see that all of these data path, and this is how exactly that it looks.
Mohamed Rabie: was an oracle EBS and how does it relate not only that you can see that we also linked with data from Oracle sorry from Oracle EBS with other data coming from jira services and desk and also from salesforce that can show you how easy it is to do such a thing with me.
Mohamed Rabie: So we can start here by exploring the data and start to build our own and first dashboard that we have alright.
Mohamed Rabie: So let's think of one of the most complex requirement that you usually get out of your finance team all right, I would say that the love pivot tables right so.
Mohamed Rabie: say, for example, you have a requirement for a pivot table that you need to build that has the quantity or the quantity invoiced.
Mohamed Rabie: And the revenue amount as well all right, and all of these are coming As we explained from the or a customer trs lines own alright, so let me take it from here, instead of the elites.
Mohamed Rabie: You can have it revenue amount quantity or the quantity invoiced.
Mohamed Rabie: right also let's see this categorized by sales channel.
Mohamed Rabie: All right, which comes from the customer account all right also let's see categorized by the party type.
Mohamed Rabie: comes from the participating and see this data segmented by the monitors all right, so we can have it here from the GL data table.
Mohamed Rabie: So you can see how easy it is that include the has picked up the visualization that you look for over billions of records of data right without any performance issues and without any.
Mohamed Rabie: need to do any models and if we go over here and click on say, for example here the revenue amount and you look for the query plan.
Mohamed Rabie: You can see that this visualization has been implemented across five different tables he we did not tell the visualization how to do the model right for how to do.
Mohamed Rabie: The facts or I mentioned the engine is automatically or actually the engineer magically understood.
Mohamed Rabie: How to get a link between the information that you entered from the transaction lines old table and the GL date, as well as the customer and the parties as well right and that's the beauty of in quarter.
Mohamed Rabie: So here, you can see here in the preview you we you can see, for each sales channel the party type of it and the month and the order.
Mohamed Rabie: The quantity ordered and the quantity invoice as well the beauty behind this visualization and this preview canvas that you can see is that we can easily locate.
Mohamed Rabie: Data quality issues within our beta right, so you can see that.
Mohamed Rabie: Some of these invoices does not have a GL day to it, so I can filter these data out by having here the monks name and the infidel filter and filter it has no none so I made sure my data is clean and ready right so once I do, that I can start to save these data all right into a new dashboard.
Mohamed Rabie: And now I have my first dashboard my first report was in my data right so as well as this pivot table that you can see here, what if I want to see listing data as well for my visualization right so for that purpose, I can easily add here in new insight alright have a listing table.
Mohamed Rabie: seems that I have Internet connection issue.
Mohamed Rabie: right here, it came back.
Mohamed Rabie: So here, I believe I have David for the customer lines all.
Mohamed Rabie: and have it here and let's press, save for this data.
Mohamed Rabie: So now, you can see, within my dashboard the I just created all right, I have to charge different first one is a pivot table that shows me all the aggregated information that I need.
Mohamed Rabie: As well as that I have the listing of all the Informations I need down in here right so whenever I click on any of these numbers that I see triggering for me say, for example, that.
Mohamed Rabie: I don't know this 29 number, where is it coming from So if I click on it, I can see the raw data that is coming out of this numbers over here in the listing table that I just created right.
Mohamed Rabie: And that's the beauty of in quarter since we are not doing any P aggregation for the data, so all of our calculations, but automatically run on.
Mohamed Rabie: Those are the transactional data that you have within your system, so you are pretty sure that whatever data, you have within your Dr P will directly affect the reporting layer that you have within your systems right so for last thing like what if I need to have a visualization in my.
Mohamed Rabie: Report so let's duplicate these.
Mohamed Rabie: charts right save this.
Mohamed Rabie: One and one let's edit this pivot table.
Mohamed Rabie: Let's have say, for example, this channel each month, of course, and the revenue amount, and I want to see this information in the form of our tree map for example let's give it a title here let's call it.
Mohamed Rabie: The map.
Mohamed Rabie: And then done so within the same dashboard you can easily use a variety of visualizations that in quotes and provides whether it was tables.
Mohamed Rabie: tables and even.
Mohamed Rabie: court, as you can see, and as we explained before like the beauty of that is that all of these Informations is already pre linked.
Mohamed Rabie: From before so that was the DEMO I needed to focus more on today.
Mohamed Rabie: For more demos if you're interested to see more demos from kota you can go to ww.co.com and then you can go to the product DEMO it has a variety of demos, whether I was getting started.
Mohamed Rabie: That was from netsuite for the financial for the HR we have basically are all demos that can cover all of your needs.
Mohamed Rabie: And if you want to try out the product on your own you can go to cloud encoding calm and then you can sign up with your business email over here to get free access and free trial of the product that you can try it out and whatever you want.
Mohamed Rabie: Right, so I believe now it's time to go and tackle some of the Q&A’s that we got here in the chat.
Mohamed Rabie: And in the QA section.
Mohamed Rabie: So the first question, I see Amit answered most of them, so the first question was about extracting the data.
Mohamed Rabie: When we extract the data, do we copied into include that yes, as we saw and architecture, we have our own data leak right which is very optimized and very smart, so we move all of the data from your source system into this data lake and accordingly.
Mohamed Rabie: We start to.
Mohamed Rabie: build analytics on top of it right, so we have other questions that I see i'ma tell does in court actually ingest the data from Oracle cloud, given that we don't have access to the db So yes, as our has explained.
Mohamed Rabie: We usually deploy and actually connect to the ECM after accepting whatever offerings that you have from the p ICC and from there, we can.
Mohamed Rabie: Directly ingest the data from fusion, so I saw that i'm it answered already all of the questions that you guys has posted so for the purpose of time, I see that we already covered the one hour that we needed so Lastly, I would like to thank you all for attending today's webinar.
Mohamed Rabie: As we said before, if you have any questions just feel free to drop us emails if you need more details about the product feel free to go to our website right Thank you.
Amit Kothari: Thank you.
Hosted by:
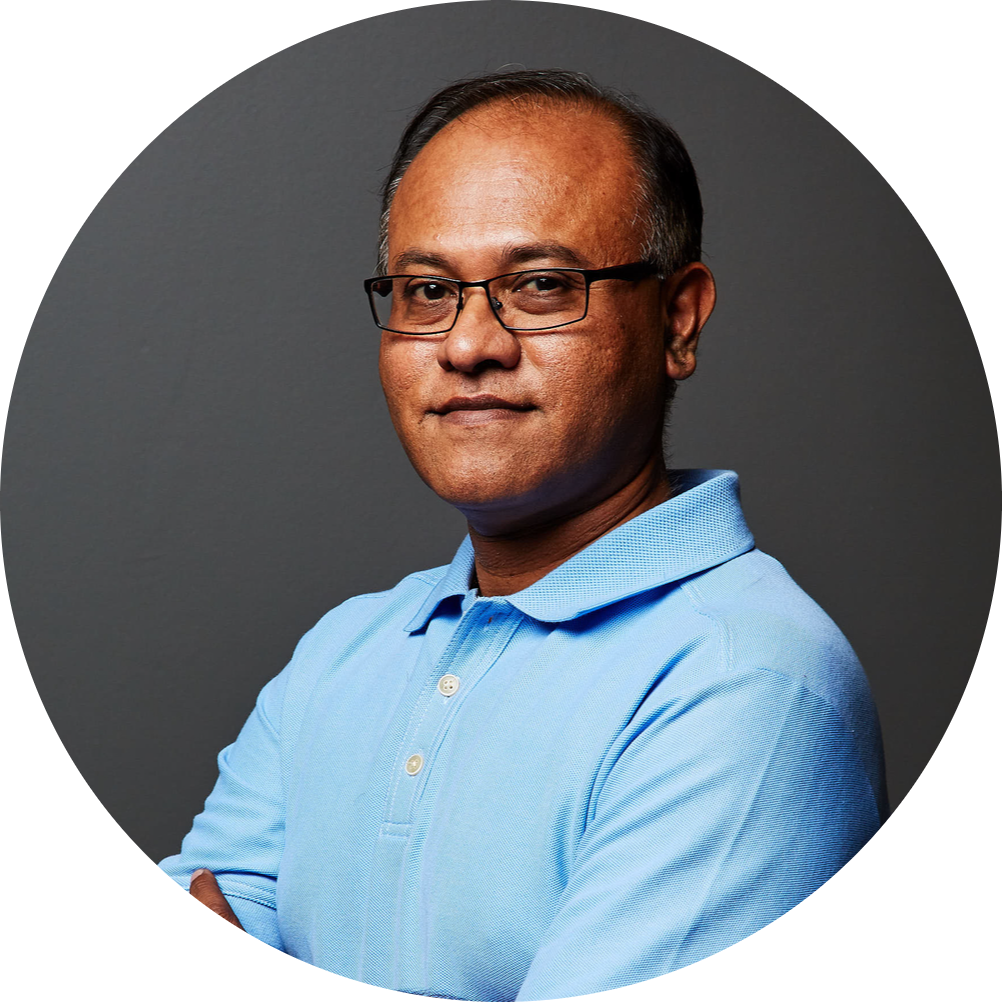
Amit Kothari
Director of Solutions Engineering
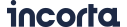
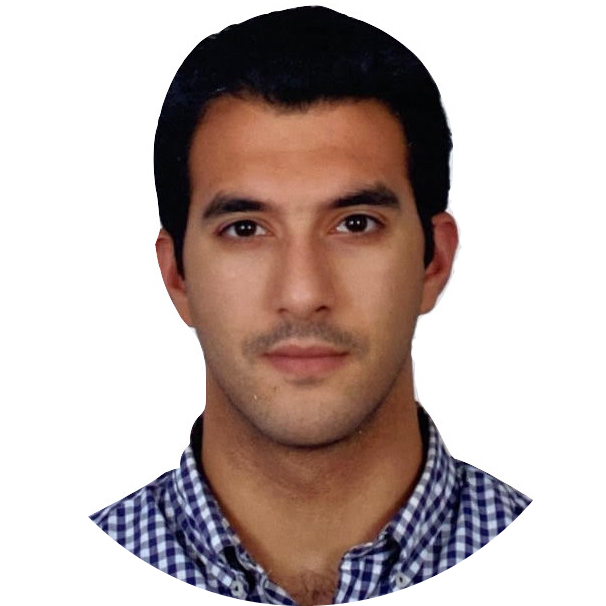
Mohamed Rabie
Senior Sales Engineer
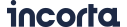