The Last Mile to Data Mesh: Enabling Real-Time Operational Analytics
Could the "Data Mesh" finally solve age-old challenges in the analytics world? The promise of domain-specific insights with baked-in governance sounds fantastic. Organizations can spend valuable data engineering cycles on the consumption side, instead of always working on fragile data pipelines. The key is an implementation architecture that embraces several core principles.
Watch this expert panel to hear former Gartner Analyst Sanjeev Mohan, now Principal of SanjMo, as he outlines the keys to success with this new approach. He'll be joined by Incorta Co-Founder and EVP of Product Matthew Halliday, who will demonstrate how Incorta can be used as the last mile to a viable Data Mesh, regardless of where and how enterprise data is stored. This approach enables operational analytics at the speed of any business.
Watch this expert panel to learn:
-
How an analytics engine helps ensure quality governance
-
Why a data mesh makes sense for improving domain-specific insights
-
How some of the world’s leading brands have transformed their operational reporting
Transcript:
Eric Kavanagh: Ladies and gentlemen, hello, and welcome to this special web seminar within quarter, the direct data platform.
Eric Kavanagh: The topic today expert panel, the last mile to data mash enabling real time operational analytics.
Eric Kavanagh: Just a quick look at our speakers today my good newbie FF sanjeev mohan founder and analyst at San Jo formerly of gardener is a seasoned data enthusiast.
Eric Kavanagh: He loves talking to customers he loves diving into the weeds he loves being theoretical, but also practical so we're gonna have a fun time talking to him Matthew holiday.
Eric Kavanagh: Co founder and VP of product at encarta i've been tracking these guys almost since their inception.
Eric Kavanagh: And they came out like a rocket ship and it been going ever since he has more than 15 years experience.
Eric Kavanagh: At Oracle, of course, a lot of folks in our industry spend some time at Oracle kind of like the six degrees of separation almost everyone either worked at Oracle.
Eric Kavanagh: Or has worked with someone who worked at Oracle and then, of course, yours truly host of the am radio I just gave a teaser we're getting the am radio into New York Boston and San Francisco soon so watch for that and listen for it.
Eric Kavanagh: Especially those markets so quick agenda i'll just talk for a couple of minutes, then i'll hand it off to sanjeev for the missing link.
Eric Kavanagh: For data mesh and then Matthew holiday is going to talk for a few minutes to some slides and also do a DEMO and explain how they.
Eric Kavanagh: can fill in that gap of the last mile to data match, we do have a wrap up and audience q&a don't be shy send your questions at any time we'll pick those up at the end of the hour.
Eric Kavanagh: And let's talk about ideal data structures, so this concept popped into my head last night, as I was pondering what to possibly say with these.
Eric Kavanagh: Remarkably, smart people in the room here and I remember learning years and years ago about how ancient philosophers like euclid like Euclidean geometry.
Eric Kavanagh: Were fascinated by the honeycomb the structure itself and viewed it as the most efficient structure for storage for durability.
Eric Kavanagh: For endurance for all sorts of different characteristics and I think this creates a really interesting metaphor.
Eric Kavanagh: To data right, because we always talked about the database for years and years Oracle of course database IBM.
Eric Kavanagh: With db to you've got Microsoft came into the data space database space years and years ago well now you've got tons of databases Open Source databases column or databases graph databases.
Eric Kavanagh: All sorts of different data bases, but then this concept of data fabric came out data fabric is not just the data base.
Eric Kavanagh: it's much richer than that, or at least it should be an all too recently this concept came out of data mash so I dove in trying to understand what do we mean by this.
Eric Kavanagh: it's really interesting stuff we had a great show a couple weeks ago, and in fact sanjeev intended.
Eric Kavanagh: attended and made some interesting comments about but we'll get into some detail about what that means, but I just want to put the remind us.
Eric Kavanagh: While this matters and where we came from how we got here you think about it, data warehousing deep many of the data warehouses there.
Eric Kavanagh: extent that are running today enterprise data warehouses at large corporations, they were designed around a set of constraints that no longer exist.
Eric Kavanagh: Because back in the day, we had to worry about.
Eric Kavanagh: The fact that storage was expensive, we had to worry about the fact that the pipes were pretty thin the processors were not very fast, and you know NPP massive parallel processing that's not new technology.
Eric Kavanagh: But in the last five years or so wow has that been optimized it is reached a level of maturity that we have not yet seen in this industry, and that is changing just every gosh darn thing about what we do.
Eric Kavanagh: And so, when you remember that we have these constraints that drove the design of our architecture or information architecture.
Eric Kavanagh: 30 odd years ago, let go of those constraints and understand that there are new ways of doing things.
Eric Kavanagh: And I view this as a very elegant design a really elegant metaphor, because think about it, even when we talk about NPP a lot of times we talk about what.
Eric Kavanagh: Worker bees, we use the metaphor of bees to go in this little massive parallel processors and all those little pockets are little notes right, so there are lots of interesting.
Eric Kavanagh: analogies to this, and I would ask all of us to kind of open our minds to what is possible in this new world.
Eric Kavanagh: that's probably one of the hardest things in an organization is shedding the old paradigm of how things.
Eric Kavanagh: used to be because of what those constraints were because a lot of those constraints like I said, are gone so this design, if you do a data mash.
Eric Kavanagh: On top of a data fabric, if you do it well it's going to be scalable it's reusable I love that one with the honeycomb it's elegant it's modular it's durable it has basically everything we want so with that i'm going to stop sharing and hand it off to sanjeev mohan take it away.
Sanjeev Mohan: Thank you Eric I am going to share my screen here, so I can.
Sanjeev Mohan: Show.
Sanjeev Mohan: Alright, so thank you for giving me this opportunity, my favorite topic I live and breathe data, this is going to be very exciting so let's start with what is it that the data, consumers are asking for every day when I talked to them.
Sanjeev Mohan: And for the last many years so data consumers want self service they want this flexibility, so they should have the ability to add new data sources so they can perform new use cases on on on data they also, of course, want this whole.
Sanjeev Mohan: process to be highly scalable and and they won't do it in with the least amount of friction, so no called local development has become a very.
Sanjeev Mohan: Important piece, finally, the businesses or the business analyst do not want to go to the.
Sanjeev Mohan: Sources directly to the underlying maybe files on object stores, they want to be able to create the analytics using some sort of a semantic layer, in other words, their business terms.
Sanjeev Mohan: of which are mapped to this underlying physical architecture, the physical model some other things that they're asking for is speed to incite this whole concept of legs etfs stuff from.
Sanjeev Mohan: source to target or let's do a Nike batch job, and then we have a complex modeling that slows things down is not.
Sanjeev Mohan: good enough in modern times so people want very quick current data be available to them once the data is available, and they start writing queries they obviously want low latency on the queries they want.
Sanjeev Mohan: To be able to run a higher concurrency so many data consumers want to run.
Sanjeev Mohan: The process and and from an IT perspective organizations are saying that, why are we spending time in doing operational infrastructure overhead we should be focused on business logic so so as a service.
Sanjeev Mohan: Deployment are really important now, why are we doing all of this, because at the end of the day, organizations are most concerned about.
Sanjeev Mohan: Doing analytics paying for it, but making sure that they're getting good value for the investment that they are making in the architecture so lowering the total cost of ownership.
Sanjeev Mohan: is important and equally important is cost predictability this whole notion, many years ago, when I first started in.
Sanjeev Mohan: My career in in databases, we used to frequently joke about somebody doing Cartesian joins on two tables with a million rows.
Sanjeev Mohan: Now we have billions of rows in a table, so we in the cloud it's very easy to run something and wake up next morning the CFO screaming at you, because you have a bell that the cloud provider just sent to you for this kind of.
Sanjeev Mohan: query that ran so there's lowering the keeping the cost predictable is also important, so this so so let's now change gears and look at what is the architecture that we are most commonly.
Sanjeev Mohan: Working with this is a busy slide, so this is this has so much information on it, we could spend pretty much all day talking about it, which we don't have so i'll go through this.
Sanjeev Mohan: Fast I apologize for some oversimplification here but.
Sanjeev Mohan: This is an analytical architecture on the far left hand side is I ingestion architecture, we are getting data from a number of operational transactional data sources such as er be CRM.
Sanjeev Mohan: point of sale, we are also getting a lot of beta in terms of files these files could be images videos audios, but it can also be log data lot of.
Sanjeev Mohan: Different types of logs get generated, we also getting a lot of streaming data this streaming data is coming on things like Kafka iot is really big these days.
Sanjeev Mohan: Finally, applications these could be SAS applications like salesforce where we make an API call and we get that data, a lot of this data is coming from on premises and it is coming from.
Sanjeev Mohan: from multiple different cloud providers, we take this data, what I don't show here is physical infrastructure layer and we landed into some staging area into our data lake or some storage location.
Sanjeev Mohan: Then comes the data engineering space, this is, this is where there's a reason why i'm walking through this architecture so.
Sanjeev Mohan: So, the reason is because now we're going to these complex transformations we have data from multiple different sources that data needs to be enriched.
Sanjeev Mohan: We used to have a very homogenous architecture in the past, now we have a very heterogeneous, so we there's a big need to to reduce the time it takes to.
Sanjeev Mohan: To do this transformation so real time processing has become really important we we've talked about.
Sanjeev Mohan: Low code no code devops has risen to be an extremely important component that was sort of the missing link for data engineering and then tools like DVD have have.
Sanjeev Mohan: really done a lot in in helping us automate it then there's a bit of the ability piece data, also the ability.
Sanjeev Mohan: Is understanding the state of your data, all the way from source there it's consumed, it is monitoring the data but it's not just data looking at data, how it behaves.
Sanjeev Mohan: And what anomalies happen in data so basically we were expecting X amount of data and and fewer elements showed up then.
Sanjeev Mohan: alerting us, but it is more than just a data it's also understanding the entire pipeline and understanding, where the pipeline breaks, why it broke and very quickly doing root cause analysis.
Sanjeev Mohan: And finally, it is also the interplay with infrastructure how's how's our data pipeline, using the resources, how efficiently.
Sanjeev Mohan: And then there's a feedback loop where reverse it to.
Sanjeev Mohan: My focus today is on the five right hand side on the far right hand side is a reason why we are starting to look at.
Sanjeev Mohan: Many of these new analytical offerings because the first thing is that now we have several more data consumers that we used to have these data, consumers are asking for self service access and all the other things we've talked about.
Sanjeev Mohan: But there's a big need now, because we are in a heterogeneous environment and your many data sources many transformations happening, so we have to have an ability to discover the data in the business terms.
Sanjeev Mohan: And govern have a govern access but governance i'm including a number of pieces here it's it's a bucket of it includes data quality.
Sanjeev Mohan: It includes data authorization, maybe even master data management, so there are a number of authorizations and approvals that needs to happen in a consistent manner.
Sanjeev Mohan: We also have more use cases as we've talked about streaming has become a big use case it used to be some years ago, a major use case for data warehouses will reports and dashboards so we would build.
Sanjeev Mohan: Do a Nike batch job and then next morning, we will have our dashboard, but that is not.
Sanjeev Mohan: not considered to be competitive enough for organizations to have stale data data science machine learning is actually one of the biggest drivers as to why we're seeing so much innovation happening in this entire pipeline.
Sanjeev Mohan: Now what's fascinating is how the data is finally made consumable how the data is delivered and there's right now, a major sort of.
Sanjeev Mohan: discussion going on in the industry between cloud data warehouses and lake houses cloud data warehouses originally came with a well defined structure.
Sanjeev Mohan: But they were for structured data now cloud data warehouses can also support unstructured data lake houses started on the other side from unstructured data, but they have now started adding new enhancements into the data lake which.
Sanjeev Mohan: Things like we used to have a patchy hive now we've added things like hoody iceberg delta that provide acid semantic schema evolution time travel.
Sanjeev Mohan: So we're reaching a point where the cloud data warehousing and the lake house is starting to merge.
Sanjeev Mohan: But then we have another category, which is called the analytics engine which is basically taking the data as it is, but building some query accelerators and being able to run queries very quickly without having to do a lot of transformations.
Sanjeev Mohan: i'm a big fan of Simon cynic Simon cynics as i'm sure by now almost all of you know he's a guy who made, starting with why as his calling card.
Sanjeev Mohan: So this is a wise slide, why do we need to rethink our analytics architecture, but something that says it start with Why then go to how are you going to do things and then say Oh, by the way, this is what.
Sanjeev Mohan: technologies are what is available so let's move from why to how.
Sanjeev Mohan: So this is an interesting slide this slide shows that, how are we helping our data consumers with reducing the friction between the data protection and data consumption.
Sanjeev Mohan: In the previous slide the even though that is the sort of the de facto way of how we are doing things.
Sanjeev Mohan: But we have a problem, because the data producers and data consumers separated to this data engineering task.
Sanjeev Mohan: And so, somebody is having to understand the context of the data in the source and somebody is trying to understand what does the consumer want and merge these two together.
Sanjeev Mohan: Not only does it add time it also adds latency to how quickly we can make that data available opens up all kinds of problems with data getting duplicated.
Sanjeev Mohan: replicated quality issues governance issues data structures constantly changing we're taking data from an oracle source structured.
Sanjeev Mohan: converting into files using Apache spark converting it into more files and then loading into structured data into snowflake.
Sanjeev Mohan: You know, so this, this is, this is a the issue which some of these these concepts have tried to solve data hubs came out almost 20 years ago I remember mark logic used to do this, where they would only ingest Meta data and be able to to build out a consolidated comprehensive.
Sanjeev Mohan: Data virtualization has recently come of age, because data virtualization allows us to leave data, where it is.
Sanjeev Mohan: it's affected by the speed of light, but with data virtualization is now doing a lot of intelligent things like caching indexing pushing down the queries.
Sanjeev Mohan: Intelligent query optimizer and in fact data virtualization is a technology that is quite heavily used by data fabrics data fabrics have been around also for many years, many organizations many vendors have better fabrics lately Gardner has defined data fabric as as a way to implement.
Sanjeev Mohan: A consolidated analytics architecture What it does is it takes data virtualization but it puts a knowledge graph on top so it's an implementation technique.
Sanjeev Mohan: Data sharing has been all the rage, in the last year or two so just last week we had an ETA on data management vendor that launched their cloud data marketplace.
Sanjeev Mohan: We have data bricks launch and before that all the hyper scale cloud providers have it snowflake started the trend, again, the idea is the same do not move your data to create copies leave the data, where it is but provide a government way to access that data.
Sanjeev Mohan: The latest concept that has come into the space is data mesh data mesh was introduced by Mr Danny in 2018 and she works for thought works.
Sanjeev Mohan: So this is how we are starting to think about our delivery aspects of analytics so now that we look at how let's look at the what what is data mesh data mesh.
Sanjeev Mohan: has four principles, these are, by the way, great principles.
Sanjeev Mohan: they're not new they've been around for quite some time, what is new is the way they've been packaged together and it's called a data mesh so the very first principle is domain ownership.
Sanjeev Mohan: Like I said, not a new concept we've had domain driven design for applications for a long time now we are applying it to data, and why not.
Sanjeev Mohan: How long have you been trying to fix data quality problems and we haven't been successful, the idea of domain ownership is to give more accountability.
Sanjeev Mohan: To the business domains, because they understand the business context, the most.
Sanjeev Mohan: So the idea is to do a shift left instead of trying to do modeling of our data curation of data on the consumer side we move it into the data producer side so data engineers.
Sanjeev Mohan: More more than ever, they shift into the domains and and what it do is not only do the curator and take accountability to to handle this data and make it available for consumption but.
Sanjeev Mohan: The package, it as a product, so that is a second principle data as a product they said data.
Sanjeev Mohan: That the domain, the business people have produced, and it turns it into a product that consists of data, its metadata maybe api's some early.
Sanjeev Mohan: implementations of data mesh has started to to also provide a notebook even some code samples some documentation on usage so it's a it's a product.
Sanjeev Mohan: Where where the consumers know how to consume that data so so basically what you're hearing is that data mash is is a concept, and it is heavily focused on the organizational aspects, in fact, one of the key.
Sanjeev Mohan: roles for data mesh is a data product manager, whose job is to to make this data available to the consumers.
Sanjeev Mohan: That next principle is that there is a self service data infrastructure we want to make sure that there are some common data infrastructure.
Sanjeev Mohan: To which these domains are adhering to, so there are some common guidelines.
Sanjeev Mohan: Now comes the most complicated piece of data mesh which is called federated computational governance.
Sanjeev Mohan: Why is this complicated is because data does not just reside in one domain data needs to be shared across different domains, so we need some sort of a consistent.
Sanjeev Mohan: Application of policies, whether that policy is how do I access it How long do I retain it, how do I do data residency so we have a whole governance piece that needs to be needs to handle common into operable data.
Sanjeev Mohan: So this is what a data mesh is that takes me to the last slide and I will address what it's not not just what it is so we've already seen what it is, it is a primarily a concept which addresses the people and process consultants.
Sanjeev Mohan: Who should use data mesh, generally speaking, it should be large organizations that have many domains, they have data quality issues they have many data silos.
Sanjeev Mohan: And they're trying to evaluate how best do I meet my data consumer needs so let's look at the right hand side, what is it not.
Sanjeev Mohan: It is not an architecture and it is technology agnostic, this is one of the big reasons why data mesh has become little bit of a.
Sanjeev Mohan: Of a talking point to be very politically correct and the reason is is because there is no reference architecture and no technology guidance, it gives different products vendors.
Sanjeev Mohan: The leeway to basically say this is this is, this is my interpretation of a data mash and technology, people like yours truly likes to be able to see things in a tangible format and that tangible format is still being developed.
Sanjeev Mohan: One of the companies that playing that is also in the space is in quarter i'm very interested to see how is in quarter supporting this concept of data mesh with this, I will stop here and I will hand it over to my good friend Matthew holiday Thank you Matthew.
Eric Kavanagh: Okay, maybe stop sharing.
Eric Kavanagh: There you go Matthew take it away and folks I do have a technical expert for any detailed questions you have don't be shy.
Matthew Halliday: All right, awesome thanks sanjeev far great hearing your insights and thoughts, this morning, so.
Matthew Halliday: One of the things I want to talk about today is really getting to where where is the big problem where is the big bottleneck when we think about.
Matthew Halliday: analytics and the questions that we're trying to answer as we go and really when you think about it.
Matthew Halliday: Questions are really how business users engage with data that's how we learn we asked questions we do this from a very young age, we asked questions if you any of you have children, you know they can ask a lot of questions and so it's really important to be able to.
Matthew Halliday: To kind of slide just jumped on me here one second okay.
Matthew Halliday: To understand where does the question gets asked and at what point in the technology can be answered and so.
Matthew Halliday: The old way is you know you go through this very protracted process of you know, engaging with it weeks worth of data to train, you know, working with the data to try and figure it out, transforming it put it into a shape that you can run a query against that.
Matthew Halliday: And then hopefully in a few short weeks, you might get an answer to your question.
Matthew Halliday: And really that doesn't cut it right it doesn't change an organization to be data driven if you're engaging with the data and you're asking questions and you get answers on a weekly routine or a weekly kind of cadence.
Matthew Halliday: That really doesn't in you know, encourage you to ask more questions, it makes you really think at the very beginning, is this question worth asking.
Matthew Halliday: Like Do I really need to know the answer to this question, which obviously gets in the way of being able to be agile, to be able to really understand what is going on, when you look at the data.
Matthew Halliday: The new way right is, how can we get the question to be closer to the source data and not to have all the data transformation be between the question and the answer, so the closer you can ask the question on the data as it is, the better, you can you can get the results.
Matthew Halliday: So encoder is a unified analytics platform that enables us to be able to bring in lots of different data sources lots of different data sets from different places.
Matthew Halliday: and to be able to bring them in whether it's you know big data stuff like Kafka.
Matthew Halliday: patchy drill etc, or whether you're going off of your some traditional aws and databases or, more importantly, maybe even your applications, and this is the bit that gets really interesting.
Matthew Halliday: Because the applications obviously you have probably many of them within your organization there isn't just one application that has everything that you need.
Matthew Halliday: You probably have a large number of these applications that really.
Matthew Halliday: paint the picture of what's going on your business, if you could bring all of the data together in a meaningful way, and so the equipment platform enables you to do that it's a one, two and three and experience.
Matthew Halliday: That handles a lot of things that we've been talking about today, actually, how do you get data acquired into a platform that can be centrally used in a format that's not proprietary if you're looking at applications today like salesforce or zora Oracle SAP EBS from Oracle.
Matthew Halliday: For example, getting that data and actually having it in a form that's not in the proprietary format is actually pretty difficult.
Matthew Halliday: Getting it in a way that can be consumed by other products is also very difficult.
Matthew Halliday: So it becomes very challenging for organizations to be able to get that data so that they don't have to replicated all over the place, you can have it in a cloud storage location where you can have you know park a as a read only.
Matthew Halliday: As a format that you can read from and not just having as a read only kind of append structure, but even getting party which is traditionally that append every time a transaction comes in, you added.
Matthew Halliday: How do you handle things like updates which happened in your source applications, you know.
Matthew Halliday: paki became very popular because it was great when you have things like sensor data or any kind of data that was just additive the event happened let's track it really good for events based.
Matthew Halliday: But when it comes to being able to handle things like updates and inserts and deletes becomes a little more challenging.
Matthew Halliday: And so, how do you handle that when you put it provides that ability to say I can have, without having to do a line of code.
Matthew Halliday: To be able to have that experience, where I can have updated parquet that represents it that can be used by other products as well that can be used.
Matthew Halliday: To opposable components that we can use with other systems without it being completely just locked into one vendor.
Matthew Halliday: Obviously we've talked a lot about just the process in terms of NPP in terms of you know, things like Apache spark which is you know, obviously, an amazing in memory platform it's great for being able to run code it's very for being able to do a lot of things.
Matthew Halliday: That is a component of thing called a platform we actually don't use that as our core query engine.
Matthew Halliday: But we use that because there are business transformations that you would want to do there's business logic that you want to do and we're not talking about transforming the data into shape that makes it more.
Matthew Halliday: easily queried by the query engine in quarter the query engine to actually run against the same format of your data as it is in the source system, so if I have 200 tables that make up my booking system.
Matthew Halliday: i'd have 200 tables inside of the quarter and then I would run against those in a live fashion running against those tables that are inserting quarter in the platform.
Matthew Halliday: In the data lake with the park a file for map and then be able to see and interact with that data without having to make all these limiting questions about what's the data that's important to me.
Matthew Halliday: So the analytics piece is obviously super important being able to do that and that's really the magic of encoded has really transformed compared to any other query engine on the planet, quite honestly.
Matthew Halliday: We focused on that one problem your data is not flat your data and your applications is not flat.
Matthew Halliday: Everyone else is missing, you need to bring us flat data we're like well what if we can fix that that's not interact with flat data.
Matthew Halliday: let's give you the data in a way that you can react and act upon it, because that gives you the agility and flexibility that we've been talking about that enables you do not have.
Matthew Halliday: A column just adding a column taking weeks and months to an existing application or a data product but rather being able to.
Matthew Halliday: Just do that in a few short seconds and then get the results, and we have customers have 80,000 columns in their platform.
Matthew Halliday: And at any given time on any given day they might use 3000 of those.
Matthew Halliday: But the fact that they didn't have to be asked up front, they weren't asked what are the columns that are important to you what's the data what other questions you can ask they won't ask that question.
Matthew Halliday: The platform is obviously flexible enough to be able to handle that so it's those kind of conversation of having raw data fabric and mash.
Matthew Halliday: That enable you to bring the data to life so i'm going to jump in and actually show you now the quarter platform, and so we can see it kind of.
Matthew Halliday: Running here, so let me just share the screen just gotta move maybe some of these videos around yeah okay cool um so here i'm in the platform, you can see there's a number of.
Matthew Halliday: components and pieces to this product i'm going to we have a we have this.
Matthew Halliday: Security obviously one of the things in quarter does very well is ensure that you can share the data and you can actually share dashboards with.
Matthew Halliday: Thousands of people, but every single dashboard they look at might be, it might be different for them it'd be personalized to them, it could be that the data behind it is actually secured differently.
Matthew Halliday: So, even though it's built by say your original salesperson and you want to look at your dashboard it'd be one dashboard for every single sales person, they can look at it.
Matthew Halliday: And it would filter down to even their individual transactions so for the first time, you can actually match.
Matthew Halliday: The analytics to have security that's the same as the source application so what I see in salesforce is what I see in my analytics I don't see more of it.
Matthew Halliday: And the reason for this is because we are bringing that data in the shape shape.
Matthew Halliday: we're not transforming it and changing it we're not aggregating it and the moment you start to aggregate data, then you lose that capability to do it because the data is not is not.
Matthew Halliday: Generally, is not secured at an aggregate level it's secured at the transactional level it's like these are my transactions, who is the owner of these opportunities.
Matthew Halliday: Well, if you're that rapids you right you're going to have that so that's that brain of record you want to see all of your transactions.
Matthew Halliday: And so, in court, it enables you to do that and, of course, built share components of the system.
Matthew Halliday: What i'm going to show here today, though, first of all, is a couple of areas, but I kind of start off with just taking you through this process just saying.
Matthew Halliday: let's say we have a source system, and we want to get data into the platform, so this is this low code no code approach, there are ways, you can do coding, if you so wish, and I can show that in a moment, but.
Matthew Halliday: In this case, you know i've got lots of different connectors that I can connect to here and bring in data from these.
Matthew Halliday: So these are all available very easy just you know click on them, provide the detail and then be able to kind of connect to that source can kind of go through that and do that now, in this particular use case i'm going to use an existing.
Matthew Halliday: connector that I have, so let me go back here i'm going to pick a mysql database.
Matthew Halliday: And here we just got some some tables so there's a number of different tables here i'm just going to select all my tables.
Matthew Halliday: This would be exactly the same as if I was connecting to salesforce at this point, whenever that connector is done this is how the data will look it will come in and i'll see it in this table structures.
Matthew Halliday: I can see, you know columns I can give it names data types, I can make any changes I need I can create custom my sequel So if I want to actually change the sequel behind the scenes here.
Matthew Halliday: I can go ahead and do that, you can see, is a really straightforward, you know select statement from that data set and then I can go ahead and proceed with these tables so just got to give this a name.
Matthew Halliday: And just do that, and then you can see here i've got my 32 tables is identified joins between those tables, we got 273 columns now.
Matthew Halliday: This isn't a huge data set right but it's still illustrates this point, and this is the same process, whether we're doing this against SAP or Oracle E business suite which has.
Matthew Halliday: Over 50,000 tables it's exactly the same process.
Matthew Halliday: So final step here is, I want to actually load this data, I want to bring it in so it's created my schema for me, you can see here it's now we're doing the extraction it's already started bringing in the data from the stats or system.
Matthew Halliday: And then i've got these few other sections I, haven't done any enrichment, to make data, but that is a section that is there.
Matthew Halliday: That I can actually go and do things I can create machine learning programs, I can create pay spark scala and do.
Matthew Halliday: Anything that I want to do that data enhance that data creep formula Collins with complex rules, and it could be based upon maybe.
Matthew Halliday: You know how much money I should give in terms of if i've got a product, and you know i'm licensing it and I o
Matthew Halliday: licensed revenue to that to that vendor I can calculate that so that, when my users use it, they don't know take them the gross revenue and actually get the net revenue that they should be looking at.
Matthew Halliday: So this data is coming in Syria about 25 million rows or so that we've brought in that's generated paki paki for me now on my cloud storage.
Matthew Halliday: So that's that's all good to go that could be used by other systems it's it's available it's that's great what we've also created though here is the direct data mapping.
Matthew Halliday: This bit is you can think of it as a metadata layer that enables us to be able to do those queries against data that has not been flattened and will see this in a second.
Matthew Halliday: and be able to to experience it in a way, as if it had been flattened so i'm getting the performance of flattened data without flattening.
Matthew Halliday: Now, why is that important well there's two reasons, one when you flatten data it takes a long time, you have to take it from a lot of cases 5060 tables to get a flattened view that you're going to use.
Matthew Halliday: You might put dimensional values around it, but similar similar use case the other problem with it is when you flat data, you have to think of what level do I want to flatten the data.
Matthew Halliday: And you have obviously you have many options for this if I was going to flatten orders, for example.
Matthew Halliday: Do I flatten the order information at the header tape level so and so placed an order, this was the total order amount.
Matthew Halliday: Or do I care about something a little more granular like what would the items that they ordered so that i'd have to go down to the line level and look at what were the products behind that order.
Matthew Halliday: Well, I could even go lower, though, if I want to know about what what was the distribution what were the tax lines.
Matthew Halliday: What was you know a lot of things can kind of come into that shipping information.
Matthew Halliday: Am I going to create flattening at every single level, because my business users might want to do analysis at different levels.
Matthew Halliday: So how do you understand that so that's when flattening if you're doing it historical way is actually very complex and takes a lot of time and it slows down your data and just too, because then you have to reflect and all of this stuff all of the time.
Matthew Halliday: So my data is available, I could actually start building on this right now.
Matthew Halliday: So just to kind of show you this, this is the way that we can build and this could be in quarter, but this could be tablo this could be another visualization tool against in quarter platform.
Matthew Halliday: The the real magic here is the fact that I didn't have to do the flattening and so it's the same experience, if I was to grab some of these columns from in in.
Matthew Halliday: In in tableau it'd be exactly the same right, so I can throw in my measure, for example, and i'll start to get some real results coming back.
Matthew Halliday: I can sell when I look at your product category so i'll just bring in you know category name here, I can throw that across.
Matthew Halliday: Very quickly, you know i'm gonna have my product category against the amount i'm saying I want this to be you know, an aggregate a table.
Matthew Halliday: So I can just change it, and look at here's the amounts against that data set of course I can go into different visualizations and i'll show you something built out, but today I don't want to focus on.
Matthew Halliday: Building dashboards I think all of us have seen that and a lot of experiences as similar definitely there's some uniqueness in terms of in quarter and the simplicity of how you can do it.
Matthew Halliday: But I really want to make sure that we don't get kind of caught up in the visual because.
Matthew Halliday: At the end of the day, the visualization is on something like this right every visualization has an most likely an aggregate behind it and you're putting in various values.
Matthew Halliday: The real hard work is how do you get to that the last bit is well how you visualize, it is up to you, so if I cancel out of here.
Matthew Halliday: i'll just accept and here i'm going to go and actually look at the schema that I created just to kind of show it to you.
Matthew Halliday: And so, in here i'll just do a search for my schema.
Matthew Halliday: Is the one I just created, and I can look at the diagram and you can see here that you know there's a lot of tables that come into play now all these tables.
Matthew Halliday: are what is actually the source application so every application has this this is your starting point.
Matthew Halliday: This is where you kind of begin everyone begins, from this point it's like where does the data come from, but it comes from, something that looks like this.
Matthew Halliday: Because I can change this, I have this in a compact view but if I do a default layout here, you can see it a little.
Matthew Halliday: Maybe a little easier and in terms of how you want to look at that data what's very clear here, though, that this is not a star schema, this is not a flattened structure, this is applications as they are just to show you a few other examples.
Matthew Halliday: let's go ahead and look at another schema I have here, this is an oracle EBS schema it's a product that I kind of cut my teeth on when I worked at Oracle.
Matthew Halliday: For a number of years, and if I look at the diagram here, you can see that this is, you know pretty complex.
Matthew Halliday: This is, you know all of the tables that come together to give you just an example of you know what's going on here, so if I.
Matthew Halliday: jump into some of these tables, you can see, are a customer transaction types are a batch, these are all source application tables, that you will find this is the big header right are a customer transactions or.
Matthew Halliday: And then, this one over here transaction lines this particular object is you know the backbone of Oracle.
Matthew Halliday: receivables and so these are all of the objects that are used directly off of that now.
Matthew Halliday: This is complicated enough and you're like Matthew Do you really want to put this in front of business users like picking be so complicated i'm getting any headache working in you know I understand.
Matthew Halliday: So this is where we say, well, this is not the way you want to interact with this data and honestly there's more than just this data to make this data really interesting.
Matthew Halliday: I probably want to use other pieces of data, alongside it like I want to get it, you know other jira tickets have been logged.
Matthew Halliday: What is there any calculations, we can look at you know, in terms of you know salesforce information or maybe write an ml that looks at some of these key parameters and does customer prediction for churn.
Matthew Halliday: And I want to look at those things, it could even be multiple earpiece systems most companies today have done some kind of acquisition at some point in time.
Matthew Halliday: And might find themselves in that situation where they have to lps or even more right bring those all into one place.
Matthew Halliday: So in our business schema this is really where the magic happens where you can create a lovely logical way for people to engage with the data.
Matthew Halliday: So, yes, we all start with that messy stuff right whether we're doing etfs and flattening and you have to do all the mess of you know coding your email to flatten it.
Matthew Halliday: or in quarters approach is a little different So here we have that source and now I have a customer 360 that I created.
Matthew Halliday: And if you look at my customer 360 you can see i've got you know customer 360 now this looks like a flat data set if I was to build on top of this, and i'll show it in the second.
Matthew Halliday: I can just grab fields and start building and it will feel like it was completely flat This is great.
Matthew Halliday: But if you look at where the objects have come from you can see, they all come from different places payment schedules customer transactions salesforce jira like.
Matthew Halliday: Information is coming from a variety of different systems, but I, as a business user do not need to know that.
Matthew Halliday: When I engage with this data, even though it's got that same messy model that we're looking at for all of that complexity.
Matthew Halliday: I just look at it this way, and I can build directly of this, and so I put my tablo or power bi or in quarter directly against this particular object and engage with this object now be very clear on this point.
Matthew Halliday: In quarter does not flatten the data behind the scenes it doesn't say Okay, we now understand you want this let's go ahead and create some kind of data model or a cube structure.
Matthew Halliday: We keep this as purely a logical semantic layer it's like pointers like symbolic links that just say here's how you can think about that data.
Matthew Halliday: here's how we can take away that complexity and make it really easy So if I need to add a column to this.
Matthew Halliday: Just drag and drop it in as long as there's a relationship between those two objects, not a problem.
Matthew Halliday: You can start to use it and so people can build directly on top of it, so this is generally the way that people would start to you know grab objects.
Matthew Halliday: Where this gets interesting is to show you a little more of an advanced dashboard here let's go look at some of our content.
Matthew Halliday: So here, I have a one of my favorite dashboards that i've that i've got, and this is showing you know customer retention.
Matthew Halliday: And so, this is actually going to cast a pretty sizable data set there's about 2 billion records and I didn't pointed out, but maybe if you are really keen eyes you're going to notice at the top, it said rose and had 2 billion.
Matthew Halliday: And this is aggregations being done on the fly against that data set.
Matthew Halliday: So, looking at you know my revenue amounts being able to look at you know tickets by customer.
Matthew Halliday: To be able to see all of these things brought together without having to go through a coding experience, without having to say Okay, this is how we plan this with a grain of record this is.
Matthew Halliday: The way we can print these dimensions here's how we're going to create a single fact table being caught up in that.
Matthew Halliday: This really does give you that capability of taking all the things we've been talking about today, and actually making them real.
Matthew Halliday: So I can come in here, I can start a search and you know I could start to say okay i'm looking for you know count name as a tape right its refining it.
Matthew Halliday: is changing, so I click on staples and I have refined everything down in this dashboard and recalculate everything down my turn probabilities change.
Matthew Halliday: I can look at the you know the different upsell opportunities the tickets.
Matthew Halliday: The amounts, etc, and the transactions, I can say Okay, I want to drill into this into further detail, so let me actually go and look at my dashboard behind this particular customer.
Matthew Halliday: So here, as I kind of go in and can see again a different view, I can see all of the details and the data that I wanted to be able to interact with.
Matthew Halliday: And I can you know see things like opportunities one revenue amounts and be able to understand exactly you know what's going on in terms of my transactions.
Matthew Halliday: So what this enables us to to be able to do is to really have this rich user experience in terms of bringing a lot of data that.
Matthew Halliday: Traditionally doesn't reside in the same products, the same applications.
Matthew Halliday: Not having to figure out Okay, what are the key questions that you really want to know, and let me build specifically for that.
Matthew Halliday: And then, of course, when you do an analysis you come up with some new set of questions and then you like shoot back to the drawing board.
Matthew Halliday: In product now it's very easy drag drop bring them bring those in bringing out of bringing complete different data sets and join them and then be able to create against them super super powerful so.
Matthew Halliday: that's a component of the product, here I did mention that you know, there are places, you can do coding, so let me just kind of show a little bit of that.
Matthew Halliday: And so here, you can you know come in, I mentioned customer churn So here we created a Python script so we do have notebooks I can enter this notebook i'll just add to this is a quick query here.
Matthew Halliday: And you can you can go through and create a really a you know, a Python machine learning gradient trees.
Matthew Halliday: A gradient boosting classifier in this case and be able to run that inside of the quarter platform.
Matthew Halliday: Now, why is this important right there's a lot of code, you can do these kind of coding, but what we're doing for the data scientists.
Matthew Halliday: is one of the things data scientists always have to start with, is, I need data to train my model on.
Matthew Halliday: And in that you need flattened data, you need a data frame and the data frame cannot be his first two tables and where you go, but you.
Matthew Halliday: encoder you can create that data frame just with a few dragon drops in that analyzer experience that we have showed create a table and then use that to feed into your machine learning.
Matthew Halliday: So makes it super easy for machine learning data scientists to actually do the very thing that you hired them to do.
Matthew Halliday: Not building up data, but actually doing data science work, and so this is just a really great experience for them to be able to very quickly.
Matthew Halliday: curate the data that they need to build to build that and then use the output of this particular machine learning algorithm as a table inside of a quarter.
Matthew Halliday: And so once we're out of this here, you can see that here's the output of that particular envy or that.
Matthew Halliday: Python script that's run and run on in quarters auto scale platforms, you can get additional resources for that.
Matthew Halliday: But here, you get you know the results, and then you can join us and use it with other pieces of the system, as well as you can see here, this is all joined, so if I cancel out of here and look at the diagram.
Matthew Halliday: can quickly see you know customer churn journey to customer accounts and that's the main way that customer accounts, obviously, then moves into a whole bunch of other things that have joined to.
Matthew Halliday: And so, this kind of continues, you can keep continue to build on top of top of these components so really gives you that flexibility to to move quickly to the needs and the demands of the business.
Matthew Halliday: But the one final thing I just want to point out here is when we talk about you know performance and we talked about you know, using the scale from things like.
Matthew Halliday: The cloud infrastructure and how we're able to you know libraries menomonie more machines.
Matthew Halliday: I always think what's interesting is is what this has done his mask some of the problems so some of the problems that people have is that you can.
Matthew Halliday: Now a query that would run can run, but it would use a ton of resources behind it, but you get resolved, and you pay a lot of money for that.
Matthew Halliday: The direct data mapping piece, though, is incredibly efficient.
Matthew Halliday: we've been able to run queries that would run on other cloud providers that would take like two hours to run on those and cost you around about 34 $35 that same query on inside of in quarter ran in less than 50 seconds 15 seconds it came out as a cost of about.
Matthew Halliday: I think it was about five cents for that particular query it's just an absolute huge difference when you compare the data between the two so.
Matthew Halliday: that's one thing definitely to be aware of is the efficiency right.
Matthew Halliday: If I put 100 nodes on something sure it's going to run you know potentially faster, to a certain point, until you hit network bandwidth limitations.
Matthew Halliday: But within quarter this direct data mapping really does transform this whole capability and this whole experience when it comes to getting them so much closer to real time and be able to ask the questions that you want to be able to ask.
Matthew Halliday: And without back to Eric.
Eric Kavanagh: yeah this is fantastic, we have a ton of great questions in the queue here but i'll just throw one.
Eric Kavanagh: broad question over to you Matthew and what I found so striking here is your ability to reach into so many different environments and I looked up.
Eric Kavanagh: The table, by the way, of all the different connectors you have 225 of them, or something 16 are in beta and almost 200 and some are in production now.
Eric Kavanagh: And really and maybe Matthew comment on this and then sanjeev comment as well the complexity of the modern business goes far beyond.
Eric Kavanagh: Traditional data warehousing as we've thought of it right, we were trying to force fit a square pegs into round holes basically to use that metaphor.
Eric Kavanagh: And you've kind of obviated that challenge, by enabling this design layer enabling the semantic layer
Eric Kavanagh: and doing this direct data mapping so to me that's what is most exciting, because for any given business.
Eric Kavanagh: They will be completely different set or mosaic of data sources and functions and analytics they want to do to get their job done, and you have opened the door for that entire tapestry, for whatever organization is that about right.
Matthew Halliday: yeah that's one thing that we love to do a proof of values right we say you know, bring in some some multiple systems let's bring in a problem that we actually can show how quickly we can do it and.
Matthew Halliday: it's quite amazing I was just listening this morning about a customer story where.
Matthew Halliday: They were quoted like eight months by a vendor to do the analytics.
Matthew Halliday: For that particular project and knowing quarter was like a couple of weeks literally a couple of weeks and we're able to turn that around and bringing these different source systems join them together and give that experience.
Matthew Halliday: it's it's radically different, but I think that's where the value is that's where our customers are saying this is where we really get to understand our business, not in isolated I understand collections, you need to understand collections.
Matthew Halliday: In relation to churn in relation to the customer issues into future pipeline to really understand you know what is going on and that's and that's kind of the magic of you know what in quarters, making available.
Eric Kavanagh: yeah sanjeev what are your thoughts on that because to me.
Eric Kavanagh: By not flattening the data that's what preserves so much context and richness, which you can then leverage as a business user and also it's easier to understand, I think you know flattening.
Eric Kavanagh: kind of flattens out your mind a bit, and you have to jump through some hoops to make it all work and then you have to address that on the back end when you're processing so by by not flattening I think they've solved, a lot of the challenges but sanjeev what's your what's your opinion.
Eric Kavanagh: I think our new you know me.
Eric Kavanagh: Being one So there you go.
Sanjeev Mohan: take it away yeah I agree everything that has been discussed, so I like in full agreement, I think, in the interest of time, we should probably move to some new questions that have come in.
Eric Kavanagh: yeah there are tons and tons of questions in the queue here i'm.
Sanjeev Mohan: bicycle one one quick question I want to answer somebody asked what is the difference between data fabric and data mesh so I want to get that out of the way data fabric isn't implementation approach.
Sanjeev Mohan: And data mesh is not data mesh like i've said many times, as a concept, so a data fabric, can be used on top of data mesh.
Eric Kavanagh: yeah now that's a very good point well let's let's focus on some of these specific questions I guess that have come across the transom here and folks will have someone reach out to you.
Eric Kavanagh: After the show if you feel a question was not answered but there's a question about json and how you deal with json with semi structured data and you already talked a bit about that Matthew but and you go into some more detail on how you, you can compute json.
Matthew Halliday: yeah so actually.
Matthew Halliday: The json structure, when you think about it is almost a relational model in disguise.
Matthew Halliday: Right it's just a different representation of how it's done so, the inquiry does that actually we we have an xml or json connector where you can look at those objects and then expand them into a relational model.
Matthew Halliday: And it will go through and it'll add those as necessary, so you you end up looking at it like this relational but yeah it's just coming directly from json so those kind of structure is not a problem.
Eric Kavanagh: Okay, good and let's see well no sequel databases, yes, you support no sequel like I said there's 225 of them out there, so you've got a whole heck of a lot of stuff going on.
Eric Kavanagh: mongo db support course etc on data frames let's talk about the business users and when they get into this environment and I love the way you have this screen, where you can.
Eric Kavanagh: Basically, facilitate the understanding of the different tables and what they mean and where they're coming from.
Eric Kavanagh: To me that's part of the magic as well Matthew because it's it's highly versatile do you wind up having to do a fair amount of training.
Eric Kavanagh: of users to get them to understand what's in there, or do they see it and then that kind of begins the exploration, so they can really dig into like the Oracle E business suite mappings and so forth talk about that for a second.
Matthew Halliday: yeah so um one of the things I didn't mention today.
Matthew Halliday: Is blueprints and quarter blueprints and they called blueprints are not blueprints for like a house where you're like okay here's a piece of paper go build it, it is much more like a building.
Matthew Halliday: And so what that provides is really all of the structures, the data models and even the business schemas behind it with some sample dashboards few bill to start to leverage and use that data.
Matthew Halliday: Of course, certain applications will have areas where not all the data is in the business friendly format, if you look at a status field, it has a K ze l you below what are those statuses mean and so.
Matthew Halliday: New quarter we provide those application logic to sit on top that will transform that into more of a meaningful value for the business users.
Matthew Halliday: So we do we do see that were business users can get up and running a lot faster, because that information is presented to them in a compelling way and that that's been very well received, so we have those blueprints for Oracle E business suite netsuite guide where salesforce.
Matthew Halliday: workday etc, and so there's a number of these options that we that we've built out to help with that and then yeah the.
Matthew Halliday: kind of experience when you're going through building and examining those structures and looking at it it's very intuitive because it's very much built on like.
Matthew Halliday: I never build anything with like 5060 tables out of the gate i'll grab an object and i'll say okay where's what's The next piece of information I want what is that reside, and then I just.
Matthew Halliday: touched that table bring it in so it becomes this really like additive agile process where you just keep adding to and it's really easy to just keep bolting on and adding understand oh let's bring this then.
Matthew Halliday: let's bring this in so you don't have to think it was like Oh, my goodness, I can't wrap my head around 200 things you might just start with.
Matthew Halliday: hey I want to do some payables analysis so like what are the key tables and payables okay.
Matthew Halliday: grab you know, maybe two tables at isn't lines you start from that, then you go, I wonder if and then those wonder riffs will naturally lead you to a place.
Matthew Halliday: That you didn't need to know all of those I wonder if up front, you can adjust and transform change them and be able to you know take those on board honestly and just you know minutes versus months.
Eric Kavanagh: You know i'm at the risk of getting philosophical i'm reminded of my favorite philosopher vic and Stein who wrote this book tractatus logie go philosophic is, which was very interesting but.
Eric Kavanagh: What he says, and when i'm thinking about this is because what you're talking about our building blocks of understanding.
Eric Kavanagh: Right you start with a couple objects you pull them together these learn something you got another object.
Eric Kavanagh: This is you're building your understanding of a particular scenario or aspect of your business right.
Eric Kavanagh: And then, once you get up into the cloud and that's kind of where in quarter isn't in my metaphor here.
Eric Kavanagh: That can sign talks about how you should use these elucidation is like a ladder to get up into the cloud, and once you've gotten the crowd you take the letter away.
Eric Kavanagh: And now you're in the analytical environment basically an eye view that's kind of what you're doing you're enabling the building blocks.
Eric Kavanagh: to piece together a structure in the mind that allows someone to wrap their head around what's really happening and then do the analysis and then go make some changes so it's really a it's a very component ties.
Eric Kavanagh: iterative environments that allows you to see into all these different avenues of the business right Matthew.
Matthew Halliday: Exactly yeah I think you nailed it right there.
Eric Kavanagh: that's very cool, we have a couple specific questions when attendees asking the state of copied and loaded as physical party files.
Eric Kavanagh: From the source systems into in quarters data platform and then maybe i'd love to hear sanjeev just talk for a second about parquet as the new norm, but Matthew thinking that's that specific question.
Matthew Halliday: yeah so you have.
Matthew Halliday: data stored in.
Matthew Halliday: Okay, so if this is accessible by different applications in quarters query engine will read from parquet and then, in conjunction with that we have this highly compressed direct data map that enables us to then run queries.
Matthew Halliday: Real time on the fly you know against that that paki data without the need to do any pre processing on it without the need for flattening it.
Matthew Halliday: Obviously, we read data, you know into memory, but one of the things that's very unique about in quarters just the way that we use compressed memory, and so we don't want to get too technical here but.
Matthew Halliday: When you bring data right normally it's compressed at rest, but once it's in memory gets uncompressed when you do join some data, most people are doing them.
Matthew Halliday: On uncompressed data we do the joins on compressed data what that means, if you think about like a cpu cpu has different levels of cash, which is the fastest memory on the machine l one l to l three cash.
Matthew Halliday: And there's like you know 10 milliseconds between the cpu and alwan cash our utilization of that year, or one cash is quite unique.
Matthew Halliday: Because of this compression because of the way that we can go through without flattening the data we find that the data is naturally in its original shape.
Matthew Halliday: how you can use it for us to be able to get highly performant queries where it hits our cash l one l to caches way higher than any other product out there.
Matthew Halliday: And so that's part of what you see this amazing performance from the quarter engine because that's a quite a technical thing that the manifestation of the value of that is.
Matthew Halliday: You get flattened performance without it ever been flattened it's not flat behind the scenes, is that the timer worry, we run the query get the results that and that's the nearest to a flattening you get is the result, set of your query.
Eric Kavanagh: that's really that's really interesting stuff i'm glad you went that deep frankly i'm.
Eric Kavanagh: understanding it better now myself sanjeev real quick you're almost out of time.
Eric Kavanagh: park hey I love that I think that's one of the best things that came out of the whole hadoop movement was this park a file for maximum part came in, or if there are other ways to.
Eric Kavanagh: To solve this challenge, but parquet has become the sort of de facto leader in that space, and I think that's good news for everybody up and down the stream all across industries for all use cases, what do you think.
Sanjeev Mohan: yeah absolutely a park a being a columnar format it's very fast to to get data specific data from the fight also Parker fights have a header.
Sanjeev Mohan: So the schema is is is there, as opposed to a csv file, where you don't know what the schema is and maybe the first line of the spreadsheet as a schema but you know so Part A.
Sanjeev Mohan: Is a much easier format to work with and it's very interesting what Matthew just said about when party goes into into memories and contrast and Matthew.
Sanjeev Mohan: This is probably not the right forum but i'm sure some of the technical people are thinking that way there's also Apache arrow right format which is like compressed columnar format in memory that works at parquet so is in quarter serve an alternative to arrow.
Matthew Halliday: yeah it's not we're not leveraging Apache arrow there's definitely I guess you know, to some degree, some similarities we we did look at Apache arrow but we're able to get.
Matthew Halliday: better performance, for what we're doing specifically around the relational data set so if you think about that it's it's slightly different problem different problem set.
Matthew Halliday: So there are some difference differences, but you know stay tuned everyone is interested in Apache arrow within quarter and, hopefully, we have some interesting updates provided in the future, for you.
Eric Kavanagh: Yes, and folks I just posted a link to an end quarter on jumpstart so you can click on that link, you can see it's cloud and quarter comm slash sign up if you want to use this stuff.
Eric Kavanagh: Using the proofs in the pudding when you start wrapping your head around how all this stuff actually works that's what you want to do.
Eric Kavanagh: What a fantastic session today, thank you sanjay mohan for your time and attention, thank you Matthew for going deep into the weeds here we've got.
Eric Kavanagh: Just a ton of great questions will pass those along to presenters today, and with that we're gonna bid you farewell, yes, you will get a recording yes to get the deck with that we're going to say goodbye thanks again so much folks take care bye bye.
Matthew Halliday: Thank you.
Sanjeev Mohan: Thank you right.
Hosted by:
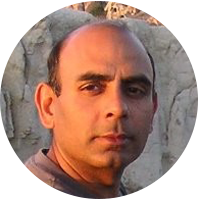
Sanjeev Mohan
Founder & Analyst at SanjMo
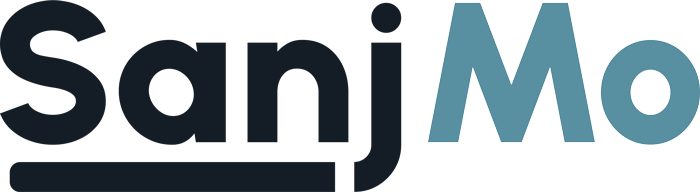
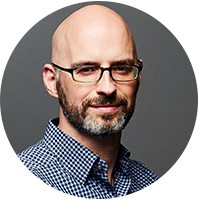
Matthew Halliday
Co-Founder and EVP of Product at Incorta
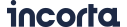
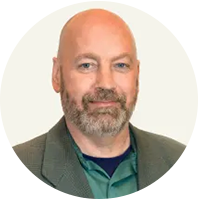
Eric Kavanagh
CEO at The Bloor Group
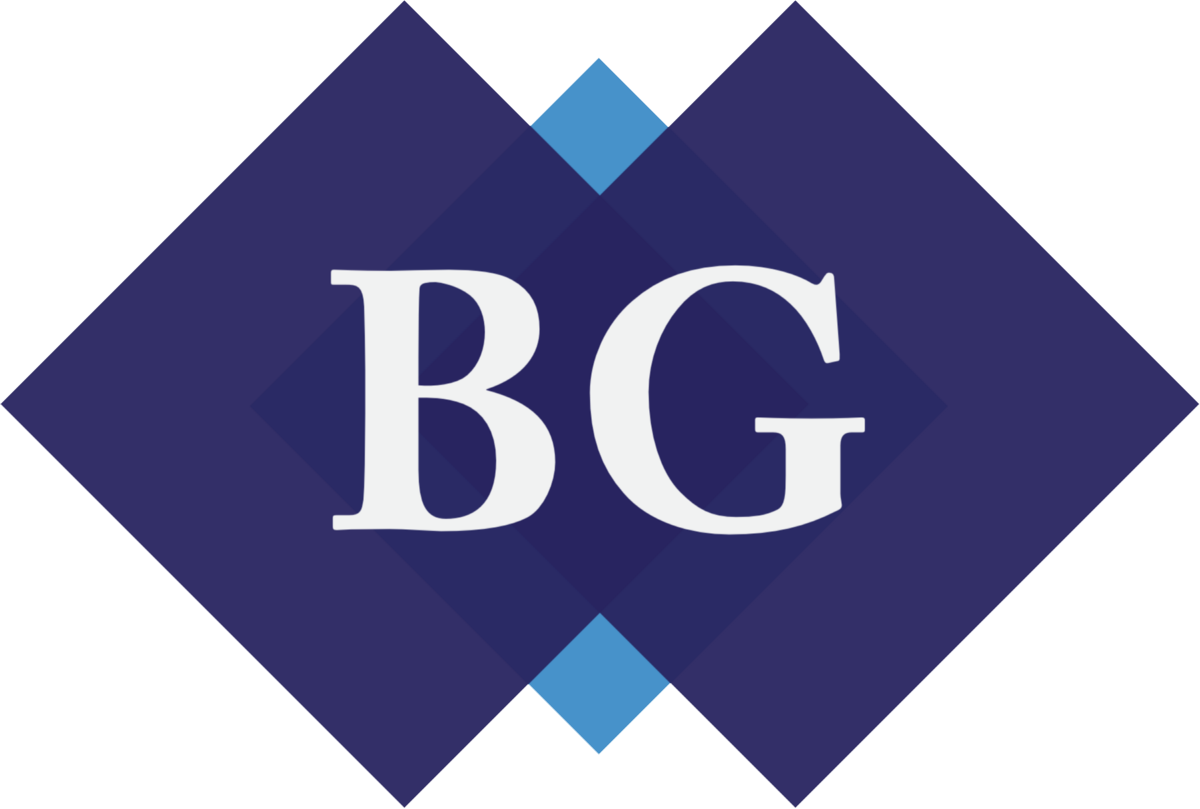