Death of the Star Schema: A Reality?
A Panel of Experts Moderated by Claudia Imhoff
For many years, the star schema has been a reliable design for creating the physical schema for business analytics. But time – and technology – march onward. We find ourselves in the enviable position where technological advances such as cloud have made the physical implementation of a star schema unnecessary. What does this mean for the business community and the technical implementers supporting multi-dimensional analysis? What is gained and what is lost as we move into a more virtual, cloud-first environment?
Watch the latest episode in our “Death of a Star Schema” webinar series. Hear Dr. Claudia Imhoff, Founder of the Boulder BI Brain Trust, moderate a friendly debate between luminaries in the analytics space.
Watch now to learn:
- Whether there’s a place for physical star schemas in a cloud-first world in data analytics
- The potential benefits to eliminating the physical star schemas
- Other factors that should be considered in implementing this new model, and what the downsides might be
Transcript:
Claudia Imhoff: So, welcome to today's webinar it is titled death of the star schema a reality, my name is Claudia him half and my slide is there's my bio slide you can read that if you want.
Claudia Imhoff: i'm not going to spend a lot of time on me because i've got much more people much more important people do to describe the only thing I will mention.
Claudia Imhoff: Is the boulder bi brain trust it is something for independent analysts and consultants if you're interested in it, we do deep dives with vendors, like in quarter for about three hours.
Claudia Imhoff: it's an open session, where you can ask any question you want, so if you're interested in that, by all means go ahead and sign up at BT bt.us okay next up.
Claudia Imhoff: All of our panelists are my lovely panelists here they are first up Tony bear Tony bear is the principle of db insight he is a veteran.
Claudia Imhoff: Industry analysts with expertise on how Clyde a cloud native architectures will transform databases most appropriate for this talk.
Claudia Imhoff: Prior to db insight Tony spent a number of years over a decade at ovum where he founded the big data practice and he is also a regular contributor to zd nets big on data series.
Claudia Imhoff: Next up my Bud Donna burbank Donna, is a recognized industry expert absolutely in information management with over 25 years of experience in data management and enterprise architectures.
Claudia Imhoff: She is currently the Managing Director of global data strategy limited and international data management consulting company and has worked with dozens of fortune 500 companies worldwide.
Claudia Imhoff: In the Americas Europe, Asia, Africa, as well as authoring several books on data management so welcome to you Donna as well next up, we have Paul coulson Paul.
Claudia Imhoff: paul's gonna sound really old he has more than 30 years experience in the.
Claudia Imhoff: Business intelligence analytics and data management industry, he spent 10 years with metaphor if you're familiar with star schemas You all know what metaphor is.
Claudia Imhoff: where he worked with Ralph kimball and was immersed in the world of the star schemas as Co founder and managing partner of stars off solutions, Paul has also led numerous engagements across the seven years.
Claudia Imhoff: i'm sorry across the entire data and technology space for large and very complex organizations.
Claudia Imhoff: Paul also spent more than seven years as the director of education for the data warehousing Institute.
Claudia Imhoff: The leading global provider of education, research and best practices advancing present and future data management and business intelligence.
Claudia Imhoff: And then last but certainly not least our sponsor himself Matthew holiday is here, he is a veteran software engineer and data analytics expert he co founded in court in 2013 after more than 15 years at Oracle and several years managing products at Microsoft.
Claudia Imhoff: With over 20 years of experience, oh paul's got to be.
Claudia Imhoff: Developing products and taking them to market Matthew has served in several key roles across the company playing a hand in nearly every aspect of in quarters growth and product development.
Claudia Imhoff: So there you have it, we have a stellar cast of characters Now let me talk a little bit about the agenda today.
Claudia Imhoff: we've got three things we're going to start the panelists discussion in just a moment as soon as i'm finished.
Claudia Imhoff: And then we are going it will do that for about 4045 minutes, and then we will take questions from you all along the way, please do enter your your questions into the Q amp a panel.
Claudia Imhoff: And we will try to answer as many as we can, before we hit the top of the hour, but stay on because at the top of the hour we're going to have a DEMO by Matthew.
Claudia Imhoff: Of in court and that's a critical piece you don't want to miss that one so make sure you stick around for an extra 15 minutes it's a bonus round there.
Claudia Imhoff: And finally, the last thing the recording of this session will be made available via email to you, and you can watch it anytime you want it'll be in the next day or so alright so without much more ado, let me go ahead and get started with the questions themselves.
Claudia Imhoff: First up i'm going to pick on you, Paul, because this is census is a star schema based thing.
Claudia Imhoff: I guess you've got the most experience along those lines, and the most history, apparently, so why don't we get started with you, why do we even create star schemas to begin with.
Claudia Imhoff: And then the second part of that is what what's the purpose do the star schema service today so tough ones for you, Paul go ahead and let her up.
Paul Kautza: Well, thanks, Claudia and it's a it's a pleasure to be part of the panel today, and I think Claude he asked me to be part of this because i've been around schemes are schemas for so long, and in that 30 years I can't.
Paul Kautza: I can't go back on those so I wish I could but anybody who is doing me for my career and I was, I always say i'd rather be lucky than good, and I was very, very fortunate to have worked for the company called metaphor.
Paul Kautza: In the early to mid 80s, all the way through 1994 when IBM purchased them.
Paul Kautza: At metaphor, we sold all of our.
Paul Kautza: products and services directly to the business community and Ralph kimball was part of metaphor, from the very beginning.
Paul Kautza: In just a tad bit of history, for those of you on the call that are under 40 years old.
Paul Kautza: This was a time before Microsoft even invented windows or delivered windows, to the public.
Paul Kautza: And it was also before apple invented the MacIntosh So if you look at what has happened in that period of time to technology you'll understand a little bit more about what we're talking about with incorporated today and different pieces.
Paul Kautza: To me Ralph was always about the business first and the technology Second, I believe that Ralph developed the stars came in for two fairly distinct reasons.
Paul Kautza: One is that it worked very well technically and it performed exceptionally well and allowing the business community to do things that they just couldn't do before.
Paul Kautza: And secondly it it allowed the business to really understand the data that they needed to make business decisions, the business can talk to us about.
Paul Kautza: What data was wrong what data they were missing what didn't meet their needs in our mission and metaphor, the entire time was all about solving business problems first and having the technology support that mission.
Paul Kautza: Unfortunately, I believe that as technology advanced through the years we lost some of that mission here and there.
Paul Kautza: But, for some reason the star schema has lived on and thrive through those years and we asked why, and I think that's part of our discussion today so i'll turn that back over with from a little bit of a story of a historical perspective.
Claudia Imhoff: You are a wonderful historian, though Donna, let me turn to you on that question because you also have quite a history with designing databases, you know design doing data models galore comments on why we have star schemas.
Donna Burbank: know I would definitely reiterate what what Paul had said I think so much of the the why data starts to humans have continued is because they're so intuitive to the business.
Donna Burbank: You know, I was in the data modeling session, and we had a business you've raised his hand and say boss matrix bus expects that's what I understand I understand my measures and the and the facts.
Donna Burbank: If you think of an excel spreadsheet pivot tables, you know that that sort of method of visualizing data is just really stood the test of time.
Donna Burbank: And, and I would also, you know second what Paul said, I mean technology has come so far, since the day before windows.
Donna Burbank: But is it apples to apples when we say death at the stars taking schema you know, do we have real time data streaming yes, do we have.
Donna Burbank: All these other tools, but is it the right tool for the right tool box, so I think you know the.
Donna Burbank: star schemas can be faster and there's a lot of more hardware and software technology, but that that method of visualizing data, as you know, I want to some by you know total sales by project by region.
Donna Burbank: is a really nice way to sum up in a star schema so where I like to say things have things evolve, you know, are there tesla's instead of you know, the old Ford model T.
Donna Burbank: Yes, but they also have wheels right so some things are still foundational we don't reinvent the wheel literally.
Donna Burbank: Because we have new technology we use that as a foundation so that's how I see with the stars game is a both and it's not necessarily one goes away I think there's just a lot of other tools to augment that.
Claudia Imhoff: yeah it's not just about performance either I mean if we if we think about a star schema.
Claudia Imhoff: The reason it existed was basically it pre joined the data for the business users in in a fashion that they could understand right we're talking about a physical.
Claudia Imhoff: Joining of customer and store and product and so forth, and all that, but is it is it just about performance, or is there is there more to it Donna.
Donna Burbank: I think a large part of it is the intuitive nature of it that that's aside from from performance like you said it's just such an intuitive way for businesses to under you know.
Donna Burbank: To for business users to understand again total sales by region by you know the what are you reporting on and what are you reporting by I just think is a really intuitive way for for people to visualize that.
Donna Burbank: And I think as Paul mentioned it's the business intuitive miss last word.
Donna Burbank: Is it is probably more important than the performance, especially with new hardware and software changes perform I don't want to say poor performance isn't important it certainly is.
Donna Burbank: But you have some bad designs now that can still performance right, but I think a lot of it is, is that business layer of it is just a really nice round on top of other architectures that can be added to really do that slicing slicing and dicing right that's.
Paul Kautza: Caught in in my experience, the the the star schema also allowed the business professional to be involved in the process.
Paul Kautza: To make sure we had that business governance around it, to make sure we had the definitions correct the integrations correct the right, the right pieces that that's where they actually understood the data and then they were able to use it more effectively to answer the business questions.
Claudia Imhoff: yeah well, we, the title of this is the death of the star scheme, I guess, we all agree that it's not quite dying, but the idea is We may not have to hard coded let's put it that way.
Claudia Imhoff: That what we what we now have in front of us is technology that would allow us to.
Claudia Imhoff: for lack of a better word virtualized the star schema we can we can bring it all together in a cash or whatever we can we can somehow materialize that view.
Claudia Imhoff: Without actually physically materializing it, and that brings up a question for you Tony and I suspect some others as well, so what's new about dynamic materialized views what's what makes this now possible today that we didn't have five years ago 10 years ago.
Tony Baer: You know what's interesting is that new material as soon as this kind of compliment star schema star seen have been basically here's how we physically instantiated.
Tony Baer: And with materialized views was okay here's the result of all that instantiate.
Tony Baer: On and you can sort of see this of an evolution over time, obviously, and it's really directly related to.
Tony Baer: Essentially kind of the equivalent moore's law of it, not just to compute but to all their other areas of IT infrastructure, whether it be storage, whether it be networking so on so forth.
Tony Baer: On and one of the interesting things about say about 10 years ago was that, with the ongoing you know.
Tony Baer: Basically, of declining cost of storage and various different tiers including memory, we start to see the possibility or the practicality of having it all in memory database.
Tony Baer: In memory not, not just for cash, and so we started to see this back about 10 or a dozen years ago with databases say like I said behind and one and one of the things that was at SAP originally promoted.
Tony Baer: was well you know it's all in memory, so you can basically you know instantly instantiate materialized view you don't have to physically persistent.
Tony Baer: And so, your question being what's changed since then, is that if you look at something like with Hannah it was I won't call it static, but it was like it was a single data store.
Tony Baer: What we can do today because of just the again because the impact of the equivalent of moore's law on the rest of infrastructure.
Tony Baer: And the emergence of cloud native infrastructure is that we can now bring this into.
Tony Baer: Bring multiple data sources in this and construct this virtual you know this virtual Star and, in turn, you know essentially a dynamic, you know materialized views so if i'm going to Point two.
Tony Baer: To one new mega trend here, it would be that now we can basically take an idea which was a signal from a single database, but 10 years ago, and now we can basically combine multiple sources.
Claudia Imhoff: All right, Matthew i'm going to bring you into the conversation, because this is kind of where quarter, you know shines, so why don't you talk a little bit about why materialized views what's what's going on here.
Matthew Halliday: yeah what I what I think's interesting is um this will make me even some older than I really am when I when I started that in quarter not included Oracle actually my career one gig of DRAM cost $19,000.
Matthew Halliday: And that makes it sound like I was probably born in the 1940s, but no it wasn't at that point, it was no possible, but that was 1997.
Matthew Halliday: Now we look at how much DRAM was a gigabyte of DRAM when encoder was invented in 2013 2014 and it was $5 per gig.
Matthew Halliday: huge difference right 19,000 to five gig and now it's less than the less than $1 per gig so when you think about the constraints that we find ourselves.
Matthew Halliday: Within and what can we work with and what's at our disposal, which is exactly what you know Tony was mentioning about the.
Matthew Halliday: The cloud the advent of the cloud the compute that we have the memory reduction it changes the way we think about him so when back in the 90s, we had to think about storing data on disk because that was the That was the option.
Matthew Halliday: And then, of course, the big one, we had then was trying to find discs that we're big enough and now it's like well, we got past that with you know cloud storage.
Matthew Halliday: And you know they had been in hdfs and then moving beyond that and forward now and the advancements we've made there.
Matthew Halliday: So it printed this perfect environment with things like columnar another invention so come together and revisit what we're doing for the business data, and while I agree that.
Matthew Halliday: The star schema is a conceptual way that people think about it, the thing is today that the way that they want to look at those schemas is ever evolving and changing.
Matthew Halliday: The business requirements change, and they were just too rigid and stuck and had too many expensive data pipelines in order to create them, so it wasn't but.
Matthew Halliday: The way that you look at the data is challenging or the way you might think about it, the way you get to it was the problem and so.
Matthew Halliday: Within quarter, and you know what we think is the way forward here is how can we get that so that can be done at query time, so if we can store data in memory, if we can know that here's the common ways that this data is joined together.
Matthew Halliday: And we can provide access to all the data and not like a cut down version of it.
Matthew Halliday: The user the business user then can derive and say this is how I want that data to be represented, and that should be a metadata layer
Matthew Halliday: The moment it goes from metadata to data pipelines and becomes very you know.
Matthew Halliday: Expensive food to do and it's going to be like Oh, you want to add one column that's going to take a few weeks, months, you know to be able to get that done.
Matthew Halliday: Whereas, you want to be able to say no, I need to do that today I need to answer that question five minutes, so it has to be a dynamic thing, it cannot be something that's physically kind of built in.
Matthew Halliday: In hard coded kind of just the very expensive needs to be plug and play it needs to be a way that you can evolve and change.
Matthew Halliday: And that's and that's why it has to be metadata driven at the end of the day, it cannot be something that's largely set in stone just does not give you the flexibility you're going to need.
Claudia Imhoff: All right, and Donna, do you want to add to this.
Donna Burbank: yeah no I think this is really interesting and it's I think not only the you know the speed and just the volume of data, we can manage now with these new tools, like in court and others.
Donna Burbank: But I think, also the business user has changed right it's no longer the day.
Donna Burbank: Where they have these static requirements and they throw it over the fence to it and and three months later, they get it, I mean they want to be handy, especially with these new tools that are more intuitive with metadata layers.
Donna Burbank: They want to be building their own and they want those real time, you know feedback loop.
Donna Burbank: So I think that's another layer as these tools have to be dynamic because people's questions are dynamic this idea of the citizen data scientists.
Donna Burbank: But, of course, being the always go back to basics kind of person.
Donna Burbank: That also you know, is a bigger need for things like form dimensions and master data and trusted data set so that these building blocks.
Donna Burbank: People are slicing and dicing of the right one, so you still need those fundamentals, but the tools have just come so far people people want to just get their hands and and look at it now, so I think that's another aspect.
Claudia Imhoff: yeah let me stay with you for a second because you brought up something and Matthew I appreciate your perspective on this.
Claudia Imhoff: We have seen advances, especially in real time data streaming the possibilities of instantaneous access to any and all data and so forth, so if that's The case is there any need to have some kind of batch processing these days.
Donna Burbank: i'll put that on I think so, and I think we wanted, we want to you know separate use cases right what's possible and what's needed.
Donna Burbank: You know, we had one client that was asking you know just recently, the finance team, please, we do not want it real time, I mean, these are financial numbers, we need to vet them and publish them, you know, at the end of the month.
Donna Burbank: And it's a both and just an example we've got a retail customer where they're doing it very advanced things with real time data streaming and tying that into.
Donna Burbank: Product inventory, so they they know their customers they're walking down the street with their cell phone.
Donna Burbank: This is a you know kind of a fast food there's a football game happening, they want to get inventory and faster real time.
Donna Burbank: And so that's definitely a need for real time data streaming and they want to get their numbers each quarter comedy products that we sell by region by.
Donna Burbank: By city, you know by location and get those conformed events and so it's it's both I think you know, sometimes when we have a tool we overuse it, so I, there is a place for slowing down and and kind of doing that and taking it.
Claudia Imhoff: To wait for things to come together before you jump and make a decision right, it does take a little bit of time to bring it all together Tony what are your thoughts on that.
Tony Baer: yeah well it's interesting to see a couple different things number one, this is the mainframe was dead right.
Tony Baer: And I was like yeah I would say the same thing, the same thing for batch is that.
Tony Baer: There will, I mean number one there always is going to be a need for historical data analysis and it's also going to need to have it's kind of like.
Tony Baer: Having your time analysis of data of record so there's a need for that the other thing also is that we talked a lot about Ai and machine learning Well, yes, you may you may apply the.
Tony Baer: Ai machine learning in real time, but when you're developing those models you're not doing this online and you're doing this offline So yes, you definitely.
Tony Baer: If there if there was one thing that really no militates that we still need the need for batch you know it's it's for Ai and machine learning just for that alone.
Tony Baer: You know, as I said, also, as I said, they're going to be some cases, especially when you have any case where you need to preserve an audit trail.
Tony Baer: Where you need to say look, this is this is this was the analysis of this data at this point in time, and you will need that from record.
Tony Baer: That being said, the other stream of thought you know sort of I guess pun intended.
Tony Baer: Is that I could also see from an operational standpoint, basically, you know streaming and batch coming together knows and it's not, as I said it doesn't basically replace the need for batch.
Tony Baer: But increasingly as you're doing what say inventory management and you're doing demand planning.
Tony Baer: You will want to have a real time component, you know I mean increase it they're going to be mini case let's put that way.
Tony Baer: where you will want to blend that real time component, and again I basically i'll sound like a broken record here when I talked about cloud native architecture.
Tony Baer: It allows us to have these different nodes that are basically, you know that are that are partition that can do various.
Tony Baer: Different jobs, so we can orchestrate all this stuff because we have this elastic cloud, they can dynamically orchestrate real time, so I guess the short answer i'll say to that is all the above.
Claudia Imhoff: Such a consultant Matthew any comments from you.
Matthew Halliday: I always find that the real time discussion fascinating because I think what most people think when they think real time it's not actually real time like real time doesn't technically really even exist in many respects, so.
Matthew Halliday: I have no customers that have come do this, we got this report you help us it runs and it takes me four hours to give us these results, but we need to run it on our production system, because we need real time.
Matthew Halliday: And you're like okay doesn't sound very real time you realize that the moment you start running that script that that sequel query.
Matthew Halliday: That it's at that point in time, that data is going to be so, if you started at noon and it finishes at you know, three in the afternoon.
Matthew Halliday: it's already the data is three hours old right it's even though just finished, it was quote on your production system.
Matthew Halliday: it's not real time, so the definitions of real time are always kind of very interesting when you take that into account.
Matthew Halliday: definitely agree, though, that the the need for trustworthy data that's that's really being refreshed at a much more frequent rate, and so what we have definitely seen as if you're doing a financial close.
Matthew Halliday: quarter and close, for example, having that data be from yesterday does not cut it like there's a lot of companies now they're trying to close that books, you know fortune 100 companies within like three, four or five days.
Matthew Halliday: And as part of that process, they need visibility into the adjustments that.
Matthew Halliday: That they post right they make journal adjustments when you post those you need to see where you are what's the state.
Matthew Halliday: And so, being able to have those frequent updates as frequent as five minutes means they can make an adjustment and then see exactly how does that reflect.
Matthew Halliday: And so, this kind of merging I think of what started it was a trend analytics and analytics is moving now more into the operational analytic side, where people are using it they're not going into the application to figure out where they stand.
Matthew Halliday: They using the analytics to figure that out, and so, for them, it needs to be that way.
Matthew Halliday: Then you see technologies like you know cafcass streaming way is like there's these great things you can take, and they can push data into places, you can store that data.
Matthew Halliday: But then you can do batch updates from that, depending on the on the business requirement of what they need so definitely seeing a lot of interest in.
Matthew Halliday: more frequent data and seeing it much more closer to the action that the person takes, and I think that's The important thing it's like.
Matthew Halliday: In that job function, what I do what am I going to do next, and I don't want to be held up in my job right, I want to be able to move fluidly between analytics and the applications.
Matthew Halliday: The moment you hold me up and you tell me i'm gonna have to wait a few hours to see the the effects of what I just did that doesn't cut it anymore that's why people get frustrated and it just slows down the whole process.
Claudia Imhoff: Well, I think we're getting a couple of questions in so let me move to the.
Claudia Imhoff: next question because it may help.
Claudia Imhoff: answer one of the questions, let me tell you what this person's question is and then i'll i'll ask you my question.
Claudia Imhoff: Karen says how's the performance for virtual joining of data and that goes right along with the question that that I wanted to ask Tony and and you also Matthew.
Claudia Imhoff: What about data virtualization does the data have to be in one place, or can we can we virtualized data, excuse me data together Tony, let me start with you, does the data have to be in one place and G, what about performance if we virtualized data.
Tony Baer: Well, I mean, if anything, as I said again the for the club broken record is that doesn't have to be one place, but more but more of the point, though, is increasing the data is not in one place.
Tony Baer: I mean we and we it's funny because you look back on on recorded history, at least with regard to data warehousing.
Tony Baer: Only back to the days of the you know the enterprise data model, the need the galactic enterprise data warehouse we've always tried to.
Tony Baer: We always try these attempts to put it all in one place, and you know 10 years ago with the Okay, we can put it all in the dupe and we found that each of these cases, it was not the answer, there was always some data that was going to be some you're someplace else.
Tony Baer: What we're finding now is that you know we have a lot of options and the thing is the answer in terms of whether we virtualize or whether we bring it into one place is not there's no silver bullet.
Tony Baer: In some cases it's going to make a lot more sense, you know, to push you know push down the process and force the processing to data.
Tony Baer: and other cases it's going to it's going, you know, going to be the opposite it's going to depend on on a number of different factors, you know one is basically your new.
Tony Baer: service level requirements it's also going to be the overhead of basically moving all that data and the fact is maybe we only want to move let's say a digest of that data or just the results set.
Tony Baer: In some it's just all going to depend so it's not it's not going to be an easy that you know there's not an easy answer the question, yes, the answer is going to be basically on.
Tony Baer: Is that know the data will not you all in one place, the question in terms of where you process, it will will vary.
Claudia Imhoff: Okay Matthew comments.
Matthew Halliday: there's always a database, I mean sorry there's always a spreadsheet right it's always a spreadsheet that's got something that someone wants to inject.
Matthew Halliday: yeah I think ultimately how I feel about is this way it depends on the data that you're your query so when we talk about.
Matthew Halliday: These different data sets they're going to be very different so let's say i've got i'm a thermostat company and i've got a smart thermostat and i'm getting tons of data being sent to me.
Matthew Halliday: The way i'm going to handle that situation is going to be different than the way I would want to look at my financial accounting.
Matthew Halliday: And I got to think about those two from very different perspectives, so one of them, probably, you know the thermostat I might be using Kafka streams Lambda.
Matthew Halliday: headless servers and i'm doing a whole bunch of stuff to land it in my data lake if i'm looking at my financial data.
Matthew Halliday: The way i'm going to approach that problem would be slightly different expecting the database to be able to run these queries is you know where it is like running and let's say it's an oracle database run it there as i'm going to run you know data.
Matthew Halliday: or queries against this data set and they expected to perform and i'm pulling from 3040 table joins in one query to be able to get it it's not going to work.
Matthew Halliday: In you might be lucky and get it to work but it'll probably take 24 hours in some cases, you know it'd be like really, really expensive to do.
Matthew Halliday: So, having a system that can actually handle those queries I don't think that data has to come out right.
Matthew Halliday: So, in most cases replicating that data as as being able to have it in that form, but then have a set of technologies that you can use against it, that can enable you to run queries.
Matthew Halliday: That can do those kind of joins and I think that's the critical linchpin in all of this.
Matthew Halliday: I think one of the reasons why we have star schemas is because three and a half or relational models which you find in this business applications they don't perform the worst thing you can ask them to do is.
Matthew Halliday: is to run a query at scale across multiple joints and the reason we all know, this is memories, to call up you know, to get like order stuff and get.
Matthew Halliday: updates on our borders and or even you know you call up about insurance or something and people say what's your record, you know your customer numbers customer ID.
Matthew Halliday: And then they always say the same thing my systems, a little slow today and it's just bringing up one customer ID and you're like.
Matthew Halliday: you're like, why is that so slow and if you're going to say like hey let's look at all our customers and do something with some analytics pure like.
Matthew Halliday: Are you crazy it takes like five six seconds, just to bring up you know 10 seconds, just to bring up one transaction, let alone look at a billion of them a 2 billion of them and so.
Matthew Halliday: In reality, like that was the core problem and that that's ultimately what we set out to fix it in quarter was like can we make these data sets actually perform at the analytical scale without the need to remove all of those joints.
Matthew Halliday: The starsky most great for removing joins but the cost of removing those joins us in the data pipelines which gets in the way of you, reducing the time.
Matthew Halliday: To get the data ran to build them and all of those kind of things, so I think the data has to be replicated, it has to be in something that gives you cloud gives you a Leicester city scale.
Matthew Halliday: Now, but also not just that, because scale doesn't just fix that joint problem it's probably a longer answer to the question to explain exactly why but yeah it's it needs to be a bit more than that.
Donna Burbank: Though so Matthew i'm curious when when tech support says that do you see, I can help you with that solution we can help you, with your performance issues when you can't find the customer number.
Claudia Imhoff: It must be tempting when you get someone saying that.
Claudia Imhoff: We had a question come in that's kind of appropriate at this point, and that is, with the district, that this is from super Thank you very much, with distributed data across multi cloud and multi accounts in each cloud is virtualization or virtual art of virtualization tools, the future.
Claudia Imhoff: You know, do you want to respond to that Matthew is if he does go on about the data mesh which Tony.
Claudia Imhoff: i'm sure you would love to answer, but that may be a longer answer than we have time for but but certainly the idea, our virtual look at our virtualization tools needed today.
Matthew Halliday: I think it comes down my pithy answer to this is it really depends on whether you like dealing in aggregate data or detailed data.
Matthew Halliday: And what I mean by that is, if you want to aggregate, then yes let's say I got two data sets and two different cloud storage is, and I want to run something up to an aggregate level and then.
Matthew Halliday: Transport and try and join that data at the aggregate level.
Matthew Halliday: You can do that, but if I want to actually know and there's a relationship between it and the more detailed level, I want to dive into details, I want to see customer churn versus you know.
Matthew Halliday: Customer tickets and my ticketing system, for example, if those two different data sets from two different places.
Matthew Halliday: I can only deal with it an aggregate level, so it might be Oh, we can just look at customers and look at the number of tickets they've you know submitted over the last.
Matthew Halliday: You know, six months and then look at a rate of churn, but if you wanted to go deeper into that say, well, no it actually depends on the type of ticket and it depends upon.
Matthew Halliday: You know how long that took it took it took to close and the more detail, you kind of go in all of a sudden, you realizing you need to join at the detail level.
Matthew Halliday: The transaction level because that's where that's where the interaction happened in the world right interactions between people don't happen that aggregate levels, they generally are individual transactions, when we deal with the aggregate.
Matthew Halliday: we're losing a lot of that nuance and I think the opportunity to really understand your data and make your data work in a more interesting way.
Matthew Halliday: But a lot of people become very used to dealing in aggregates and not having that ability to see and just have to trust those aggregate numbers and not be able to drill down and verify and certify.
Matthew Halliday: Are these numbers true can I trust them should I make a decision based on them, and so that I think is one of the reasons why we have challenges with organizations moving to truly being data driven.
Matthew Halliday: Because oftentimes the numbers don't give them enough of what they want, because they're dealing in aggregates and not detail.
Matthew Halliday: well.
Claudia Imhoff: Go ahead Tony.
Tony Baer: what's it gonna say at the risk of sounding kind of I guess kind of like what I hear the fact is, I mean I I mean, I agree with Matthew that basically that you know it depends on which level, you want to look at the data.
Tony Baer: The fact is, though, that there is definitely a role for aggregates, I mean the fact is that when you're first starting your search you're not going to start on the details.
Tony Baer: So there is something for basically kind of like what we used to call exploratory analysts do the exploration, to see what the what the questions me to ask what's.
Tony Baer: What are the details that we need to see, and then you know Double Click down to that the other thing also, though, is, and this is just getting to the whole idea of multi cloud.
Tony Baer: Yes, in reality, most organizations tend to have one of everything.
Tony Baer: And that's and that and the cloud is not changed that they're very few organizations that are actually.
Tony Baer: Going all in on one cloud you do see a few poster children and you'll go to let's say.
Tony Baer: You know, you know your reinvent conference or you know or any of the other folks and you'll see these posters on like we've all Community we've all committed to.
Tony Baer: You know, to aws or azure or Google that's the exception to the rule.
Tony Baer: On and it's basically repetition of the same pattern, we saw with enterprise systems, you know, there will be lots of database different.
Tony Baer: One part of that organization will have Oracle know they'll have some of you will have the sequel server and so on, so forth, and same with data warehouses um.
Tony Baer: So, yes multi cloud is going to be reality, I say, though, that going between different clouds is something which you want to make more the exception than the rule, because there's so many operational complexity there.
Tony Baer: And so what I would say, with something like that is key, is, if you are dealing with data in multiple clouds keep that very, very exploratory and I think i'll.
Tony Baer: echo what Matthew was saying here's a few of them want to get serious, you are going to have to you're going to have to bite the bullet and move some data.
Tony Baer: That being said, I also expect at some point I don't want to open another can of worms.
Tony Baer: But some point you're going to see some cloud providers start to basically relax on things like eagerness charges, but even if they relax and egress charges is still going you're still gonna have overhead.
Tony Baer: Moving data, so I don't want to you know, be a pollyanna there, the fact is yes multi cloud is going to be reality, basically, I would keep your dealings with trying to bring an aggregate stuff together across multiple clouds to.
Tony Baer: Make that you know minimize that as much as possible but yes, at some point, you will have to bite the bullet.
Donna Burbank: Time in on the virtualization I mean data can be stored in multiple places, but the these be governed centrally, and it doesn't mean one person making the decision, but it just needs to be mine done mindfully.
Donna Burbank: Oh right if you are getting you know whether streaming data you don't have to or stock market trades again makes sense i'm not going to replicate an existing third party data source that i'm.
Donna Burbank: querying from that's I think a good, you know way for virtualization or or an integration.
Donna Burbank: But, and I think Matthew touched on it i'm trying to get a single view of my customers are things like master data.
Donna Burbank: that's really hard to do in a virtualized what way you're going to have some of that redundancy, so I think you want to pick your battles, I think, with the technology, yes, you can distribute where that makes sense or it's a data source you can't own.
Donna Burbank: We were working with the university and some of the different colleges literally couldn't you know share their data so that that was a good case for some federation, but I mean virtualization but.
Donna Burbank: I think in a lot of cases you do kind of have to get that data in one place to do that, you know rationalization and joining.
Tony Baer: yeah so you might be like the same cloud you'll have.
Tony Baer: Multiple databases and so i'm going to be, are you there would be a case virtualization.
Paul Kautza: I don't know if we're going to get into the business side of the governance.
Paul Kautza: And those pieces if we're going to have time to do that, but I think that's part of that's part of what what comes into all of all of the everything we're talking about as an overarching perspective.
Claudia Imhoff: Well dang Paul I was just gonna.
Claudia Imhoff: So yeah.
Claudia Imhoff: What does need to be considered, you know we are talking about distributing data we're talking about creating virtual stars and all kinds of things like that.
Claudia Imhoff: There was a question that came in that kind of leads to that, and it was from Kevin he says, address the issue of time.
Claudia Imhoff: slash complexity, it takes to add modify manipulated traditional dimensional model in a large corporation versus some of the newer technologies, such as in quarter.
Claudia Imhoff: One of the things that I want to bring into that conversation is how do we govern this environment if we are going to make virtual stars and have them.
Claudia Imhoff: Willy nilly being changed or spun up or whatever not Willy nilly hopefully not but certainly many of them being spun up and that sort of thing so Matthew I wanted I wanted the spotlights on you right now, I think, how do we govern an environment like that.
Matthew Halliday: yeah, so I think.
Matthew Halliday: That role still exists right this, we still need to make sure that.
Matthew Halliday: The revenue numbers, the correct number that we don't have different definitions and that people are having that, and so this there's this element of trust and how do I know that I can trust the data that i'm.
Matthew Halliday: i'm using, so I think there's two pieces one is being able to get the person that understands that data set so.
Matthew Halliday: In every analytics project, regardless of the solution, using even if you're still you know coming from the 80s and you're still doing exactly the same thing we did in the 80s.
Matthew Halliday: You you literally still need to understand your source, you need to understand what are the important columns one of the calculations, how do I create an end result.
Matthew Halliday: That doesn't change, I think that it's how you get to that has fundamentally changed now.
Matthew Halliday: The star schema was a rigid way of enforcing it, it was a a schema level data model level of way of doing it.
Matthew Halliday: If we think about that, now as being an application way of doing it in the sense of we need set of processes, we need a way where there's metadata that you can put in that you can grab those columns you can give.
Matthew Halliday: Meaningful labels and descriptions and various things like that, so that people who using that data can trust it and and can understand it.
Matthew Halliday: I don't think that changes so it's not that the business users unnecessarily just saying hey I want to get this, and this, and this it's given them the freedom to be able to say.
Matthew Halliday: Oh i've got this table it's got 250 columns on it, and you know it's I in the star schema model you're generally be asked you know what are the important columns you want, and maybe you've pirate down to like 20 columns.
Matthew Halliday: And then there'll be other dimensions have come from other tables and you build it out, but.
Matthew Halliday: Some of our customers have 80,000 plus columns inside of there and quarter installs at any given point they might be using 3000 a day.
Matthew Halliday: And so, being able to give them like the flexibility to pick Oh, I want this column I didn't use it yesterday, but today I want it it's already at the same grain it's already the same.
Matthew Halliday: tables that have already used before it's very easy for them to be able to leverage and use that and the person who's making that data available.
Matthew Halliday: doesn't have to make these limiting assumptions that kind of reduce the.
Matthew Halliday: The the freedom that the business user gets because.
Matthew Halliday: They can't just bring in everything because they say it'll take too long it'll be too expensive, we you know we've got to kind of look at the what's the cost of that data residing you might never use versus the benefit.
Matthew Halliday: And so that was the trade off that we're playing, but in this this kind of World War now it's that governance layer being able to say here's a published data set.
Matthew Halliday: And if someone says they need to make a change to it, you can make that change in a few seconds not being like.
Matthew Halliday: Okay, let me get back to you in 12 weeks I think that's that's part of that issue and so really I think every organization should be asking themselves like what's the metric of success if you're.
Matthew Halliday: If you're a marketing person right, you know you're looking at mq l's and pipeline that you've influenced right that's that core metric.
Matthew Halliday: If you're an analytics team like, how do you know you're doing a good job well, I think one of those metrics that you might want to look at is how long does it take to respond and actually bring in just a new dimensional column to an existing.
Matthew Halliday: analytical query if that's something that takes you weeks to respond to business i'd say that's not a that's not a great great right there if it's something you can do today.
Matthew Halliday: In you know the same day that the question comes in, then you know you're doing something right and so you've that's got to be the goal of the objective, I think, to get to that point and, of course.
Matthew Halliday: Make sure it's done in a way that the people using the correct data, so its freedom or the way that they interact with the data versus, let me just spin up infinite star schemas and just kind of create things that you know i'm i'm you know i'm sorting.
Matthew Halliday: Ahead of value by distribution they mentioned like something wouldn't make absolutely any sense right.
Matthew Halliday: you'd be you'd have multiple lines and an invoice and then you're going to use the the header amount and then.
Matthew Halliday: multiply it by the number of lines and your numbers, would be completely wrong, so you have to prevent people from doing things that are inherently are wrong.
Matthew Halliday: And so just giving them free access to the data isn't the way to do that, so that absolutely has to be governance control and that'd be something i'll be showing you the DEMO of the top of the hour is i'd be How does that look.
Claudia Imhoff: All right, let me bring it back around, though, to the the overriding reason for a star schema and that's the business involvement understanding the business needs up front and Paul, I want to turn it back to you how do we govern or what.
Claudia Imhoff: it's not so much governing, but that is certainly an important part of it, but how do we keep the business needs up front, when we can spool up a you know, a virtual star what, what do you see is as being maybe a bit problematic, or perhaps something to focus on.
Paul Kautza: yeah well i'll start with I don't believe that the star schema needs to be physically instantiate okay just i'll get that on the table, I don't think we need to do that.
Claudia Imhoff: However, from you.
Paul Kautza: Okay, however, I have watched this my whole career as a technology advances data visualization cool tools came out because people's business wasn't getting what they wanted, so they could slap it right out of any database they wanted and get answers.
Tony Baer: That were wrong.
Paul Kautza: And going through that process, because they didn't have the business governance side of it, they just had results coming in and I don't want to see us as technology advances keep repeating that problem.
Paul Kautza: So the business side of data governance to me and putting this together is really the value, one of the huge values.
Paul Kautza: Of a star CMO or some conceptual equivalent that the business can bring in you can bring into the business and talk about things like in consistencies across the data, you can take.
Paul Kautza: four different data sets that deal with loan Origination and slap them together on the right keys.
Paul Kautza: And this has happened to me over and over and the financial institutions guess what the business has different ideas of what that definition is.
Paul Kautza: And they don't even realize it until you sit them down and get them to talk about it and put it on the whiteboard and then give you the transformation rules to make that correct the other side of it is how many.
Paul Kautza: derived attributes, have we had in star scheme is that that attribute doesn't exist in any data any place it's in the business has about what combination what pieces.
Paul Kautza: of data Do I need to get an attribute that I can do analytics against that is critically important to me and that's where I think the star schema still has.
Paul Kautza: A tremendous value in having the business having the conversations with the end with the with the with the technology group and vice versa, and that's.
Paul Kautza: Part of the hard work and rolling up our sleeves that we all run away from from the last 30 years and it doesn't go away, just because we give great technology which is great technology, we have to.
Paul Kautza: ferret that down to are we doing the right things, with the right data, and I hope the last point i'll make is that kind of 8020 rule.
Paul Kautza: A lot of stuff we're doing and the and the high end technology is to take care of the top 20% of this market rough give or take.
Paul Kautza: don't forget there's 80% of the people that are still trying to.
Paul Kautza: get ahead of the game just by being able to do the right things with their own data not Ai not ml not all of the different pieces we're talking about but there's a huge swath of the marketplace that are still struggling to get the basics right yeah.
Tony Baer: yeah can I jump in here.
Claudia Imhoff: Which is that there are no Oh yes, you can very quickly, we got about a minute left.
Tony Baer: gotcha Okay, I just want to hop on a couple things which is basic Toma governance and metadata and point you kind of refer to this in terms of game, this is to speak the same language.
Tony Baer: Was it Ralph kimball who was our thing was in one said that the only goes into an organization that very often they're going to see multiple definitions of customer.
Tony Baer: And so I think what it's all going to is one thing this all boils down to is that we need to have we need to be speaking of common language with metadata, we need to be all be reading from the scene, you know sheet of music so i'll just leave it at that, for now, Claudia let's move on.
Claudia Imhoff: Okay.
Claudia Imhoff: All right.
Paul Kautza: My point my point is that only as the technology can't solve that piece.
Tony Baer: No, no, well, I mean that starts to start to get into how do we govern this another question, Claudia I know you want to move on.
Donna Burbank: But i'm going to just think we're all agreeing that like tempering the business conversation that might take weeks to agree on what total sales means should be separate from the fit your physical limitation be so brittle once you decide that.
Donna Burbank: The other eight weeks, it should be instantaneous once the business.
Paul Kautza: Correct yeah.
Claudia Imhoff: we're all in agreement on that.
Claudia Imhoff: One yeah well I think what the bottom line is.
Claudia Imhoff: Technology can do a lot, but we still have to stick to the methodology of how do we get the business requirements for for that technology to shine otherwise.
Claudia Imhoff: we're going to you know garbage in garbage out we don't know what we've got the business users need to have some kind of a roadmap.
Claudia Imhoff: metadata or a data model or whatever it is, but there has to be something that they can follow, and we have to gather that from them as well, so I think.
Claudia Imhoff: i'm going to i'm going to kind of wrap up the the the panel part on that note I think that's a good place to.
Claudia Imhoff: To start answering some of the questions from the audience so with that please audience i've been watching the chat as much as I can, and holy cow you guys have been putting all kinds of questions and comments in the chat.
Claudia Imhoff: Please move them to the Q amp a so if the if it isn't legit question, but let me start off with this first one it's it's been waiting a long time to be answered, and I didn't have a place to fit it in.
Claudia Imhoff: But now i'm going to how do you think data lakes fit into all of this, so Matthew, let me start with you and Tony I got a feeling you're chomping at the bit.
Matthew Halliday: yeah so I alluded to a little bit of this earlier on, and when a man says about one of the challenges used to have was finding discs that were large enough.
Matthew Halliday: And so you know, hence hdfs came in that will be able to put together multiple disks and make them appear and feel as one I think dealt add data lakes and especially things like delta lakes right being able to now.
Matthew Halliday: easily taken incremental updates, not just in a pan data store where you've done things but be able to bring those in.
Matthew Halliday: Definitely, I think, is a strategic and make it good way to think about where, am I going to store that data, I think that.
Matthew Halliday: Also, in conjunction with data formats open standard data formats you think now paki is obviously now the the clear winner in this fight.
Matthew Halliday: that's been out, you know as awkward as a contender for a little bit, but it's obviously parquet.
Matthew Halliday: Has is a much more robust better you know data format for storage layer, and for that we then seeing.
Matthew Halliday: different options for being able to interact with that data and to me that's what's exciting about this it's.
Matthew Halliday: it's that ability that you're not tied in like you put your data historically inside of a certain vendors database.
Matthew Halliday: it's resided in that database, so when you think now about data storage, you can think about that truly gives you the flexibility and the options to try different analytical engines right, you can try and coordinate you can try something else.
Matthew Halliday: The data is there you already have it so having it really opens up.
Matthew Halliday: I think a world where we'll probably start to see more purpose built solutions that can leverage that data, so there might be certain types of technical challenges with the data that you have.
Matthew Halliday: That lends itself better to one solution than the other so, for example, again.
Matthew Halliday: thermostat data coming in you're looking at that you want to run machine learning models to help kind of predict.
Matthew Halliday: You right well use a completely different engine to do that type of query then something that says, I need to figure out an EP trial balance report from my financial statement two very different technical challenges.
Matthew Halliday: And and honestly I don't believe a tool, like a single engine or query engine could address both of those because they're really radically different.
Matthew Halliday: And so that's why I think this is exciting, because it really opens up the door to many opportunities and, of course, using the elasticity of the cloud.
Matthew Halliday: it's a win, win in my mind yeah.
Tony Baer: The risk of being buzzword compliant hear a lot about what we're talking about here is the data lake house and that's a very.
Tony Baer: that's a very fuzzy concept it's in the eyes of the beholder but essentially what what we're talking about here is that.
Tony Baer: we're seeing the confluence one is Matthew is saying oh there's an industry state those become kind of consensus industry standard formats both getting your.
Tony Baer: effect on and foremost and which is, which is parquet that's becoming kind of default, so you know column or storage format of choice in cloud storage and essentially we have you know you know Amazon s3.
Tony Baer: Which become a de facto standard it, you know, basically, you know protocol you don't necessarily have to physically stored in St but you're seeing lots of.
Tony Baer: You know object storage systems, you know come out, you know which basically have s3 compatible use s3 API.
Tony Baer: And the result is that you're saying you know data lakes that are now saying oh we're now adding acid capabilities that's kind of like with delta links all about.
Tony Baer: Reducing also allow the traditional folks the journal data warehousing from say hey we can now basically treat that object storage, you know and basically and do direct your direct query you're using let's.
Claudia Imhoff: say your.
Claudia Imhoff: API.
Claudia Imhoff: You blanked out on us a little bit there Tony I think the last sentence at least I didn't catch it, but I hope everybody else.
Tony Baer: Okay, it was essentially what we're saying is at the data and the data storage layer we're seeing a convergence is flowing both of the data lake folks and from the database folks.
Tony Baer: And, which means that it gives us a lot more freedom at the software layer to virtualize or physically instantiate that data.
Tony Baer: Hello yeah.
Claudia Imhoff: Sorry, my Internet went down for just a SEC all right, let me move on.
Claudia Imhoff: Next, questions and they're a bunch of these about well are if we're not going to do a star schema, what are we going to do what's the model look like I think we've all agreed that the star schema.
Claudia Imhoff: is in fact not going anywhere for those people that need that kind of capability, but are there other data modeling techniques that we should be looking at to store the data, one of them that's mentioned in a question is about the data vault.
Claudia Imhoff: Is that perhaps the way we should design the data for the underlying layer in quarter.
Claudia Imhoff: And let's start schemas evolved from that i'll just throw it out there, what do you guys think.
Paul Kautza: i'm just going to let them down with the technical side of it for me.
Paul Kautza: The the data volt could be a wonderful aspect on the back end side of it for the technical piece I don't think it helps the business side of it at all and that your question, I think, was from more from the business perspective.
Paul Kautza: You know, and having a a visual star schema for the business may not be what you need either having a a.
Paul Kautza: You know, in my business I call it the business dimensional model, where it was a instantiate of in just English terms to the business and in rows and contacts and dimensions, how they think.
Paul Kautza: A mechanism to talk to them to engage them to say is this what you really mean by this or how does this role into here our these how do these people pieces fit together and have that conversation.
Paul Kautza: So, at the end of the day, you have a conceptual design from the business that they understand that I can give to any of the technologists to say.
Paul Kautza: This is how we think about this here are the definitions here are the how how these derive derived attributes are actually contrived and be able to do those things in an effective, efficient manner, from the back end.
Claudia Imhoff: Okay.
Donna Burbank: We have some customers using data vault and I almost called a sort of an intelligent landing area where I mean you're sort of kicking some of the business requirements down the.
Donna Burbank: down the road that it's modeled in a way that you can defer some of the decisions or it's flexible.
Donna Burbank: But with that flexibility comes a lot of complexity, I think I saw that and one of the chats to to to then take that vault and and make it to something that, as Paul was saying is consumable into walmart we're actually going through that right now.
Donna Burbank: it's a bit of a challenge, so you just again we keep hitting the point of consider the use case and it does have its place for kind of again, you know aggregating a lot of different.
Donna Burbank: areas in a kind of flexible way, but it is not intuitive for the average average user and that's a big part of these abilities.
Claudia Imhoff: yeah okay i've got I think about out of time actually but Matthew there was one that came in and maybe you could give a very short answer before we go to your DEMO because I really do want to watch DEMO.
Claudia Imhoff: The question is, can you please elaborate on the quote published view you've mentioned as the data consumption layer
Claudia Imhoff: He says it completely agrees with your points of turnaround time for a few simple attributes to be added.
Claudia Imhoff: i've taken the path of starting with a strong semantic model operationalize it with technology that is decoupled from my semantic layer
Claudia Imhoff: So I think he's just question wants to know what exactly is this published view and maybe if you're going to show it in the DEMO maybe that's the answer.
Matthew Halliday: yeah I think that that that's best shown shown so i'll definitely touch that in the DEMO in a couple of minutes.
Claudia Imhoff: alrighty alright, well, we are actually out of time at this point, I do want to turn it over to you Matthew those of you.
Claudia Imhoff: In the audience stick around you're going to get a lot of the questions and a lot of the comments in the chat answered.
Claudia Imhoff: With the DEMO so with that i'll turn it over to you Matthew and then at the end we'll come back and say goodbye to everybody, but thank you, Paul don and Tony for your contributions today, it was really a good good session I really appreciate it all right Matthew off to you.
Matthew Halliday: Right, thank you very much, let me just share my screen.
Matthew Halliday: Hopefully, you can see that okay.
Matthew Halliday: All right, awesome so i'm going to jump in here, this is an encoder this is there's many different parts of the system i'll go over that real quick just kind of break down what are you looking at.
Matthew Halliday: But one thing I want to get very clear off the, off the.
Matthew Halliday: right out of the gate here is do not think during quarter as a visualization product do not think of in quarter as oh it's a another version of tableau or power bi while there is visualization and the same way as if you're using notebooks.
Matthew Halliday: there's some visualization capabilities, the way in which we want to engage with data does have visualization as being a helpful component to that.
Matthew Halliday: But the real magic value of in quarter is really on everything that comes before that.
Matthew Halliday: And so it's on the data side, the data acquisition, the date of publication, the data query that's really where they caught innovation lies and so.
Matthew Halliday: Now here i'm in my encoder instance doesn't tabs across the top that break down, you know things like security models, I can have users.
Matthew Halliday: This data sources, where I can connect to different data sources there's a myriad of different data sources available here that I can connect to we also have an SDK where you can add in additional ones and build them out, which has been done as well.
Matthew Halliday: In terms of schemas This is where the data resides once I connect to a data source, I want to bring in, and I want to have that representation of that data, so this.
Matthew Halliday: Is a list of schemas and i'll get into this in a little more detail in a moment, but this is going to in this particular case relate to Oracle EBS, so this is.
Matthew Halliday: replicating an oracle EBS installation into in quarter having parkey versions of that on my cloud data store and then being able to interact with it in a very different way than you would traditionally from from an analytics perspective.
Matthew Halliday: The questions we've been getting a lot about today is the business schema area.
Matthew Halliday: This is where I do not want to put my business users right in front of things like the actual physical data model that comes from EBS and you'll see why in a moment.
Matthew Halliday: But this is where you put something together in a way that they can discover understand and engage with.
Matthew Halliday: And then of course there's things like schedulers and obviously content which will jump into a second now what I want to start off, though, showing you.
Matthew Halliday: is something that's very dear to my own heart, and so I worked at Oracle and I worked in the Oracle EBS.
Matthew Halliday: Environment when I was there for quite a few number of years I was application architect, and I was part of the data modeling team, and we would create.
Matthew Halliday: These data structures, and this is actually what.
Matthew Halliday: The real each CRM would look like for Oracle so.
Matthew Halliday: These are all you know your Oracle tables and if you're familiar with these your your recognize them, they can each see parties are a customer transactions or that the real big heavy hitter table and some in terms of.
Matthew Halliday: The environment, you can see there's just a lot here, I can navigate through and have to zoom in to be able to see it because there's so much that's connected as part of this.
Matthew Halliday: Now this is the actual application data model, so this is how it stores, when you bring up an invoice This is where all of the things that you would see on the screen actually end up residing inside of these tables now.
Matthew Halliday: If I was to go and do analytics on this, you could see that this would be overwhelming, I do not want to put a business user and say here's your data go at it like there'll be like.
Matthew Halliday: Probably spending the next six months, just trying to figure out how to get an amount by a customer name right having to navigate through all of these different objects and they go across multiple schemas, of which there are many.
Matthew Halliday: And so, where the star schema came in, which was actually helpful.
Matthew Halliday: You know, was really it brought all of the columns together into subject areas, and so, for this i'm going to just jump over to.
Matthew Halliday: an oracle documentation here that just shows in the case of like General ledger.
Matthew Halliday: So in general ledger they create all of these different stars So these are all the different stars that are available.
Matthew Halliday: for you to just understand what's going on there now, this does not look like the data model, I mean when I first looked at this.
Matthew Halliday: I had no idea what it was, I was like I don't recognize any of these things like what are these like these are not common to me as the application developer.
Matthew Halliday: Who was on the other side, building the application, this was done by a different team.
Matthew Halliday: And so you're going to go through these, and you can see there's all these models and structures, and this is how you would be able to you know query them now, these are not connected, so if I want to actually look at this will refer to as a fact table.
Matthew Halliday: And then join it to another fact table that's not something that you, you do you look at these as siloed analytical subject areas.
Matthew Halliday: Now this data structure has to be repopulated as be copied built and what we refer to as a process of.
Matthew Halliday: d normalization which is taking that relational model and putting into something that looks like this now, the problem with that is obviously expensive takes a long time, exactly as we've been talking about today.
Matthew Halliday: Now, if I was to go and take this data set, but everyone starts with this data set that that star schema had to start with this data set it had to understand this, so, if you look at this goes this looks very complicated.
Matthew Halliday: that's what everyone has to start with it's just me give you a visual way to represented to see it.
Matthew Halliday: Now, in reality, though, if I want to you know engage with this data so let's say I want to look at some GL data I can click on my GL area, and I can see now this feels a lot.
Matthew Halliday: more manageable as a business user and now I go into GL I can see, I can see my natural account, I can see some journal details.
Matthew Halliday: I can see a whole bunch of transactions in here, you can see where they're actually coming from so they're all coming from different objects G headers G lines.
Matthew Halliday: And you know different sources G sources, so this is where those complex data model is curated in a way that a business user can navigate through this and say.
Matthew Halliday: yeah This is exactly what I would expect to see you know, a GL data on journal details that makes sense to me.
Matthew Halliday: And I could start exploring this data, so I could open this up inside of my environment, and I could start you know building out some some queries I can.
Matthew Halliday: drag and drop, and this is that bit with the visualization experience, but really i'm building up data tables and structures to be able to see exactly what's there and how to navigate through my data.
Matthew Halliday: that's great, and this is, you can see there's a lot of columns available to me here, but what happens if there was a change well if we actually go back to the underlying schema.
Matthew Halliday: And let's say we open up the the GL one here so let's open up GL.
Matthew Halliday: And and go ahead and explore the data, you can actually see that here, these are all of the columns these are a one to one mapping of everything that's available than tbs like there's a ton.
Matthew Halliday: When you go look at a star schema you wouldn't see every single column that was available, but in reality, I can use any one of these so.
Matthew Halliday: If I needed to add this attribute column into my business schema is literally just a step of.
Matthew Halliday: Not having to change anything, not have to start with a data pipeline or change or transform it's just coming in and saying Oh, let me go ahead and update the general ledger and let me go in and add you know.
Matthew Halliday: I can go ahead and Edit this and just drag and drop in that particular column that attribute seven column, and then it's available my business users can use it, they can obviously put in things like this is how you use it, I can give descriptions and they can help them understand.
Matthew Halliday: Now let's go ahead and look at some some data here so here i'm going to open up.
Matthew Halliday: A dashboard that we that we have here, here we go Matthew and i've got this GL summary I just took a copy of it, so I can edit this.
Matthew Halliday: But we have a number of these, and these are one of the things that we provided in quarter.
Matthew Halliday: is obviously this platform, but we also provide applications and a local applications and we focus heavily on things like Oracle EBS netsuite SAP.
Matthew Halliday: To be able to provide a very fast way for you to be able to deploy these applications, so you don't even have to start with understanding what's the relational model in Oracle.
Matthew Halliday: will give them to you with some pre packaged business schemas, but if you need to change them and add something to it, you can see it's very easy to adjust now.
Matthew Halliday: This is, you know that data that's coming in and being able to look at this at scale, you know with billions of records something that's you know pretty exciting.
Matthew Halliday: So if we were to look at this journal details and i'm just going to edit it up open this up and show you where this data is actually coming from.
Matthew Halliday: So it's got all these different grouping dimensions, but here i'm going to go look at what we refer to as a query plan and within this query plan.
Matthew Halliday: It really just shows you where all the data is coming from, and so these are your GL tables that are in Oracle.
Matthew Halliday: This is the base, even though we went through a business schema to get these you can see, these are where they were actually coming from.
Matthew Halliday: So this is the underlying model, so I can always go back and map it directly to what's going on.
Matthew Halliday: Now this is great if you're particularly like an EBS customer, because in Oracle EBS one of the nice things about the product.
Matthew Halliday: Is on any record, you can go ahead and open up the help of the about about that page and it will show you where that data is coming from.
Matthew Halliday: So a lot of time business users actually do know that some of that data is coming from these different objects so it's an easy way for them to tie in to have trust that the numbers i'm looking at is this actually accurate.
Matthew Halliday: So if I go out of this and actually look at the data, you can see here i'm seeing the the raw journal details so sit down to the journal detail line level.
Matthew Halliday: And so, in here this num these numbers and values have not been transformed and changed, unless I really wanted to.
Matthew Halliday: I can do that and there's an area in the product, where you can do formula based calculations to maybe put in business logic.
Matthew Halliday: But in here i'll be able to tie this directly back to what I see in Oracle so in this particular case, if I found there was a mistake, I could easily go back from here, and then update Oracle and then see it flow back into the system in a few minutes.
Matthew Halliday: So here, looking at this data, you can see, you can interact with it, I can drill into you know different batches.
Matthew Halliday: I can filter by that and then see exactly the details behind that particular batch.
Matthew Halliday: So it becomes very powerful, to be able to have all these things connected because they're not individual stars they're fully connected so that data is actually coming from the raw transactional level that we have so becomes very.
Matthew Halliday: interesting when you can think about how you can look at the data, think about the data and also navigate off of the standard things and say you know what I need to find something else I found an issue I want to dive in.
Matthew Halliday: and get some more detail around that, let me go ahead and look at the details behind it.
Matthew Halliday: If I go back here and look at you know some of the content we have.
Matthew Halliday: For this there's obviously different areas within the product, I can look at your receivables and I can look at Maybe my aging details.
Matthew Halliday: and go ahead and you know, look at these transactions find out exactly what's going on at the aggregate level, so this is where we think about how you want to create your data.
Matthew Halliday: To engage with it and that's you know that makes a lot of sense but, as we were talking about today, and as Tony mentioned.
Matthew Halliday: You do then want to think about it this way, but I want to be able to find out the details behind it so here at the bottom, you have.
Matthew Halliday: All of the details of the lowest green all the time, and all these numbers have been calculated from that so any change, I make any filter that I want to apply.
Matthew Halliday: will affect So if I wanted to say I just interested in net 30 payment terms of apply that filter this whole dashboard has not been changed.
Matthew Halliday: I did not have to do that and go ahead and set it up and figure out in my star schema models do I have that as a dimensional asset it's there because it's in the base tables.
Matthew Halliday: And because we're doing this on the fly at the time of query.
Matthew Halliday: You don't need to feel like you're tied in so it's very much a virtual experience of thinking in aggregates thinking in the way that you want to look at your data, but always having the freedom to transform change add.
Matthew Halliday: and be able to still have that confidence that what you're looking at makes sense, so you know.
Matthew Halliday: Dr driven try a drilling in here, I can filter by this account again, it will just read read update everything, so I can see.
Matthew Halliday: The details around that I can look at the top collector for this particular account.
Matthew Halliday: drill into that again all the detail keeps getting refined as I go, so I start with all this detail, but I can always see here the 40 rows that tie into this particular incident right.
Matthew Halliday: So being able to look at that level and understand and then you know remove any particular filter so I can just let's just look at.
Matthew Halliday: This collective Dupont across the board, and I can start just deleting all of those and then seeing all of this individuals accounts and what they're working on.
Matthew Halliday: So that freedom to be able to navigate through your data is really what I think this virtual kind of approach takes versus a physical infestation a manifestation of the structures that you think about.
Matthew Halliday: When now they're done at this point, I do have that freedom, as I mentioned to can navigate through and to experience it in multiple different ways.
Matthew Halliday: even be able to search across your data being able to say you know there's some transaction, let me look for you know something around here so maybe.
Matthew Halliday: I want to type in like you know computer so as I start typing you see no fines, though there's a bookmark that i've set up okay there's some.
Matthew Halliday: subtypes here and Oracle EBS and then I can say you know, keep typing and keep refining.
Matthew Halliday: Those answers for me, depending on the prompts i've set up, so I can you know drill into looking at maybe one of the dashboards that i've set up and look at those values.
Matthew Halliday: So becomes very, very engaging and reduces that kind of friction that you have with being able to get what you want from that data.
Matthew Halliday: So obviously there's more things I could dive into this, a lot of questions here I guess around things like what about the transformations you want to do so i'll just touch on that there is this ability and option to be able to go in so.
Matthew Halliday: let's let's look at the GL example we were looking at to look at an individual table, so the say GL balances.
Matthew Halliday: And here, maybe I want to create a formula column so here, these again is just a direct mapping of the table, so if I looked at this and previewed the data.
Matthew Halliday: This is actually what I would see if I went to Oracle and I said select star from GL balances, this is what I would actually see.
Matthew Halliday: All of these objects, with all of these details in there now if I wanted to say, you know what I don't want to engage with a piece of that data in that way, like.
Matthew Halliday: A flag, maybe, so I can look at this, you know, be a me that doesn't mean anything to me.
Matthew Halliday: I won't want to add a formula in here, so I could create a formula which would translate that a b&e into more meaningful values, so I can put my business rules and add them in very much like I would in excel.
Matthew Halliday: build out a formula here to to make that translation, so that the business users can use that.
Matthew Halliday: Once that's been done the kind of the nice thing here if I go look at my business schema and let's go back to this GL example, and I want to explore my data.
Matthew Halliday: Now i'm exploring it here, I can actually find out know where this data is actually coming from, so I can click on it and see.
Matthew Halliday: here's the source column here's where it came from so came from this business schema because the underlying source column.
Matthew Halliday: In cases where there's actually formulas in there as well, i'll be able to see those and engage with those and again create formulas again at this point as well, to be able to see the data that the way I want to see it.
Matthew Halliday: So when you think about that freedom compared to the approach here, which we kind of see as being the traditional approach.
Matthew Halliday: This really does not give you that flexibility and anytime I want to make a change i've got to change the data pipelines, the flows versus just a literal.
Matthew Halliday: metadata change and so these physical schemas in encoder but those business schemas are all metadata driven it's just Just what do you want to see what should be in here, and as long as those relationships are known you're able to build on top of it so i'll come back to you, Claudia.
Claudia Imhoff: All right, thank you very much, and as a wrap up, first of all, thanks to all of you, Paul Donna Tony and Matthew for your time today, this was an excellent excellent.
Claudia Imhoff: panel discussion and a good DEMO and thanks to you in the audience as well.
Claudia Imhoff: For hanging in there with us, I do want to before we say goodbye I do want to mention that this is the first of two panelists the second one is coming up fairly soon April 20th.
Claudia Imhoff: And it will be from a practitioner standpoint of how do we implement these virtual stars, how do we make sure that they're doing what they should be doing a lot of your questions had to do with that, so I hope you'll stick around.
Claudia Imhoff: And and join us on April 20 with that i'm going to let's see I think there's one more slide that we wanted to show.
Claudia Imhoff: As a I guess a gift Matthew you might want to describe what it is.
Claudia Imhoff: But I believe it's a it's a download that somebody I be able to.
Matthew Halliday: Do yeah so we actually know the download even easier that's too hot in this day and age have to download and install software, but rather in literally.
Matthew Halliday: The average user takes 90 seconds, so you go test yourself start the stopwatch and see how quickly you can do this.
Matthew Halliday: But there is a free in quarter cloud trial, where you can go to encoder so you can just go to encoding calm and the top right, you will see.
Matthew Halliday: get started, free, you can click on that, and then it will just ask you for your email.
Matthew Halliday: Put in your email address it will send you a link you click on that link and you'll activate account and right away you'll be dropped into the quarter environment.
Matthew Halliday: And within then there's some there's some guided navigation tours that will help you connect look at data and be able to kind of go through and see that so.
Matthew Halliday: Just the details, there you can you know sign up again, you can find it also add encoding calm, if you cannot remember that URL at the bottom.
Matthew Halliday: But that that will give you at least 30 days to be able to look at in quarter to experience it to dive in look at a little bit more of the features that are there.
Matthew Halliday: And there's also some great things in terms of if you do want some help along the way, definitely reach out there's a.
Matthew Halliday: chat that is there that will speak with one of our agents technical agents that will actually lead you through the process and help you, and there is also, we do have.
Matthew Halliday: training as well, so we do have on demand training classes, where you go should take an exam and get certified, if you so wish.
Matthew Halliday: So you can go through that process and become familiar with the with the application as well, so i'd love to offer that and have people.
Matthew Halliday: Experience for yourself because honestly, a lot of what we've talked about today.
Matthew Halliday: A lot of people say it sounds too good to be true, or it sounds like yes we've heard this before and that's probably been one of the biggest challenges in this industry is so many claims have been made that have yet to deliver on that promise.
Matthew Halliday: But what we found with a quarter when people actually try it for themselves.
Matthew Halliday: and see it.
Matthew Halliday: On their data and the DEMO never does it really justice because you could say, well, you probably did something behind the scenes to make it work to make it fast and there's probably some limitation that I don't know about.
Matthew Halliday: Well, try it for yourself, because if this is true, and this works which obviously I believe it does this is a game changer for how you can think about your business application data.
Claudia Imhoff: Alright, so with that I guess we're going to say goodbye to everybody and again don't forget next panel is April 20 do login will get the URL up and start publicizing it very, very soon.
Claudia Imhoff: Thanks everybody again, thank you to my panelists you guys rock it was just wonderful and with that we'll See you in a couple of months, then bye bye everybody.
Matthew Halliday: Thank you.
Hosted by:
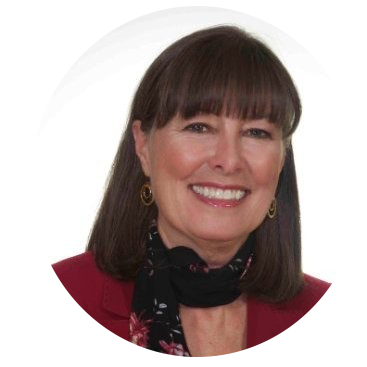
Claudia Imhoff
Founder
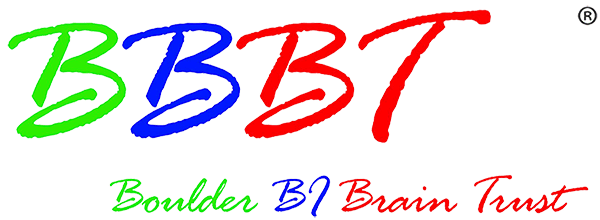
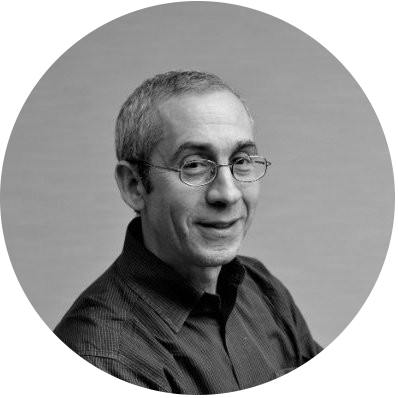
Tony Baer
Principal
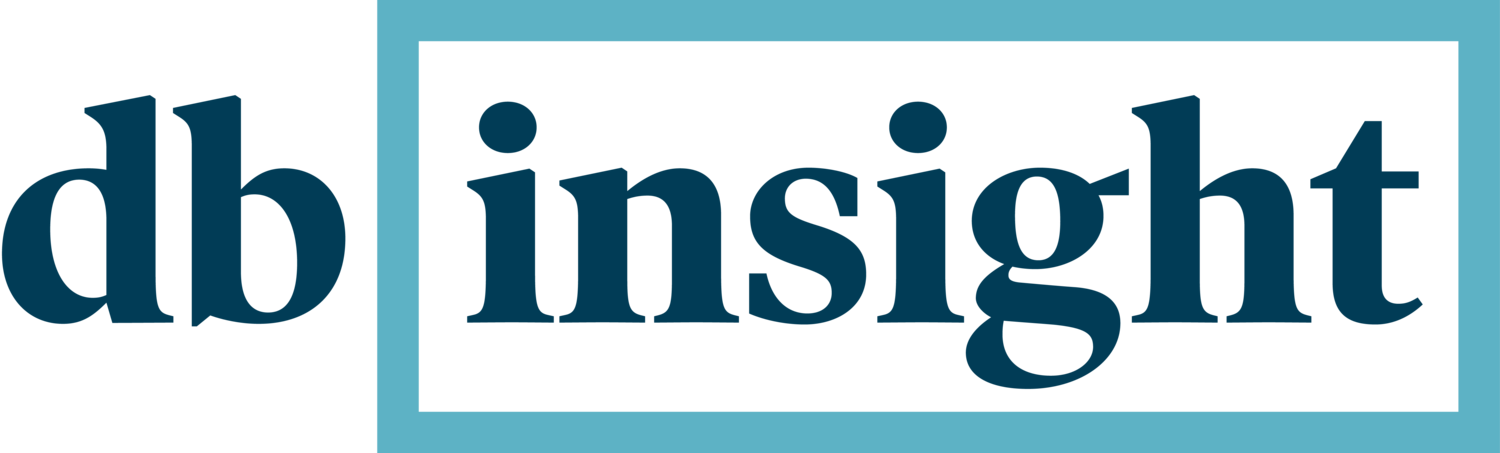
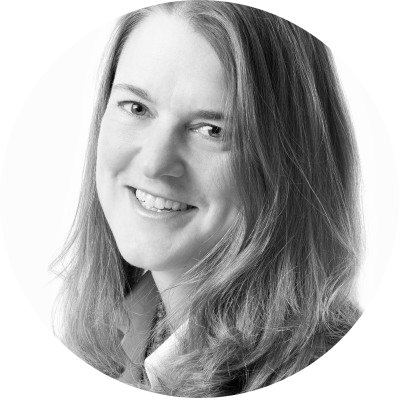
Donna Burbank
Managing Director
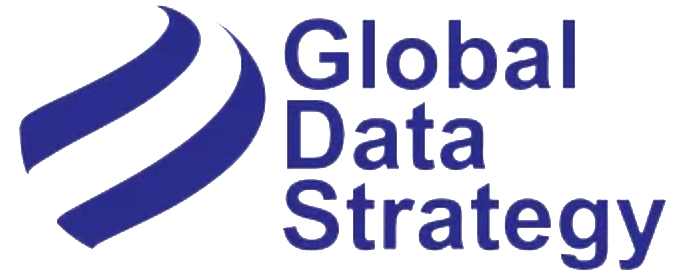
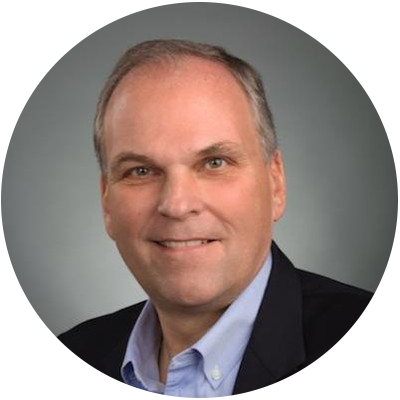
Paul Kautza
Managing Partner
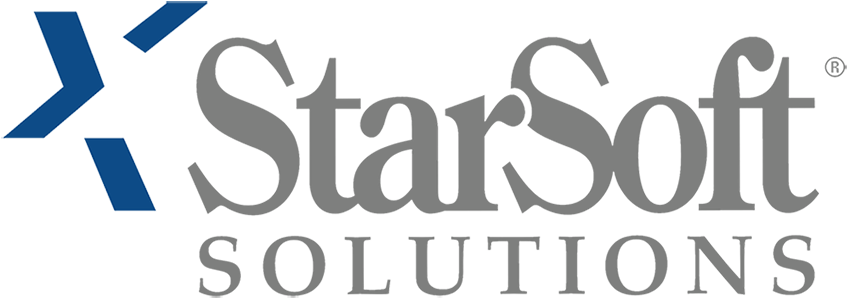
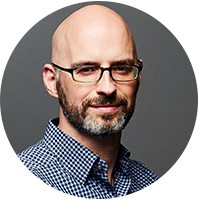
Matthew Halliday
Co-Founder and EVP of Product
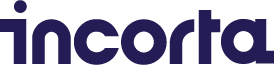