Beating the Data Delay: Building Data Value Faster for Financial Services
“Data delay” refers to the time lost when complex source systems, limited resources, or insufficient funding prevent the completion of a data project. Unfortunately, data delay is all too common in industries like financial services where data’s magnitude and complexity grow at an astounding rate.
But data delay can be overcome. By leaving the traditional data factory behind, you can empower business users to generate insights, grow revenue, improve profitability, and manage regulatory complexity on their own — all while remaining consistent and compliant.
- All the ways data delay significantly, negatively affects your financial services business.
- How a unified data analytics platform from Incorta can give any user revolutionary access to real-time data and accurate insights while leveraging your existing enterprise-system investments.
- How financial services companies like AXA use Incorta to eliminate data delay and get the data insights they need, whenever they need them
Transcription
Stephen Ibach: Good morning. Good afternoon. And good evening everyone. We're just going to take a few minutes to let some other folks join. Uh, and we'll get started in just a minute.
Stephen Ibach: As I said, uh, welcome everyone. Uh, good morning, good afternoon. And good evening to all of you who have joined us today. Uh, I'm very grateful to you. I'm here with my big headphones on so that no doubt means that I'm, uh, in another webinar for all of you. And, uh, my name is Steve and i.com the VP of industry solutions here at Incorta.
Stephen Ibach: I'm joined today by my colleague Margaret Dhabi, who is the senior sales engineer in the MENA region. And I'm going to hold off introducing our special guests today. Uh, from our wonderful and extremely grateful to him today for joining us, we'll be introducing him momentarily after a few slides. Um, thank you very much.
Stephen Ibach: As I said, for joining us today for beating the data delay, uh, building data value faster for financial services. And frankly, I think we could say beating, uh, building data value faster for any industry, not simply financial services. So to all of you on the call today, uh, thank you again for joining us and, uh, let's just dig right in.
Stephen Ibach: The first thing I wanted to start in talking about this is really setting up the definition or at least what I'm calling your, a working definition of the data delay. And in talking about this concept and in using it as a way to frame the story, um, on how in quarter really beats the data delay and how we are enabling and empowering gate engineers internally with the end for enterprise, and then ultimately business users.
Stephen Ibach: I started to think about word origins and everybody in Incorta always says to me, you're always talking about word origins. And I say, well, I think word origins give a lot of understanding and appreciation for what the true meaning of a word is. And in this case, uh, to our French brothers and sisters, we owe them a debt of gratitude because, uh, the word delayed.
Stephen Ibach: Uh, comes from deli aid, which means to dilute and, and ultimately the English definition is to make someone or something late or slow, or a period of time by which something is late. But what I want to really talk about is not only the time dimension here, but also the dementia and the notion of diluting the value within the enterprise and the data value within the enterprise.
Stephen Ibach: So it's not simply, as we would think a time delay. It is also a dilution of the enterprise value and the enterprise data value within the organization. And it's, there's an interesting nuance here. When you look at the French use of the word, the verb delay, it's also to waffle, to not be as exact in what you wanted to represent or the idea you want it to communicate.
Stephen Ibach: And so there's this notion and again, a working definition so that we can anchor to a concept is this is really about. Uh, diluting the enterprise data value, but it's also about limiting our data engineers that incredibly valuable resource and not having them be free to have the kind of agility Davy.
Stephen Ibach: They're an overburdened resource. So the data delay is this dilution of enterprise value, right? A lack of delivering ability to deliver actionable insights because of the limitations on our data engineers. And before we get into those limitations, a lot of the traditional approach and where we think the in quarter really shines.
Stephen Ibach: I just wanted to take a moment. Okay. And just say, uh, you know, surveys and studies, w we often quote some here I'm citing post Gartner and Accenture. Please reach out to me if you'd like to know, uh, the more detail on these studies, but I'm, I'm citing the source specifically. We'll look at three ideas here.
Stephen Ibach: Um, in the last two years of survey, the first is I think we would argue that Gartner on data and analytics and understanding of data analytics world and being the foremost researcher in that market and understanding both the technology and the enterprise approaches and data analytics in their study.
Stephen Ibach: 60% of respondents, uh, recorded and said that they weren't the lowest three levels of data analytics, maturity under Gartner's framework, 60% put themselves in the lower three runs. From a maturity perspective at the same time, Accenture has obviously had a strong and formidable approach in working with enterprises on their businesses and optimizing their businesses and of all firms that they were talking through with this study, 80% of them saw a volatile feature ahead of, for their business while in the face of driving by data-driven enterprise.
Stephen Ibach: And then lastly, This is a, I think a metric that is often quoted and discussed. 97% of data in firms goes on used 97%. Now I would argue that that percentage is going to come down dramatically as we move into solutions that are innovative and why court, uh, that are really giving, uh, driving that better agility with data engineers and also setting the stage for the utilization in ML and the promise of machine learning going forward.
Stephen Ibach: So we want to bring that number down dramatically, and we do it in spades and in quarter. And we're going to talk to you about why, but in the context of financial services, Let's take a moment and just dig a little bit deeper on the data to insights and recognize that the data delay is everywhere. And again, as I mentioned in studies and surveys, um, us to understanding and getting a sense, and I'm getting citing sources here at the bottom, but it begs 33% from this survey said they had a fully deployed analytic solution and a mere 21% said they were ready for real advanced analytics.
Stephen Ibach: At the same time in the asset management space, data integration, 57% of asset managers said data integration is a barrier to their business. And near 89% of them have said they have difficulty integrating data. And then lastly, in the insurance space, Almost 30, 50 plus percent, nearly half say legacy tech is a barrier to their success, ultimately, and I would argue being such a data driven business, and we're going to hear from an insurer, uh, later on that legacy tech is, uh, and that issue is really centered around getting better data agility going forward.
Stephen Ibach: And of course, uh, I would, uh, be remiss if I didn't mention that insurers are really looking for. Cost-effective solutions that are giving them really significant outcomes at a lower cost and a higher performance. Why do I highlight those initial surveys? And these here, I do that to say that exactly that the data delayed is everywhere and all of our, uh, regardless of some industry, all of financial services is really experiencing this.
Stephen Ibach: And as I said to start, I think we could see this in every industry, in every industry, across the world, in every company globally, every one of them is experiencing the data. Now again, to be more specific to financial services for a moment, uh, in the context of the series we just saw, I don't believe that financial services can afford to have a data delay.
Stephen Ibach: And I think it really comes down to what I'll call the three pillar position here. And the first is the relationship between revenue and profitability clearly within financial services, regardless of the sub industry that you're in, there is a significant revenue, revenue, pressure there's pressure in fees, across all lines of business, across varying sub-industries.
Stephen Ibach: I think that's a well-known position while at the same time, there is a pressure for profitability. So if we couple those two together, what we're really focused on is we've got to make more revenue and we've got to be more profitable, but we've got to do it with less investment. So we've got to do and make more with less.
Stephen Ibach: The second is coupling with a regulatory complexity with risk discovery. Let's talk about regulatory complexity. We know that the regulatory environment globally is extremely complex, requires a lot of data engineering and data coordination internally within organizations. We know that that complexity is just going to continue increased regardless of the region that you're in.
Stephen Ibach: Whether you us Europe, MENA, or Asia Pacific. And we also know, I mean, and let's just take a seat, car Cecil, uh, EPA reporting for ESG and the green asset ratio. I mean, the list can go on in terms of level of complexity for regulatory reporting, but also coupled with that is this notion of risk discovery.
Stephen Ibach: So we're guard plus counterparty risk, credit, risk market risk, systematic risk, any of those, uh, uh, risk categories that we would have all of them require. And as we move further and further into building new products and services, all of them require the data engineer to be able to coordinate across product across our systems and really get a full picture to understand where exposures are for firms and where they sit with it in the risk, their risk positions and their tolerances.
Stephen Ibach: And then lastly is this notion of digital digital transformation. And I'm coupling that to the FinTech market. Certainly every financial services. Is engaged in digital transformation. That is a data-driven exercise part excellence. And I think that what we have seen is the FinTech market has been exceptional at not only delivering terrific UX and customer experiences, but they've been exceptional at leveraging data.
Stephen Ibach: And so the large enterprises in conjunction with their cooperation and what I'll call their co-op petition with FinTech, they really have to recognize that their digital transformation efforts are all about data engineering. They're all about aggregating and coordinating and bringing systems together to really reduce the cost to serve the customer.
Stephen Ibach: So again, I don't think financial services given these six categories of real focus for them going forward can afford to have a data delay
Stephen Ibach: now. I think, and I really believe that the date of delay is coming from a traditional data factory and your data engineers internally and your enterprise. This is really their pain and notice there's an offset here, right? Because as business users within the organization, when we have questions, When we want to ask a question of data to get an answer, be it about a client or product or a client's engagement.
Stephen Ibach: We have to call it. We have to assess what kind of data sources we want to bring. We have to pull them all together. We have to establish them security, and then maybe we have to see it and use it and make it actionable. Well, the it teams, they're going to wait and garner your first set of requirements.
Stephen Ibach: And then they're going to run through the exhaustive, traditional data factory of having to do a lot of coordination from those source systems through ETL and transformation, more modeling, more transformation, more modeling, and then they're going to stage that data and make it available for dashboarding and Ford reporting at your utilization.
Stephen Ibach: But here's the problem. Not only is that an exhaustive process for the data engineer and it doesn't free them. To do the kind of Ford creative, really solid work to create new insight and new opportunity within the business. That answer that they deliver to you may engender new questions. And now you've got to go through this process all over again within a traditional data factory.
Stephen Ibach: So I really think that offset and that notion of a data delay, both for the data engineer, but also in the businesses collaboration with the data engineer is formidable. So what if we could. Give the data engineer, the freedom from that pain. What if we could free him from the pain and the exhausting process of the traditional approach?
Stephen Ibach: Right? So is it the ideal for the business to have a question, to be able to see all of that data available to them? And we know they can within a quarter and then generate new insights within minutes on that data and notice no offset. We work directly with the it team to collaborate immediately on the data sources we need, and we can get them out very, very quickly to deliver valuable and actionable insight and free the, the data engineer from, uh, having to do that burden of the traditional data factory and give them more opportunity to deliver value.
Stephen Ibach: That's what every data engineer in your enterprise wants to do. That's why they're a data engineer. If that's their expertise, they want to deliver terrific value to you going forward within the enterprise and deliver value to the. I'm going to pass the mic to mark now and let him focus on, uh, the court, a technical architecture and how we're empowering with the technical architecture, those data engineers.
Mark Agaiby: Mark. Thank you, Stephen. Um, hello everyone again. We'll be with you today. So I know once we hear the word pre-sales or sales engineer, a lot of technical terms are expected, but I'd like to challenge this concept. So I've started with something very kind of introductory to give you a clearer idea of understanding.
Mark Agaiby: Assuming that I am a traveler, which I am, and that visited, let's say the courtesy. Okay. And I would like. To have an attack of interest. The places I've visited, don't get on my own. Let's say with the engineering attitude of me, I like to build things themselves from scratch. So I create, let's say puzzle of 200, 500 pieces of the courtesy.
Mark Agaiby: That's perfect. But what would have happened if I had visited even tower in the same trip that I, and I want to keep them both into the same person, why the requirement is I want to do, I have to location two photos into the same person since they were part of the center. So how long it would take me to do this.
Mark Agaiby: Let's try to find out the answer for this. So the first part is I need to resize the effects and print. So maybe this might take a day, but this is equivalent to crystallizing the equivalent, the, in this case, The next step. It will take much more time. Why? Because this is related to the modeling. I need to cut this picture and paste it onto the Colosseum puzzle pieces.
Mark Agaiby: This will take a lot of time and most probably it will never be as accurate and as sharp as if I'm having this, took the picture from the beginning. After that, I need maybe two or three days to rebuild the whole puzzle again, after treatment, then the business. So this would be around eight to nine days with a pixelated that's not as clean or as precise as if it was manufactured from the beginning with this requirement cleared from the.
Mark Agaiby: Man-days using one mover, which is me in this case, the assembling one data engineer, a student who saying, even if you're trying to work things in parallel, then you cannot make it in one day because there are three sequential stages. So I think the most optimal I'll get to will be, uh, four days with maybe three disputes.
Mark Agaiby: So the output is average as possible. Not very good, but it's the most important pain here. It's not responsive to dynamic requirements that are needed to be molded into December. So who has that? I think the answer is quietly laborers. Lego here is giving you first even power in a three-dimensional mouses.
Mark Agaiby: This is adding this new dimension is taking the two D view of the puzzle into much more, really insightful dimension that. Moreover, it's very easy to add this for data sources. So adding the Coliseum when maximum technique one day, why? Because, and using the same one data engineer, because it's giving me the replying to the agility of the ever-changing requirements without the need to do every time, the modeling with every new business card and the last and most important part is the legal concept is giving you the access to the raw materials.
Mark Agaiby: I assume that one day before the trip I've changed that to be instead of a Euro trip, it will be only a Paris trip. So in less than a day, I will demolish that. Couldn't see him and I'll replace it with channeling his aid and very ability to adjust to new requirement without being only restricted to the pre defined kind of requirements.
Mark Agaiby: So again, the fact that you need to always keep in mind if the flexibility and I think label now is very clear after a simple illustration, that Lego is motor resembling. The idea of Incorta where the proposition of, so please. Put this in my wallet illustrate the architecture. Now the whole concept is about freeing your data engineer sources.
Mark Agaiby: It's not anymore the burden on the data to reply to a business card in the opposite with the proposition I'm giving the business users more opportunity to come up with new questions. And at the same time, I'm giving the data engineer to be as fast and as responsive to these requirements with them. So again, this is kind of the old way.
Mark Agaiby: Again, the data, how the methodology was kind of revolutionary in its time 40 years ago, and it might be appropriate for these tactical. But in the new digital transformation world we're living in with every new day I'm having in a stream of data and new dimension that needs to be taken into consideration into my data.
Mark Agaiby: More that it would consume tremendous number and hours of your data engineers to work on these three stages first, to start your transformation part based on your previous defined requirements, and then do the modeling in order to build it. I mentioned a little bit into a star schema. This will be the only way that the data warehouse will be available for the visualization tool.
Mark Agaiby: That's what I'm report. This is the only way the BI wouldn't be able to transact this data. So the problem here is. That's the most valuable resource or the most valuable new enterprise, your data engineer, our lock into this kind of traditional data factory consuming most of their time doing the same repetitive tasks for every new business record.
Mark Agaiby: So how the story is different. And this is what we'll see kind of the evolution really different because we're creating a direct data path directly between all the data sources you're having and your data consumers. So relying on the middle, it will be first, you need acquisition part will be loading the data, and then it will be available into our Incorta smart data lake ready in, in competitively, fast, in very analytics of Incorta engines that will avail hundreds of millions, billions of records to all of your data consumers in just a few clicks in a few seconds.
Mark Agaiby: We'll talk more about each of these four components in the next. But what's important here to show the meeting quarter pressure proposition. It's coming to an adjust data as is in its level, without any shipping or remodeling. And without vacancy that we'll be talking about it in more detail, it will be kind of zillions of record of these transactional payments into your analytics fingertips without any need to be into the same old data warehouse modeling with us unnecessarily, continuing your data entry resources.
Mark Agaiby: So this slide is what I'm calling the anatomy of a quarter. So now we'll be digging deep to see more about what each of the four components openly speaking to your data, engineers, superhero, but still I'll be trying to make it simpler for the business people to understand more the capabilities and roles of each of these four components without getting deep into the technicalities of.
Mark Agaiby: So there's only starts here with the load, the loader part that connecting the court, that your data sources are very long list of connectors, more than 200 connects with them integrating to all your data sources to increase the congestions filter the auto detection part. Here is one feature about connectors that understand all the relations are doing between your transactional data table before loading it into our kind of smart leader.
Mark Agaiby: So this will be the second part. The data will be loaded as is without any monolingual transformation, the same transactional payment with the same transactional record or save the hidden party. Okay. Case an open standard that can be accessed by any third party tool. And it's a columnar, which means higher compression ratio.
Mark Agaiby: So hundred gig on your data source will be 20 to 40 maximum onto the parquet. And this will be the data actually the rows of the into the parquet, but the showings smartness of data, the relationship will be saved here into the meta data and data mapping, which is our DNA. We'll be doing this glue between these two islands in order to be available for the analyzer analyze.
Mark Agaiby: That is what I like to call our kind of magic hat, which is finally making the dark data mapping tangible. What it does. It's intelligently pulling the data from the party while calculating the optimal aquatic plan between the tables from the meta data returns. So this is quite complex. Technically I'll assume it in kind of a scenario assume that I'm building an accounts receivable analysis.
Mark Agaiby: That's aggravating, let's say 15 columns of data from 10 different teams in our AR scheme. So this is where the analyzer will be intelligently pulling the data, maybe 500 million plus of these rows from these 10 tables. And at the same time building the need, the joins between these statements, all of this into our in-memory, uncompatible kind of fast performance analytics.
Mark Agaiby: I know this is too good to be a belief, but the technical people will not believe 10 VC because this was impossible in a step. Boom. And without doing the model important or the star schema, but when it's possible on the transaction, even without the modeling, the output of this analyzer can be shown in the visualization suite, which is very advanced wedding quarter right now, 60 to 70% of our customer gradually migrated to solely depend on our visualization tool.
Mark Agaiby: What's the 30% are still using their third party BI tools that can use kind of mix of the benefits of both worlds, the visualization aspects. You weren't used to it. Your execs get used to it from the BI tools where the uncompatible fast in the medical abilities of quarter. So this will be the story of the in-memory analyze that end-to-end the dark data management part.
Mark Agaiby: We used to say before that it was kind of. All of our cards now are using spark because spark is an open style. Any data scientist, one on people know how to deal with it. It's coming embedded what the quarter with forest as cutting light by therms color, orange secret. So what that does help you with it's embedded with the coordinator to write your own scripts on your own, that books to do all the business logically, whether this business logic would be kind of cleaning and preparation of data, or much more advanced kind of AI machine learning, predictive models.
Mark Agaiby: It's coming into one component and toy to give you one reporting layer on top of it from the budget, maybe the predictive model at the same time with the actual model. So in a nutshell, this was the anatomy quarter. I know it was maybe five minutes tough for business persona. I've tried to make it as simple as possible to show how saving your data because it's not the shipping data.
Mark Agaiby: And at the same time was offering on compared fast and they come back with extremely less consumption of your data engineering resources. I live in, in the Mike now for Steven to lead us back to the different business use cases that Incorta to achieve in the different kind of, uh, different financial services enterprises with again, Mark.
Stephen Ibach: Thank you very much. Um, and, uh, let's just talk about that, uh, in beauty, in the data delay here and, uh, talk to some news cases and certainly get to our special guests. Um, but the first scenario I wanted to cover here was, uh, we did some very solid work for a large Swiss bank. Um, and what I'm calling it is going beyond CRM and capital markets and really what this was a focus on was to sit down and we're all familiar.
Stephen Ibach: Uh, with CRM and the leveraging of CRM, but really there's a strong limitation. When you start building out a, a really work context-driven understanding of the customer from a variety of sources. So as you can see, I'm running the business technology, and then ultimately talking to you about the delay here, um, in the traditional approach.
Stephen Ibach: So let's go through the use case. So in this case, any CRM, in this case, the utilization of Salesforce, this client was basically asked the question, well, I don't see the client data that I need it in the context that I need it. And I know that data is in there in Salesforce, in that technical platform. So how do I get at it and utilize it?
Stephen Ibach: And mark, I love the idea of that, of raw materials, right? Uh, we always hear that the, the analogies data as oil data as capital, um, here, you know, we have a source system that has. That raw material that we need to get out so that we can build that Sean's, Lily's a understanding of the customer, but we just can't get to it.
Stephen Ibach: So what do we ended up doing? We ended up going to the internal Salesforce resource and they drive that value for us. And maybe that takes a day or two, but then suddenly this investment bank started to ask a question and they said, well, how does this specific client's set of interactions relate to their holdings information?
Stephen Ibach: And in this case, the 13 F filings that are done for regulatory purposes here in the U S well now for this client, coordinate that CRM against the 13 F filing, they had another two days. Well, of course, then we have this notion of, well, what's the dimensionality of the company, fundamentals of these companies, as they relate to those client engagements, you see what's happening here.
Stephen Ibach: As we try to grow context and get a more richer understanding of the customer, we end up inevitably having to have more data engineering and applied more resource to the problem. So now we also have a constant today update. We're gonna have to have a constant updating here as company fundamentals change like PE market cap, price changes.
Stephen Ibach: But then we take all of that and we say, well, maybe there are services we deliver to those customers. And we want to understand how they're engaging with those services. Purpose-built applications, um, our own products and services, get the usage and engagement metrics and align that back to how that's relating to their holdings in our company, fundamental information and our engagement with them.
Stephen Ibach: Again, that could be two to five days with constant updating. And if I want to be a financial services organization, that's bringing to bear all of the services within the organization, to the customer. I need to understand how my client's exposure, um, you know, is internally in my organization, outside of my line of business, right?
Stephen Ibach: And other product lines, what other businesses services are they utilizing? What we all know when in, in my experience, in the past, and certainly in talking with all of our customers, that can be an incredible, that could be weeks or months worth of work to generate and have an understanding on, in coordinating across lines of business, especially for a very large diversified financial or very large Swiss bank with a strong presence in investment management, this is a really difficult problem to solve.
Stephen Ibach: But then even on top of that, how does all of everything that I've done relate to performance? And if you're within financial services, I think you can recognize that this kind of compounding problem really exists when we want to get a really insightful position, uh, uh, on our customer and ultimately act on it and build actionable insight.
Stephen Ibach: So there is in many ways, uh, A really long delay, almost three weeks here. I think it's conservative to say that to really get a full view on the customer. Now for this customer, they didn't have to do the constant hunting, doing the constant permission and the constant updating and losing all that time to value on their data.
Stephen Ibach: What they did is they leveraged in quarter and they went to all of those disparate data sources with their high degree of complexity and their high volumes, Salesforce, 13 F filings. We know company fundamental data in 13 F filings are massive. We know that the data in CRM is massive. And what did they did?
Stephen Ibach: They left their data. Engineers leveraged Incorta to connect across all of those source systems. And now immediately be able to build and answer those questions that they were asking about the customer and iterate across that data very, very quickly. And by the way, it begged the question from the previous, uh, uh, before in court.
Stephen Ibach: It's still requires full security, right? From the source system. You've got to manage that data and govern that data appropriately. That's all handled within the Incorta platform. It requires full fidelity. There's no reshaping or remodeling here. You know, you're getting the data that's within the source system.
Stephen Ibach: You're getting near real-time updates on the Incorta platform with extreme query performance. We heard that from mark, right? We're going from raw material straight to the, to laissez straight to that insight that you want for the business and the organization. And what only putting the data engineer in the position to have to do business value at a transformation, leveraging their own insight and collaboration with the business.
Stephen Ibach: It frees them up to be faster and have greater agility internally in the enterprise. So this was in a terrific use case to really demonstrate and highlight how Incorta, uh, solve this. For this really large, uh, global Swiss investment bank, um, and giving them greater discovery, greater agility, and letting them really ask client questions and get real answers.
Stephen Ibach: It's certainly a competitive advantage. And with that, uh, I can't be more grateful myself to introduce, uh, our customer access. Talk about, uh, the customer use cases that we've seen in leveraged their common core. Hasn't helped AXA and I'm very, very pleased and grateful. Uh, first to access Saudi Arabia to allowing us to, uh, have, uh, Huston Abdel Rahman.
Stephen Ibach: I do not have to tell you about access Saudi Arabia. They're one of the largest KSA insurers and certainly, uh, access Saudi Arabia is one of 61 entities of AXA global. They have more than 370 employees in Saudi Arabia, and we know AXA is extremely well known for delivering solutions that are reliable, that are really meeting the needs of customers in auto travel, home and health insurance.
Stephen Ibach: Uh, as I said, I can't be more grateful than to have, uh, Huston Abdel-Rahman. He's an ITC ner, uh, architect manager at AXA joining us today. So Husson welcome. And thank you so much for being here. Thank you, Steven. I'm I'm so great. Grateful to you. And before we come in and talk about AXA and your architecture of a use case, and we dig in just, I would love for you to tell us just a little bit about yourself and your role.
Stephen Ibach: Um, it, senior architect manager, I'd really call you a, uh, senior data engineer, really delivering on that data engineering value to the enterprise. So tell us a little bit about your role at AXA. Okay.
Hassan Abdulrahman: Uh, an access to manage the architecture. Yeah, we have different responsibilities that we are managing, like architecture, data architecture, AI, machine learning, uh, technical architecture, all these lines.
Hassan Abdulrahman: We are managing in AXA, uh, and, uh, humble. We are doing a great progress in all areas, especially in the data architecture part. Uh, especially after we, uh, where we are working, we were working with, uh, with Incorta. We are doing a good progress in this area, which is, uh, becoming the core of all our innovations and you, uh, uh, transformation projects, uh, like CRM, like paper properties, and casualties application in healthcare.
Hassan Abdulrahman: And also in SIA, as I mentioned in CRM and. So an AI and machine learning, uh, we have successfully built a data layer using, uh, with the help with Incorta, uh, facilitating a lot, the extraction of the data from the core applications to be ready for all the new applications that we are developing.
Hassan Abdulrahman: Fantastic. So tell me, tell me about your previous architecture. Tell me about your before in quarter. Okay. Before in court, we were facing a lot of, uh, difficulties preparing the, uh, reports and the data insights for the company. We were heavily, depending on an Oracle application, uh, deal security queries that were executed, uh, in the, in the database using some store procedures.
Hassan Abdulrahman: And those were delaying a lot, uh, our performance and preparing the reports to the regulatory. And we were facing a lot of penalties. Most of these reports were CME or manual reporting. We were extracting the data from the core applications and preparing them in Excel sheets to the end users or the data scientists or that the engineers to use them individualization.
Hassan Abdulrahman: And they were doing a lot of work and a lot of efforts and time consuming. You can imagine for a single report, we will have. Uh, a specific FTE fully dedicated for one month to prepare such a report that will come to like summary reports. As an example, we will come in more details. So, uh, we were, uh, highly relying on ad hoc query and also a highlight on the core application, impacting all business operations and other applications because executing a huge amount of queries to extract a huge amount of data we're consuming all the machines or all the CPU's, uh, resources and memory, which were impacting the daily work for, for all, for our end users and for our clients.
Hassan Abdulrahman: And we're causing a slowness in the database for cool applications, uh, also heavily relying on it, it developers, or it members and the vendor who were developing or developing the core application, uh, or end-user. Computing using Ms. Excel sheets and the most of the formulas they were doing it manually in the Excel sheets, which were consuming a lot of time from them.
Hassan Abdulrahman: And, uh, the, the, the, the error or the mistake percentage were very high. It was very high. So, uh, the accuracy of the, uh, of the reports was not, was not in that way. We want to re to present to the regulatory because, you know, in such fields, the error percentage or average should be very, very minimal. Only one, one column could cause a huge banality for the company.
Stephen Ibach: Uh, It's incredible Hassan, the way you're talking about it as a data engineer, or you're, as mark had mentioned in his metaphor, pulling that raw material out, and then going through all of this effort to then have raw material again, that has to be worked on and worked on over and over, uh, and doing it.
Stephen Ibach: It just, uh, it sounds like it was extraordinarily difficult in that regard to, to get this, to, to have this kind of architecture. That's not empowering you. I'm curious. What did the architecture look like after in quarter?
Hassan Abdulrahman: Okay. ,Incorta uh, actually it gives us a lot of capabilities to organize the work, uh, especially to handle the data extraction, which we're consuming a lot of time.
Hassan Abdulrahman: You can imagine that for some reports that extraction were taking. Uh, two weeks or one month, even for one month for some, some reports and sometimes the operation of that extraction where, uh, uh, corrupted or, uh, or field. And we have to rework again from scratch to prepare the data, uh, fixing this issue, using Incorta and preparing the data directly.
Hassan Abdulrahman: We were able to extract millions of free course in milliseconds, and we were able to extract about 20 year data for some reports in less than one hour. Reshaping, we don't, we don't have to reshape the data. As mark has mentioned in his slides, we don't have to reshape the data to be presented or visualized to the end-users from Incorta directly.
Hassan Abdulrahman: We would, depending on the capabilities of that extraction, our modeling, uh, and visualizing the data directly because also it's not as the gap between storing the data in data warehouses and visualizing. You know, the guy, there was a big gap between the business needs and the data stored there and that a big gap between the business subject matter experts and the data engineers, uh, to transfer this knowledge from, from the subject matter experts to the data engineers in the previous architecture was very difficult because it was consuming a lot of time to prepare, I think, I think report, but using Incorta, we gathered them together in the same session, on the same table to transfer the knowledge and understand each, each, each element of the business itself, which I facilitated a lot, presenting the data to the end user and to hire.
Stephen Ibach: So incredible and opportunity to collaborate with business knowledge, get the data engineers involved in the high value tasking and delivering for the business and really getting a collaborative dynamic between the business and the data engineers. It really sounds like not only did in court, uh, re re reduce the time to market on the reporting and requirements that you had and beat that data delay, but you also had the opportunity to build a stronger collaboration between the business and the data engineers to deliver more value to.
Hassan Abdulrahman: Exactly Steven I'll also one of the major challenges we were facing is how to translate that data, uh, that the naming convention to the end user, because it's storing the data in the database or the data sources, you know, mostly the naming convention, doesn't, doesn't Yanni. It doesn't have a meaningful, uh, meaningful names or, or, uh, giving ideas.
Hassan Abdulrahman: What are those data? So that gives us the capability to build another layer above the data layer, which is the business layer. Which is naming the, the, the data or the columns with the business names and which facilitated a lot, the, uh, the, the, the communication between the data engineer and the business business owners, uh, and also the use the dependency on the it and the data engineers, because at this moment, the, uh, the business owners can understand that the businesses.
Hassan Abdulrahman: Uh, previous Lizzie will not able to understand the data schema, and now they can understand the business schema, which we have built upon the data layer and they can build their own reports or insights or KPIs without any need, without need any need of data engineers or a specialist from it, which also give us a lot of capability, a lot of, uh, comfortability, uh, on, on focusing on the architecture and enhancing the performance and enhancing the operation for other, uh, a very complex, uh, reports, uh, and KPIs.
Stephen Ibach: So fantastic. So you're, you're really driving. I mean, in this case, we're going to talk about medical claims analysis and we're really talking about the aggregate KPIs and what you weren't able to do with that immediate dashboarding. Uh, it seems like it is this like a really strong example of what you were doing in AXA, uh, on that first use case in terms of it just the complexity of.
Hassan Abdulrahman: Yes. Yes. This use case especially has a special memory for us because it was the first use case we have implemented the will Incorta and it gave us a lot of insights and a lot of indications about how is that how our data is stored and how we can handle it in a better way. Uh, you can imagine for this, for such a report, it contains the data from 20 years.
Hassan Abdulrahman: And, uh, we have extracted, uh, those data to about 400 million records and less than one hour previously, we were doing this report more on monthly basis and it was taking two weeks to be prepared. And the most of the time it was failing because of the, uh, uh, the deadlock or the user logging on the database.
Hassan Abdulrahman: So we, it was a rework and a huge, a huge work and difficult work to prepare this report. Now, after Incorta, we were able to extract all this huge amount of data in. And visualize it in seconds. And also adding a lot of filters and the capabilities to the end user on how they can present the data in different shapes and how they can extract from the same report, multiple reports, tens of reports and KPIs from the thing from the same data source.
Hassan Abdulrahman: As an example, you can, you can see here that we have aggregated. You can keep it. On total gross bay, the amount and the number of claims, uh, the providers, the nationalities, uh, gender, is there a lot of other, uh, inside KPIs that we will, we can filter on, uh, based on this, uh, to present the data also that's Hassan.
Stephen Ibach: This seems to me like you were able to very, very quickly deliver value. And as a data engineer working with the business, you could immediately address this, um, and do it quickly in collaboration and not have to focus on the tedious task. If you will, if the data delay in that traditional approach, I I'd love to talk, um, you know, also about the Samara report, just because of its regulatory complexity.
Stephen Ibach: And I'll build in through the slide now, but talk to us a little bit about this difficulty in generating the samurai report and, and doing this even quarterly, how difficult it was.
Hassan Abdulrahman: Uh, one thing I would like to highlight for the previous one, because of, you know, that the data delay impacts medical claims approvals.
Hassan Abdulrahman: We have a specific period to reply back on each approval or each claim. If we exceeded this time, we will be punished by the regulatory. Uh, so if there are any delay in this, we were facing a lot of penalties from, from, from CCHI, uh, uh, the head council of health council. So, uh, after Incorta, after extracting the data, we'll handle that we are not facing this issue at all.
Hassan Abdulrahman: And that's incredible for summary report this one of the most complex or sophisticated reports I have. We are generating this report from about 12 report from the core application. And as I mentioned, we were hiring a fully dedicated resource, especially to prepare this report. It contains about 50% of AXA data, uh, that we have to present monthly to, uh, Selma, which is a, like a central central bank in Saudi Arabia.
Hassan Abdulrahman: And if there is any mistake in this report, we will face in reality, uh, and huge venality from summer because it contains all the data related to health care, all our clients and members, a number of, of, of, uh, of, uh, of health number of, uh, of, of lives. Uh, the total amounts we are paying, put a number of claims.
Hassan Abdulrahman: The net premium, gross premium. A lot of calculations are very in this report. To gather or to combine this report with taking one month from, from, from an employee to do this. And he was only doing this. We handler handler. When we started working on this, you can imagine that we were working on field by field.
Hassan Abdulrahman: Each field of this report has its own criteria and own way of how to extract the data and how to predict. And there is a lot of dependency between the fields on each other. Also the level of the and the level of the data, which is stored in the database itself. You can imagine that only a single field can, we can bring the data from 10, 10 tables from the database to present only one field in this, in this report.
Stephen Ibach: And it's incredible because what you're really highlighting here I'd fit given the process. And the complexity is that regulatory complexity that we're talking about before. And just the fact that also as evidenced by this path and the technology, the data engineers are constantly doing ETL, constantly having to pull data together, take that raw material and pull it together and pull it together.
Stephen Ibach: And they only get that from a, you know, have to do that for every single provider. They have to do a calculation for every field. And there's a great deal of. Uh, at stake here with this report, as you mentioned, it's a regulatory report. It's absolutely required. It's one of the key elements that has to be delivered from a regulatory perspective, from exercise to the, to the regulator.
Stephen Ibach: I love to get a sense from you, um, you know, uh, uh, post that, uh, what happened, I mean, did, did you have the kind of performance that you were looking for, the creation that you were looking for? Did you, were you able to avoid those mistakes that you had referred to and re and deliver faster to the regulator?
Stephen Ibach: Exactly. I just want to highlight, I will tell you that I have spent about one month to just understand. The temporary self, not
Stephen Ibach: can imagine how it is complex. Uh, after, after we have used Incorta, we have spent a lot of time to understand it and to prepare the data. Uh, actually it was a great effort from, from Incorta team and from our business team to transfer the knowledge and to prepare this data, to write the formulas that the prepared the algorithms and this and that.
Stephen Ibach: Now you can, you can see that we can filter the data or can prepare the data with milliseconds. You can extract any amount of data for any specific period. For three years, you have translator for three years, not only for one month using any filter of the data, using the clients, the policies, the total amount, uh, the number of lives, uh, the, the insurers that.
Stephen Ibach: All this, you can, you can extract, or I can prepare for the end user in milliseconds, just changing filters. So you've got the kind of requisite data agility that you need in the face of something so complex, as you said, you're, you're, you're pouring over simply the template for a month to really get an understanding of it and, and understanding what kind of raw materials you've got to aggregate and bring together from a data side to then populate that report.
Stephen Ibach: I mean, I think it's fantastic, you know, you, you really have beaten the data delay and it, it feels like, uh, there are some really direct elements, uh, that you were using in the legacy approach to beating the data. Did I, and, and I just, if you could close on those, like, what were the things that you really think we got out of, uh, leveraging in court, uh, uh, in both of these use cases, this,
Hassan Abdulrahman: this, this slide summarize what we were facing before.
Hassan Abdulrahman: What we are doing currently, what, what we have currently with Incorta and the legacy systems or the previous architecture, we were limited. And we have limited ability to view the data across tables and periods. As I told you to prepare a report for one for one month or a one month, we were spending a lot of time and to change it, we have to rework again, don't do the work again manually.
Hassan Abdulrahman: And now for one quarter, we have unlimited access to all the data ingested from the source systems or any system. Also, you can imagine that we can combine data from different data sources, and we already implemented this. We have a claims system and we have approval systems. We succeeded to link between the approvals and claims you just click on the approval, go through the other big, all the claims related to this.
Hassan Abdulrahman: This is to disregard traffic so we can combine between the total you'll have unlimited access to all the resources. Also using queries with manual transformation to teach together. Uh, this wasn't that we were facing on that legacy system, and now only value added transformation for, for the use cases.
Hassan Abdulrahman: Uh, as the data is aggregated and cannot be drilled down into transaction level details. Uh, and as we have explained, if you want to drill down, you have to do their work again, and you have to build from scratch, everything you have to, uh, extract the data was different queries, which were adding additional time and additional efforts.
Hassan Abdulrahman: Now there's like, like you can just click or filter. You can drill down to any level of data that delivers data drill down is to supply, spend details, uh, showing the PO lines, uh, also for the solution, uh, overhead, uh, it was heavy. It involvement for, uh, additional reporting requirements. We have, we have to develop.
Hassan Abdulrahman: Uh, any report you can, you can, you can, you can, um, understand that for Oracle reports six, if you want to generate a new report or add a new field, you have to develop it and you have to use developers. So that dependency on it was very, very heavy. Now it is, as I have explained that we, after building the business schema, the end-users not it specialists can build their own reports or.
Stephen Ibach: Yes. And it sounds to me like you've now, uh, I think certainly what you've demonstrated to us is that in court to let you beat that data delay and also as a data engineer or really be empowered to deliver, deliver new value to the enterprise and to the business users. And I have to tell you Hassan, um, I am extraordinarily grateful to you, uh, for joining us today.
Stephen Ibach: Uh, Shaquan Justin Long, my friend, you have doing nothing but perfect for us in talking about the Incorta value proposition. So thank you very much.
Hassan Abdulrahman: You are so welcome. Thank you very much.
Stephen Ibach: Very grateful. Thank you so much. Uh, Hoston, Abdel, Rahman from AXA, and I would encourage, um, all of you, uh, on the call today that have joined us, you know, engage with us in further discussion. We have a number of other use cases in a number of other clients within financial services and across industries, where we are really modernizing the office of finance, where we're really bringing new value to core banking reporting across banking source systems, and also moving that to machine learning.
Stephen Ibach: We've got a great story to tell there. Um, we're doing an awful lot of work in enhancing the AML process. Do signals false positives and engaging, uh, on the SAR report for the regulatory. And of course, I spoke to you about doing real true customer 360, which is about taking all those customer information that is the raw material to really understand the customer and service them appropriately.
Stephen Ibach: So I would invite all of you on this call, uh, to join us and come talk to us and in coordinating. Um, certainly to, uh, we can talk to you about these other use cases in more detail and particularly that pillar of modernizing. So finance, uh, we can really demonstrate, uh, the value across all of these use cases.
Stephen Ibach: I guess I am so grateful to HASA for joining us today. I'm incredibly grateful to my colleague, uh, mark Agaiby, uh, in the MENA region. Um, and I would just say to all of you on this call, thank you so much. Uh, we'll take a minute, uh, in the interest of time for questions, but so very grateful to you, everyone who joined today, uh, and, uh, listen to how we believe you can beat the data delay, uh, certainly in financial services, but in any industry.
Stephen Ibach: So thank you. And we'll see if we can take any questions for a few minutes.
Stephen Ibach: Okay, well, what's that if we didn't have any questions from the field, I would just say to all of you again, thank you so much, mark and I are so grateful for joining. We had a terrific, uh, participant here in Hoston. Uh, certainly, uh, we are very grateful to him as a, both a customer and a partner and a friend.
Stephen Ibach: And, uh, we thank him so much for his insight and his storytelling today on how he beat the data delay. We hope all of you will beat the data delay too. So join us here at Incorta let's become partners, uh, in beating the data it's away. Uh, someone has raised a question. Is there any prerequisites for this system?
Stephen Ibach: Again, Incorta as a platform, uh, we need, um, it has two kinds of, uh, classroom implementation, whether it's on cloud or on prem, uh, in case there is a need for long term and we need, uh, Nunes with the normal hardware sizing and we can discuss. Yeah. And motivates after understanding the exact data sources you're having to propose the kind of starting with EBITDA.
Stephen Ibach: Let's start with a solid use case that will make you understand how the quarter is bridging the gap between the data sources to. The data insights directly without heavily dependent on the operational and data engineers, a lot of consumption. And I, and I think more broadly speaking, first of all, thank you for, uh, seeing the question.
Stephen Ibach: I apologize. I think the other aspect of, to that is, um, the prerequisite for the system. No, I think that the platform gives you such a high degree of flexibility. You understand? I think from Mark's insight here at a high level, there are really no prerequisites. We can connect to any source system and bring that data over and leverage it, uh, almost immediately for insight out to the business and really empower your data engineers, as we said.
Stephen Ibach: So, uh, the short answer for that would be, no, I don't think there's any prerequisite. I think if you come to one corner with a problem, we're gonna solve it.
Stephen Ibach: Any other questions?
Stephen Ibach: Uh, that's a terrific question. Yeah, absolutely. Um, we, we connect to this and I'll have mark answer this question as well, but the question that was asked was, uh, how does Incorta, uh, affected the source system? Um, and ultimately, um, we're connecting to the source system and certainly in an important implementation, uh, you would connect this our system.
Stephen Ibach: You could bring all of the data over if you chose to, and then we can schedule incremental update against the source system. So the difference here is we're not impacting the source system in any data virtualization way. We don't need to go back to the source and we, we acquire the data and persistent on our platform, and that enables us to not impact the source system and only run scheduled, incremental updates after the full data load.
Stephen Ibach: If that makes sense. So we're not putting any burden on the source system and obviously can align on that schedule appropriately. Mark, go ahead. Sorry. I'll reply with what hasn't said, because I was part of this proof of concept before AXA. So the first clue we've done it for 20 years of data, we've not for sure on a Friday.
Stephen Ibach: It took us one hour. This work, the only time you have impacting really impacting the actual with only the access. So don't expect a lot of heavy kind of a load on the resources after this. As you said, we can do the incremental route and as different illustrating you can do the incremental loop every day, every week.
Stephen Ibach: It depends on the frequency of the updates to take maybe a few seconds. So don't expect any outage on the actual, uh, data sources that are operational. Terrific.
Stephen Ibach: Any other questions? It's our pleasure. Thank you. Say very grateful for your questions, both from you and a walkout. Thank you so much for your questions today. We appreciate it.
Stephen Ibach: Well with that, mark, I think we're going to close out. Um, I certainly hope that we'll be talking to more, uh, customers, uh, today and, uh, and help them beat the data delay. So, mark, thank you so much for joining us. And again, I know you're on. Thank you so much again. Uh, so very grateful. my friend. Thank you.
Stephen Ibach: Thank you so much.
Hosted by:
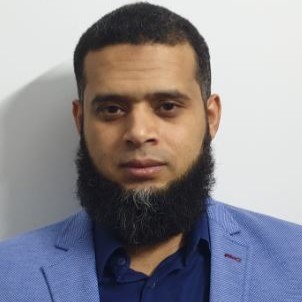
Hassan Abdulrahman
IT Senior Architect Manager
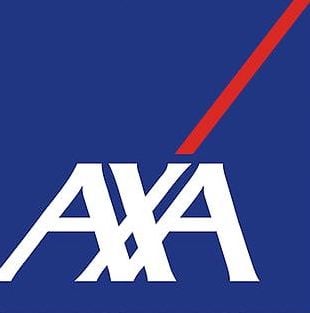
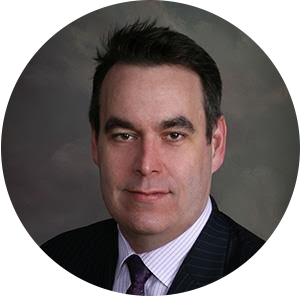
Stephen Ibach
VP of Financial Services Solutions
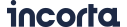
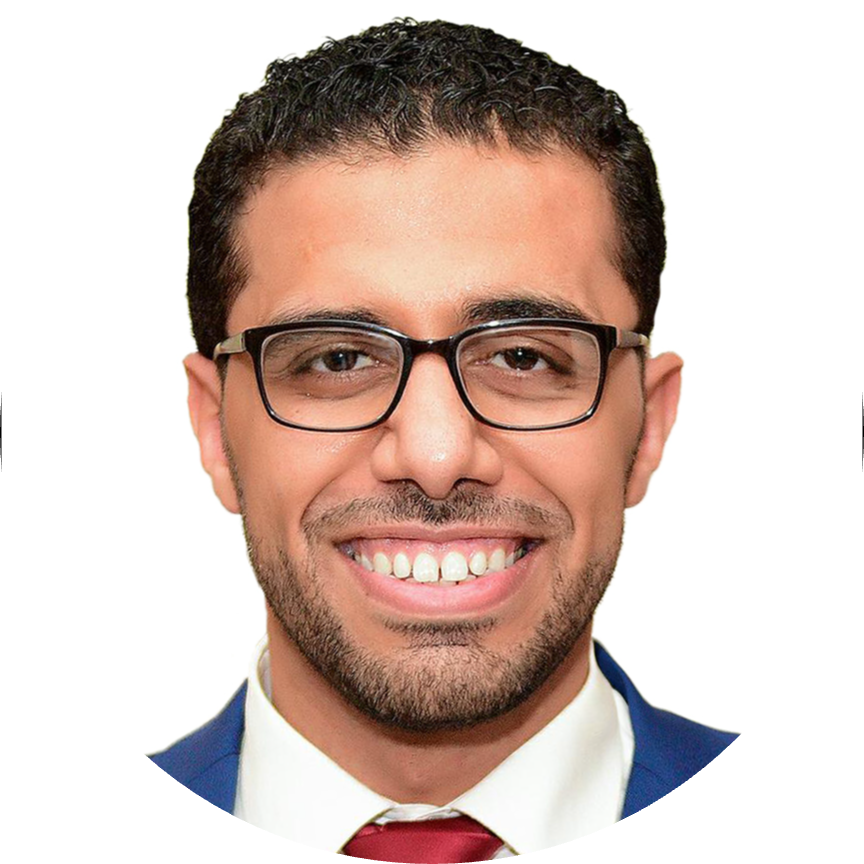
Mark Agaiby
Senior Sales Engineer
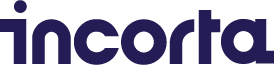