Can Data Mesh Help Fix Your Data Mess?
Unpacking the traditional architecture of data warehouse and data lakes
Do you ever have the feeling that there might be a better way to manage and utilize data in your organization?
The tools and strategies evolve, but at times still struggle to keep up with the growing demands of AI, data science, self-service BI, and enterprise reporting.
Incorta + PMsquare examine and discuss insights into the latest industry trends, and practical steps to advance the way your organization leverages analytical data.
In this webinar, we:
- Review common strategies under a microscope to see what's working and what needs to change.
- Discuss the buzz-worthy "Data Mesh" architecture: Is it an ideal only attainable by Silicon Valley tech firms, or is it a paradigm shift that may change the landscape of data and analytics?
- Explore how Incorta is naturally and uniquely aligned with many of the tenets of Data Mesh.
Transcript:
Margaret Guarino: Hi everyone, welcome to our webinar today, "Can Data Mesh Help Fix Your Data Mess?" My name is Margaret Guarino and I'm Incorta's senior director of partner marketing. I'll be your moderator today.
Margaret Guarino: Before we get started, I wanted to do a bit of housekeeping we will be recording this webinar recording will be shared with all of you, following the conclusion of today's session.
Margaret Guarino: We will be taking questions at the end of the presentation, however, we encourage you all to submit your questions throughout the presentation in the Q amp a box.
Margaret Guarino: We will do our best to address them all, at the end, but for any question not address the speakers will follow up with the individual directly i'd like to introduce our speakers today we have with us Mike dubois great cool Angelo and Cameron over over.
Margaret Guarino: Mike has been helping organization uncover insights in their data for about 17 years with a special focus on data architecture platform configuration and data visualization.
Margaret Guarino: He is a partner services director and solutions architect at PM square Craig joins us from PM square as well, he has been in the analytics games, since the turn of the century.
Margaret Guarino: Who remembers learning rpg and cobalt at school, sadly, he does those early reporting languages sparked an interest in him for reporting and decision support, which resulted in his 20 years or so, career of working with analytics products in various roles.
Margaret Guarino: Cameron is the senior director in technical product marketing at incognito with over three decades of varied experience.
Margaret Guarino: In in around Silicon Valley, including 20 plus years at Oracle he's seen it all he's designed coded architected soul demonstrated and explain how to use data and technology to solve business problems for some of the largest organizations.
Margaret Guarino: Across multiple industries, without further ado i'll hand it over to Craig to kick us off.
Craig Colangelo: Thanks Margaret and good morning or afternoon I guess early afternoon for a bunch of you.
Craig Colangelo: just wanted to generally tell you the agenda here so i'll spend about 10 minutes talking about the history and shortcomings and opportunity here mike's going to jump in and talk about.
Craig Colangelo: All about data mesh in depth for about 35 minutes or so and then camera is going to pop in at the end and tell us a little bit more give us some insight into how in quarter fits into the world potentially of data mesh is going to do some DEMO as well.
Craig Colangelo: And at the end, like Margaret said we're gonna have some Q amp a.
Craig Colangelo: So.
Craig Colangelo: How can it fix your data mess we don't really know to be honest with you right so.
Craig Colangelo: We don't know if data mesh can can actually fix your data mess right it's it's relatively new concept and it's not.
Craig Colangelo: Some exacting architecture right you're not going to find a how to book on data mesh quite yet it's more like design an organizational ideas around a new data management strategy where conventional analytic solutions are just nodes on the map.
Craig Colangelo: But the main driving ideas here behind data mesh are to treat domain focused data products is first class concerns, and then the tooling and the pipelines as really secondary concerns.
Craig Colangelo: Which is pretty different than you know, the way that we're used to rationalizing our analytic worlds and environments right and approaches.
Craig Colangelo: So what it does, is it shifts the current approach from more of a centralized one to a really a distributed ecosystem.
Craig Colangelo: of data products and that's kind of core to data mesh in and of itself is the idea of a data product and all these data products play nicely together, and you know, create effectively a match.
Craig Colangelo: So data match is still very, very young, but you know we think it's going to gain traction over the upcoming years and we're certainly not experts, yet, but we're really intrigued by the.
Craig Colangelo: potential for new thinking and potential paradigm shift here that could yield you know better data management practices, especially for those larger practices experiencing.
Craig Colangelo: Problems scaling or complexity issues and, of course, our hope with this webinar is that maybe it'll help your organization to.
Craig Colangelo: So why is an analytic data management working perfectly for many of us now, it feels like most of our analytic data management work is going all right we're you know we're getting by but generally.
Craig Colangelo: A lot of the work we analytics practitioners wind up doing on a day to day basis isn't quite business transformative right.
Craig Colangelo: Many of us are operating under the constraints of yesteryear there's been a ton of technical, technological evolutions right since the constraints that in men and kimball had to work under.
Craig Colangelo: So, think of big data tech think of ubiquitous cheap and easy cloud everywhere real actual practical data virtualization Ai infused everything.
Craig Colangelo: These tech advancements alone demand a reassessment of how we as analytics practitioners best provide data to consumers.
Craig Colangelo: We have so many more arrows in our quiver now just from a technology perspective.
Craig Colangelo: So this isn't to say that the conventional data warehouses aren't enough they're absolutely foundational and are definitely enough in some circumstances.
Craig Colangelo: But there's lots of new ideas and new tech that we can leverage to do more, better, faster.
Craig Colangelo: So maybe it's a good time to reassess our current approach to analytic data and seize opportunities to evolve a bit because it's been a while.
Craig Colangelo: So first let's quickly talk about the history and, most importantly, the kind of the realities of data warehousing to help put this all in context so data warehouses emerged around the early 90s.
Craig Colangelo: based primarily off of the works of imminent kimball and they've definitely been the bedrock of bi for decades.
Craig Colangelo: Now, keep in mind that back in the early 90s memory and disk were you know really expensive and processors were slow.
Craig Colangelo: You know anybody who's been on Twitter is seeing that there's images from the 1950s of those one ton IBM machines with you know disk drives that only stored like five megs of data right so.
Craig Colangelo: It wasn't quite that bad when inman and kimball revolutionized data warehousing a few decades ago.
Craig Colangelo: But you get the point, the pace of change in technology, as it relates to things that data warehousing can take advantage of has really been.
Craig Colangelo: very fast since then so traditionally our conventional data warehouses are built on these you know conventional monolithic often proprietary RD bms systems.
Craig Colangelo: And what they're really good at doing is transforming that third normal form operational data.
Craig Colangelo: into highly structured analytic ready form data right, and this is definitely schema on right you've got to land this data in the structures that are suited for querying.
Craig Colangelo: And they must be able to answer the questions that you expect them to be asked right, so that in and of itself sounds a little kind of naturally limiting the structures themselves are easy to understand you've got things like stars and cubes and dimensions and facts.
Craig Colangelo: But when it really happens that data warehouses kind of become these analytic systems of record and single version of the truth is the central theme historically here so they're graded answering known questions.
Craig Colangelo: And, of course, as we all know, conventional data warehouses depend on complex and costly atl and data pipelines in order to build them a lot of times, these are fragile a lot of times they're mostly back.
Craig Colangelo: And they're built to kind of last not necessarily to change so it's challenging to oftentimes for conventional data warehouses to adjust based on new tech or changing business conditions or even a crazy external factors right like a you know, a global pandemic, for example.
Craig Colangelo: So the key to building a data warehouse well is to generally capture all the requirements up front, and this means structuring you know.
Craig Colangelo: shaping refactoring of the data before the data is even use, which is kind of costly.
Craig Colangelo: So it's not really quick time to insight and because of all this there's invariably tension between the project scope.
Craig Colangelo: And the desire to put everything in order to know not miss anything right, this is the classic struggle between you know time and cost versus a more holistic sort of all inclusive solution.
Craig Colangelo: So, just a quick recap and data warehousing We often find that we generally model model data pretty rigidly it's generally very hard to build well and hard to maintain but it's very easy to query and use and understand.
Craig Colangelo: But then, you know, along comes a data lake house or i'm sorry the data lake right, so this new component comes along it's a new note in the architecture and it emerged, you know, a decade or so ago.
Craig Colangelo: it's kind of an antidote or a supplement really for conventional data warehousing problems, so the data lake is file based and it's built on object storage things like Amazon s3.
Craig Colangelo: And can use hdfs and mtp technology to store and extract data so memory and commodity servers are cheap and you can throw lots of data into it.
Craig Colangelo: So it takes data, as is in structured semi structured or unstructured formats and you can report directly off it from there, and an ideal scenario, a lot of times you need a middle tier but.
Craig Colangelo: that's another story right and, of course, because of all this, we generally just kind of stuff our lakes, full of whatever and they become these.
Craig Colangelo: Instead of systems of record for analytics they become these records of operational systems so rather than filling you know with carefully curated sets of measures and attributes.
Craig Colangelo: It tends to fill with any old data from any old system and further structures applied it only as needed right as not as a necessary condition to land, the data it's more like schema on read.
Craig Colangelo: So sometimes, these are certainly better suited for you know new analytics use cases because there's no need to guess or presuppose what there'll be used for exactly you just kind of land, the files and then you know they're queued up.
Craig Colangelo: But sometimes when you can answer any question broadly it's tough to answer specific questions precisely.
Craig Colangelo: And there's obviously a ton of noise right and the data lake world it's hard to get at the meaningful bits.
Craig Colangelo: If you need something you basically have to go fishing in the data lake amongst all of this, you know iot and unstructured and sensor and third normal form data.
Craig Colangelo: So it's hard to get out what you want oftentimes these data lakes turn into swamps and they're very hard to catalog understand and use which are kind of necessary precursors to getting value from it.
Craig Colangelo: So you know a lot of a lot of the reality of data lakes, is that it's a big ask for end users.
Craig Colangelo: So many data lake implementations fail or don't quite hit the mark due to these sorts of issues and self service outside of the data science persona is pretty difficult so to recap and data lakes oftentimes we store data, as is.
Craig Colangelo: We leave using and interpreting the data to the consumer on read, which is a bit of a challenge but they're very easy to build and load maybe too easy but challenging obviously to to query.
Craig Colangelo: Then over the past few years, along comes the data lake house which combines you know analytics structures, the data warehouse and the data lakes together as nodes on the lake house right with progressively better data and each zone each his own building upon the previous own.
Craig Colangelo: So lake houses solve a lot of conventional problems, you can access organized and raw data, you can have schema on read or write you can ask prepared or ad hoc questions so it's a great platform for lots of different use cases right bi planning data science, they all work really well.
Craig Colangelo: And you only do the work that you need to do and they're you know way more scalable than each you know the individual solutions on their own.
Craig Colangelo: So it's an elegant technical solution to kind of a lot of the analytic challenges that we've experienced and better suited for the various kind of distinct personas.
Craig Colangelo: So the lake House gets us closer to a better technological solution, but I think fundamental problem still exists, and this is where we kind of bring in some of the data data mesh stuff So what are the fundamental problems.
Craig Colangelo: we've observed at PM square that analytics and data management practices over time or either kind of centralized or distributed, depending on how recently, like the last multimillion dollar data gap is.
Craig Colangelo: So organizations seem to generally contract and pull into a more centralized model when something bad happens, or when data leaks, or you know their mistakes or log for J exploits or whatever.
Craig Colangelo: or they expand to more of a decentralized or distributed approach, when all is kind of the norm, or when rapid growth is needed.
Craig Colangelo: So we're you know another issue is that a fundamental problem here is that we're all kind of trying to do more with less these days, and sometimes that means fewer human resources.
Craig Colangelo: And, as it relates to data engineering, you know too few people have really excellent data engineering skills.
Craig Colangelo: and inevitably in the analytics world these data engineers are often too distant from operational data and business context in order to you know, provide the best accumulation or presentation of data to the business users so data engineers.
Craig Colangelo: You know our other highly specialized practitioners, who know their their stats and they know their methods well but oftentimes not the data itself because again they're so far away from the source data that they utilize.
Craig Colangelo: So we have these varying degrees of technological solutions and often most they mostly correlate to kind of like these proxy business problems but.
Craig Colangelo: I would argue that the business problems and the business culture and the business factors themselves kind of need to be more at our Center of our very Center of thinking.
Craig Colangelo: So consider this legos first product way back when was wouldn't ducks Ikea started off by making pens and Dupont way back when long, long time ago started off by making gunpowder.
Craig Colangelo: Now, considering what those organizations do now, is it enough to presuppose that business conditions or current problems and then narrowly build to that.
Craig Colangelo: Probably not so maybe we need to change our perspective, even in the world of analytics which is kind of slow to change sometimes from technology first to more of a product and process first approach.
Craig Colangelo: And with that really think of the data our data as the valuable product that it is not just an asset but a product and then treat it as such, so with that Mike, would you please give us a little more info and tell us what data mesh is all about.
Mike DeGeus: Sure i'd be happy to thanks for setting things up Craig I think it helps a lot to have sort of context for this conversation.
Mike DeGeus: As there are various different levels to kind of how how we talk about data mission how we're going to talk about it today there is certain way that philosophical.
Mike DeGeus: elements to it, but we don't want to stay there, we also want to try to get a little more practical, pragmatic with it as well, so we will try to traverse both here but.
Mike DeGeus: So, in terms of how we actually define what it is there's a few different ways we can do so.
Mike DeGeus: at a very high level it's basically just a new approach to how to share access and manage analytical data within or across organizations.
Mike DeGeus: and in doing so we're borrowing ideas really prone to software development world.
Mike DeGeus: And it's very important I know Craig mentioned this, but I just want to kind of remind here that that this is all really knew.
Mike DeGeus: These ideas are still works in progress as Craig said we don't consider ourselves as experts and data mash by any stretch and, in fact, if someone claims to be an expert in data mesh I would.
Mike DeGeus: approach that a little bit cautiously because there's no one out there really that's just done a dozen data mesh implementations and so can therefore claim expertise.
Mike DeGeus: Really, the people who know the most are the ones with sort of the most questions.
Mike DeGeus: and trying to figure out sort of how this is all applied, but it is, it is a very exciting new approach we think and there's a lot that can be gleaned from it.
Mike DeGeus: So let's let's dive in a little bit deeper so here's here's a definition of what data meshes and i'll just let you kind of marinate in that i'm not going to read it off here, but you know it's a little bit of a mouthful, but I think it is helpful and kind of summing things up.
Mike DeGeus: So a couple things to know about this one, it was invented by someone named show mock the Ghani and her first article was published just in in May 2019 so again that kind of speaks to how recent have an idea, this is, and that was just a first sort of.
Mike DeGeus: proposal of the sort of high level overview of these ideas.
Mike DeGeus: So shoebox thoughts and conclusions were definitely influenced by our time helping larger companies with their distribute operational systems and overall she's she's just a big proponent of decentralization.
Mike DeGeus: didn't mesh really does begin with the change in philosophy or culture of an organization, but there is also a prescription of key functionality so it's not philosophical only it does kind of get into how you actually implement some of the outworking out workings of that philosophy.
Mike DeGeus: it's also good to be aware that this isn't associated with a particular vendor.
Mike DeGeus: Definitely some vendors will kind of mentioned it, these days as it's gained some notoriety but this isn't a vendor philosophy and in fact sort of at its core it's it's agnostic to any particular tool or tech stack.
Mike DeGeus: We think it's it's easiest to understand by looking at the core principles of data mesh and we're going to look at that in just a second here.
Mike DeGeus: Well, first it's a little bit more about the Origination of the idea so Jim I spent decades in Dev OPS and distributed systems and she saw how practices.
Mike DeGeus: And those Dev OPS teams and operational systems could really benefit the world of analytics.
Mike DeGeus: And so, she adapts these ideas from the software development world things like microservices and an API API revolution, she tries to apply them analytical space.
Mike DeGeus: And so, she kind of lays out the back there's these two traditional planes I think these I will resonate with most people on this call there's the operational plane there's the analytical plane.
Mike DeGeus: And then the data between these two is connected through hcl right extract transform load thing we're all very familiar with, and so the operational plane has.
Mike DeGeus: not always for a very long time utilize domain driven design concepts domain driven design basically means designing software in a way that aligns as closely to the business as as possible.
Mike DeGeus: The analytical plane has traditionally been very much distinctly downstream from operational systems, so the data flows into a central repository rather than domains hosting and serving that data and it's sort of disconnected from the business domain.
Mike DeGeus: architects of this analytical plane, they tend to know their tools well but they don't necessarily know that actual data so while or the meaning or the business context behind that data.
Mike DeGeus: And so it data matches seeking to do is to bring some of those domain driven design concepts from the analytical plane.
Mike DeGeus: From sorry from operational plan into the analytical plane, so that the benefits from the operational round can be realized in the political realm you things like responding gracefully to change the standing agility in the face of growth being able to really scale as needed.
Mike DeGeus: And so there's there's some fundamental shifts really that are associated with this change around how we manage us and own analytical data so organizationally there's really a shift from centralized ownership of data by specialists who run the data platform.
Mike DeGeus: To instead of decentralized and ownership model that pushes ownership and accountability of the data back to the business domains, where the data is produced from where it's used.
Mike DeGeus: architecturally there's a shift from collecting data in monolithic data warehouses and likes to instead connecting data through a distributed mash of data products through some standardized protocols.
Mike DeGeus: I technologically there's a shift from technology solutions that treat data as really a byproduct of running pipeline code to instead solutions that treat data and Code as a sort of one combined autonomous unit.
Mike DeGeus: operationally we're shifting data governance from just a purely top down centralized model with human interventions to instead more of a federated model that has these will call computational policies that are embedded sort of within at all.
Mike DeGeus: Principally it's just our value system from data as an asset to be collected to instead data as a product that really should serve to the lights.
Mike DeGeus: The internal and external data consumers.
Mike DeGeus: And then finally infrastructure really it shifts from these two sets of fragmented infrastructure services where one is serving that that you know application operational systems.
Mike DeGeus: than the others for analytical to instead integrating those two were the same set of infrastructure concert both realms.
Mike DeGeus: Okay, so let's let's talk about how data mesh proposes to accomplish these shifts and here's where we're going to start to dive into the four main principles or pillars of data mesh.
Mike DeGeus: You know today we're really just scratched the surface on each of these over the next few slides there's there's a lot more that can be clean by by additional kind of diving into these concepts.
Mike DeGeus: So the first principle is domain ownership through domain oriented decentralized data ownership and architecture.
Mike DeGeus: The second is data as a product shared directly with data users of all personas analysts data scientists external customers and other domains.
Mike DeGeus: The third principle is self service self service sorry self serve data platform through a new generation of self serve data platform services that empower domains cross functional teams to share data.
Mike DeGeus: And finally, the fourth principle is about federated computational governance with embedded centralized governance policies, but with federated decision making and accountability at the domain level.
Mike DeGeus: Alright, so now we have those those definitions of those out of the way let's dive into this a little bit more what each one of these is so domain oriented decentralized data ownership, in addition to being a mouthful here.
Mike DeGeus: kind of what is this so first of all, we got understand the terms within the larger term so what's the domain in the world of data mash really it's just a an area or a function or a slice of the business.
Mike DeGeus: From the guidelines of domain driven design it's a sphere of knowledge influencer activity okay so to find the delineation here, because this can be a little harder in practice, of course, then in definition.
Mike DeGeus: data shows that we should try to just look for the schemes of of organizational units kind of how the business is already functioning.
Mike DeGeus: So instead of how existing data architectures tend to be portion by sort of a market or a pipeline or the underlying tech solutions.
Mike DeGeus: These sorts of approaches are kind of at odds with the organizational structure of modern businesses and a lot of ways that they're really set up to centralize complexity and cost.
Mike DeGeus: So, instead, we have this this concept of being domain oriented decentralized and so Jim I use this example in our writings of a fictional company called Daf which is like a global music streaming company.
Mike DeGeus: And you see in the illustration there those those large ovals are essentially the domains, and you see sort of the business cases that connect to the various domains that she identified.
Mike DeGeus: Now analytical domains can kind of fall into one of three different what we call fuzzy archetypes and understanding them can be sort of helpful from an implementation standpoint.
Mike DeGeus: Although it's not isn't something that like users in a data match need to understand.
Mike DeGeus: So the three archetypes are source align data and that's really critical analytical data that reflects the business facts generated by the operational systems very directly is also call it a native data products.
Mike DeGeus: And while the source system generated data and analytical data off coming off of it are considered separate they are very tightly integrated and there are owned by the same domain team.
Mike DeGeus: The second archetype is aggregate domain data that's really just kind of a roll up of analytical data from multiple upstream domains.
Mike DeGeus: And then the final one is consumer allied domain data and that's analytical data that's actually been transformed to fit the needs of some specific use case or use cases.
Mike DeGeus: Now here's something that's sort of controversial and Craig alluded to it earlier and our data mesh we're actually not going after the single version of the truth, which is.
Mike DeGeus: Maybe a barrier, we have to get over if you've been in the data world for a while this is sort of been the thing that everyone talks about for the last couple of decades, at least, is how we have to get have a single version of the truth.
Mike DeGeus: But instead of that approach instead on our data mesh replacing the most relevant to a particular use case particular need for particular domain.
Mike DeGeus: The problem with single version of the truth is that it doesn't necessarily reflect reality of the business and it's costly to come up with this and it really impede scaling.
Mike DeGeus: So now their data mesh we're we're still looking to address we don't want to have you know multiple copies of stale beta.
Mike DeGeus: And you know untrustworthy data spot all over the place that's still a problem so some of the some of the principles behind single version of the truth, of course, are still there are still valuable, but that that one gold copy single version of the truth isn't really the goal here anymore.
Mike DeGeus: So there's a British status statistician named George box in the mid 70s, he made this observation that all models really wrong, but some are useful.
Mike DeGeus: So models always fall short of the complexities of reality right but doesn't mean they can't be useful and so applying that here to figuring out our domains.
Mike DeGeus: is important because you got to start somewhere and trying to figure out exactly how to flip it organization domains you kind of sit sit right there at that point forever ever make any progress in terms of implementation.
Mike DeGeus: And so we kind of don't want to let perfect be the enemy of the good, here we can sort of jump in.
Mike DeGeus: All right, so let's talk now about the roles that actually exist within this decentralization or within a domain.
Mike DeGeus: So traditionally and analytics roles have been functionally divided, but this devops movement of recent years which.
Mike DeGeus: everyone's familiar with, has led to more and more cross functional teams and you know the value of that has been realized in many ways customer obsessed product development has brought the design and product that are closer to the developers.
Mike DeGeus: So in the world of data mash cross functional domain teams.
Mike DeGeus: Their main roles consistent these these two roles, so the one is a data product owner.
Mike DeGeus: And the data product owner is accountable for the success of domains data products and a few ways so delivering value satisfying and growing data users, maintaining the life cycle of data products.
Mike DeGeus: They also responsible for the vision roadmap for data products they're concerned with customer satisfaction for trying to measure and improve data quality and defining success criteria and kpis for products and.
Mike DeGeus: at its core domain data product owners, they have to have a deep understanding of who the data users are how they use the data.
Mike DeGeus: And what the native methods are that they use to access that data, how they consume it.
Mike DeGeus: So just think about like the difference between a data analyst, for instance versus a data scientist data scientist is likely.
Mike DeGeus: Does a bit of a generalization but is more likely to probably access things through code Reza analysts might be more comfortable through some sort of you know self service graphical interface, so those things have to be all taken into consideration.
Mike DeGeus: And the second role is the data product developer, and this data product developer, you know this is there's some similarities to the atl developer, perhaps, of the past.
Mike DeGeus: So this person is responsible for developing serving and maintaining data products, as long as they're live and being used so, whereas the EPL developer was kind of you know they have this expertise and data engineering tooling.
Mike DeGeus: But they didn't necessarily know a lot about the software development world those two things hadn't existed separately.
Mike DeGeus: Whereas the data product development or data mash has understanding of both of those things, so they know they know their their data tooling then they also can bring to bear some software engineering best practices things like continuous delivery automated testing, etc.
Mike DeGeus: Okay, so so data as a product so we're going to start real sort of philosophical thoughts topic here first and then we'll we'll dive in.
Mike DeGeus: So if we're going to treat treat data as a product, first of all, again we got to define our terms what what is a product actually.
Mike DeGeus: Well, sometimes we might think in our heads, you know just as consumers as a product is something we can buy right.
Mike DeGeus: But conceptually products are sort of intersection between users Business and Technology, so a product can be defined as the result of a process between users and a business with technology acting as the bridge between the two.
Mike DeGeus: So, then, we need to employ something that's called product thinking.
Mike DeGeus: And so we have this problem space, which is what the users need between the users on the product, we have a solution space between the product and the business and that's what businesses can give.
Mike DeGeus: And then product thinking is it's the journey really from the problem space of the users to the solution space of the business or another way to say that simply it's a singular focus on solving problems with the goal of reducing that gap between users in the business.
Mike DeGeus: Okay, so it's kind of a fancy way, maybe to say something that seems very simple.
Mike DeGeus: But the reality is and why I think it's worth talking about here is because a lot of times this isn't actually what happens in the real world.
Mike DeGeus: A lot of times businesses, they start with solutions they build something that they think is cool and then they say hey hey users, consumers.
Mike DeGeus: We built this thing hey how about you use it for this and it's a little bit backwards.
Mike DeGeus: Often oftentimes you see a lot to just sort of thinking and features or or selling features well hey our software does this or a widget does this thing.
Mike DeGeus: As opposed to that you know the value it provides and really solving a problem and so they're Actually, I think it is useful to kind of keep this in mind and keep this product thinking in mind, even though it's not complicated.
Mike DeGeus: In the real world, we can kind of get off track.
Mike DeGeus: So let's apply this to data with data as a product analyst data scientists data engineers and clients are all potential your customers.
Mike DeGeus: And so domain data product owners need to ensure that they understand customer problems deeply thoroughly before delivering solutions and really their aim with these solutions should be to delight these customers, whoever they are.
Mike DeGeus: Okay, so that that is is the philosophical approach to a data as a product, but again data mash it gets into the practical specifying some capabilities that should be part of a system that treats data as a product.
Mike DeGeus: So here's what those are so first of all discoverable and understandable, there should be a centralized data catalog where domain data products are registered, preferably in an automated way when they spin up.
Mike DeGeus: But at the end of the day, they need to be discoverable and be able to be understood by users.
Mike DeGeus: intentionally designed discover ability features and continuous sharing of things like source of origin top use cases applications enable timeliness quality, etc.
Mike DeGeus: doesn't need to be addressable this just means some sort of standard unique address for each data product whether you're accurate, whether it's being accessed programmatically or manually.
Mike DeGeus: It can be continuous change here's the mash grows, but should be some sort of continuity here for users.
Mike DeGeus: A trustworthy and truthful right, so no one's gonna use it if it can't trust it right.
Mike DeGeus: Even if there's, not a single version of the truth, the relevant versions that we're looking at must be trustworthy.
Mike DeGeus: So we want to create some guarantees around this like a cellos service level objectives for truthfulness how closely, it reflects the reality of the business events.
Mike DeGeus: This might contain agreements around things like data lineage so how How did the data get from where it started to where it is now.
Mike DeGeus: The interval of change, you know how often that data is updated the timeliness the skew between the time that the business facts actually occurred and when it's available to consumers.
Mike DeGeus: How the data is shaped like distribution range how much data and precision and accuracy, over time, so the degree of business truthfulness, as time passes.
Mike DeGeus: forth natively accessible.
Mike DeGeus: So many different personas are going to access this data.
Mike DeGeus: And therefore data product needs to make it possible for various data users to access to read it's data in their native motive access is kind of what we talked about a second ago help there's there's different personas you might have access to data very differently.
Mike DeGeus: interoperable and governed by global standards so.
Mike DeGeus: This enables joining to other data products and aggregating as you start to think about data mesh and you start to come up with objections, maybe this This to me when I first heard about this was my first.
Mike DeGeus: sort of Okay, so you have this distribution, how does it all fit together, because you know at times you got to pull data together from different domains.
Mike DeGeus: Answer business questions and So yes, that is critical to be considered as a lot of data mash it's already to do there's something called a global federated entity or a poly seem was maybe you needed.
Mike DeGeus: there's sort of like conform dimensions, they give us, they give us sort of standard entities across the organization that can be used to pull data together from different domains.
Mike DeGeus: And then, secure and governed by global access control so that's going to encompass a lot of different things things like sso single sign on.
Mike DeGeus: role based access control, this should be included with the code of security policies and governance encryption data retention, and then you know rules around regulations and agreements things like gdpr contractual agreements etc.
Mike DeGeus: Okay, so we're to the third pillar here self service data infrastructure as a platform.
Mike DeGeus: So what this is saying is that there's a platform that's built and maintained by a dedicated platform team.
Mike DeGeus: And they're responsible for all consumer experiences and infrastructure supervision, so this is kind of interesting because this is almost centralizing something that.
Mike DeGeus: I can at times be more distributed in organization again depends on the organization.
Mike DeGeus: Sometimes, this was centralized already, but just in a implement it implemented in a different manner than we talked about their data match the times it gets distributed, like everybody has their own tools.
Mike DeGeus: and have spread all over the organization, this is under data metrics that we need this, we should be standardizing that submit.
Mike DeGeus: So the keys to building include these sorts of guidelines, so the platform should hide the underlying complexity there shouldn't be any domain specific concepts or business logic that the platform is domain agnostic.
Mike DeGeus: it's designed really for like the generalist majority of the organization, rather than kind of specific use cases.
Mike DeGeus: It favors decentralized technology and it favors open conventions and tries to steer away from proprietary languages.
Mike DeGeus: And so, when it's built, well, it provides things like encryption for data at rest and motion data product burgeoning.
Mike DeGeus: The data product schema automation for things like data ingestion registering the product with the catalog and then management of these you know autonomous different.
Mike DeGeus: Data products exist from different domains and really the recommendation for all this is cloud infrastructure.
Mike DeGeus: This allows for you know the on demand spin up of this infrastructure, you can basically.
Mike DeGeus: Say ahead of time here's our menu of infrastructure options that can be used our organization.
Mike DeGeus: And whenever they need to be provisioned they're sort of ready to go in the cloud and in a few clicks those can be spun up, they can be made available to a domain, you can then get to work and creating data product.
Mike DeGeus: Alright federated computational governance so governance is the mechanism that assures that the mash of these independent data products when they all come together as a whole it's secure trusted and delivers value through all this inner connection.
Mike DeGeus: We have to find a balance here at maintaining domain autonomy while still enforcing some global policy standards which is you know potentially sort of a tricky tightrope to walk.
Mike DeGeus: But the animation is all about federation and domain autonomy, but you still have to have these standards and global policies they ought to follow.
Mike DeGeus: In order to be a member of the mash and order system can can be functional and so data product owners are going to contribute to the definition of these policies that are going to govern all the other products on the on the mash they're going to have a voice in that.
Mike DeGeus: So, ideally, the governance function is sort of invisible it's out of the way it's automated it's extracted by the platform and it gets embedded in each of these data products and apply at the right moment.
Mike DeGeus: So this gets done via computational governance so really that means policies are embedded in each data product as code.
Mike DeGeus: And this is the mechanism of checks and balances for local decisions against the global agreed upon normal the mash.
Mike DeGeus: And I know that's sort of like maybe abstract, but if you think about it, you know if you're in the USA, we have a federation here right, so you.
Mike DeGeus: there's always this balance, this tightrope between the federal government, and then the state, so you know in each state, you can get an idea driver's license right it's lost to conform to some.
Mike DeGeus: global standards set by the Federal Government and so that's sort of what we're talking about here, the implementation of it, of course, it can be tricky but the concept itself isn't terribly complicated.
Mike DeGeus: Alright, so with all these principles and pillars, we get some new language, I think this new language can actually be helpful and provoking organizational change.
Mike DeGeus: So instead of ingesting massive amounts of data and said we focused on serving the right data and delighting our consumers.
Mike DeGeus: Instead of costly conventional atl we focus on delivering our enabling discovery and we use that data products like different personas for different use cases instead of flowing data around be essentially the tail pipelines instead we focus on publishing events as streams.
Mike DeGeus: We didn't dive into that that fear, but you know that's another concept related data mesh and instead of a centralized data platform team and a bottleneck be focused on creating a distributed ecosystem of accessible data products.
Mike DeGeus: Okay So where do I start with all this, what can I just go out and buy the software stack that's going to do this for me.
Mike DeGeus: Okay there isn't it no such thing exists, this isn't a product you buy off the shelf, as we mentioned data meshes really product agnostic tooling as a second class concern.
Mike DeGeus: You definitely will hear vendors use data mesh and marketing and but nothing wrong with that because they certainly can be enablers they can be nodes on the mash.
Mike DeGeus: But it really is more about an approach, a philosophy that has to be coupled with a structural and organizational shifts.
Mike DeGeus: So before you just jump in headfirst into data mesh I think it's really worth considering if it's right for you.
Mike DeGeus: Joe mock uses this term socio technological described data mesh, which means that it has a lot to do with people and work structures, in addition to just technology.
Mike DeGeus: That were left it recognizes the relationship between people and technical solutions in organizations, so I really have to ask are people ready for these these types of shifts in terms of teams and accountability is that really like the DNA of our organization.
Mike DeGeus: We think it's fair to say that organizations with complex analytical landscapes, or those that currently have scaling issues due to bottlenecks.
Mike DeGeus: are probably the ones that would benefit most from Beit shemesh principles these types of organizations, they usually already have mature devops practices.
Mike DeGeus: Who sort of forbes the way I can help provide advisement on the data mess journey.
Mike DeGeus: Those organizations also might have some formal change management skills and my change management I don't mean like.
Mike DeGeus: hey we're going to add two new columns to this report, but any change management like change in an organization like that affecting the people how you bring people along when things are changing organizationally.
Mike DeGeus: So typically these are large organizations that kind of have these prerequisites in place and data much as a whole, might be overkill for for many.
Mike DeGeus: Well, regardless, everyone can really benefit from recognizing the limits of current approaches and the potential value of some of these new ways of approaching data management.
Mike DeGeus: So you know whether your organization is ideal candidate for data mesh right now.
Mike DeGeus: The principles and ideas themselves, we think are really sound and all can benefit from understanding these to some degree in in whatever form.
Mike DeGeus: You know, for instance, data as a product thinking of thinking of data as a product, we think it's very useful anyone should probably be picky about data, a little more that way versus just hey this is some ass out, we want to turn out as much of it as possible.
Mike DeGeus: So what's our next steps, can you take well check out your marks book just came out in the last couple months it's really recent as we keep saying.
Mike DeGeus: There were previously there were some articles online and people trying to kind of figure it out from there, there is actually a book on it now that just came out so that's worth reading lot of good info there.
Mike DeGeus: dig deeper into the principles for better understanding about how you can you know gauge applicability, for your particular organization and then learn from the early innovators journeys, you know if you talk about the the adopter curve here.
Mike DeGeus: You know, really, and, at best, the early adopter stage it's not the innovators stage.
Mike DeGeus: So you really you have to kind of be brave, you know that the innovators are the or the brave the early adopters are eager so there may be somewhere in between there so just think about.
Mike DeGeus: If you want to jump in now, or, if you want to just start learning about it, and maybe once things are a little bit more well established a jump in later, but there are organizations that have.
Mike DeGeus: embarked on this journey already and i've done a lot of work in this area, so you want to learn lessons from from their mistakes for sure, and not make those on your own.
Mike DeGeus: Alright, so with that i'm going to turn it over to Cameron and he's going to share a little bit about how a quarter might fit into this picture.
Cameron O'Rourke: awesome thanks Mike so great, so what i'd like to do is sort of go from you know the principles of data mash and get into I guess some practical technology and i'd like to.
Cameron O'Rourke: You know use in court, I just happen to work it in quarter use that as a vehicle, I guess, just so show some ways that you might implement data mash.
Cameron O'Rourke: Right, so you know, looking at the four principles of data mash and as we think about you know, the first principle right i'm going to stop my video just for bandwidth reasons.
Cameron O'Rourke: You know domain oriented ownership right, it becomes really obvious that we can't know ahead of time right how different.
Cameron O'Rourke: Business domains right, that was the first principle might use all the data all the questions that are waiting to be asked to the data and what direction the analytical exploration will go right, so one idea.
Cameron O'Rourke: is to collect and make available as much of our data as possible to give the business units flexibility, you know, and in doing their analysis so by way of example, I mean quarter, this is our ui.
Cameron O'Rourke: It has a pretty unique approach to analytics and we'll get more into that.
Cameron O'Rourke: But i'm going to just start at the at the schema level which catalogs the data that we're collecting from different sources right and.
Cameron O'Rourke: I want to take a look at this one down here this schema shows data actually coming from an oracle E business suite the ap module right and.
Cameron O'Rourke: What you're seeing here all the tables and relationships that were.
Cameron O'Rourke: That were looking at you know from that system So the first thing to note is when we're working in this system and in Canada, we capture and maintain all the original detailed data from the business application.
Cameron O'Rourke: And this is important because it means that, instead of throwing away a lot of the data that you would in an email process or building a dimensional model.
Cameron O'Rourke: All the data is available right, so this is rather unique in the analytics world, at least so far.
Cameron O'Rourke: So the different business units can leverage this data for different different purposes right and build a different views of the data very quickly so let's imagine i'm a business user maybe I mean the purchasing department.
Cameron O'Rourke: And you know because of that I know my data pretty well.
Cameron O'Rourke: This is all familiar to me and.
Cameron O'Rourke: And I can quickly build up a query you know from all these multiple tables right so, for example, maybe I just want to do an invoice report, I can just come down here Okay, here we go so maybe I want invoice number that makes sense, maybe I want the invoice date.
Cameron O'Rourke: Perhaps the invoice amount right and then maybe bring the vendors into it, rather than searching i'll just look for vendor.
Cameron O'Rourke: here's my suppliers table that makes sense, upon the vendor name and about the type look up code.
Cameron O'Rourke: Right pretty easy right, and you know what you'll notice is this gives business users, a ton of flexibility, I can literally go anywhere right, I want, using all of the data from my system, but the key is that it also gives them tremendous agility to try out different ideas and.
Cameron O'Rourke: do so without having to wait for a new data set from it, or to create a new to detail pipeline it's just load the data and go right so.
Cameron O'Rourke: Leading into the second principle of data mash data as a product that would include packaging up this data packaging up these ideas into something that can be consumed by other users right.
Cameron O'Rourke: And as an example of that that query that I just put together here and analyzer could be saved as a view with metadata for other users so i'll just go in and do that i'll choose you know, an existing.
Cameron O'Rourke: Business schema which holds business views and i'll give this the name, perhaps it's just open invoices something like that and i'll save that guy and.
Cameron O'Rourke: What happens is then we start to build up this view and it's really easy then for users to come in and just reuse this right in their in their own queries and it makes makes a lot of sense for them right.
Cameron O'Rourke: And I can keep going I can follow an iterative approach with this if i'm a user so, for example, someone here created this table of payments right, I could come in and.
Cameron O'Rourke: I could look at this and see if it's what I need and Edit this I don't need all these filters in this invoice numbers duplicated and just make a few changes here and I could save this guy is a business view.
Cameron O'Rourke: So i'll just choose the same view here and i'll save that, and you can see that i'm starting here once this gets saved don't hit the button, yes, that i'm starting to create you know, like a.
Cameron O'Rourke: almost like a data package for my users and i'm preserving the structural insights.
Cameron O'Rourke: That the users are creating through the through the dashboard and process, and I can continue to use this so, for example, you know, maybe I want to do an aggregate listing and.
Cameron O'Rourke: let's look at this by vendor name or group it by vendor name the amount of payments renaming maybe the total invoice amount and and I don't know how about.
Cameron O'Rourke: How about like this will use this one as account, so this is the count of invoices here.
Cameron O'Rourke: Just like that, so all my vendors everything we've invoice the amount of payments remaining in the invoice count that's a note the speed here right it didn't spend weeks, creating a data pipeline.
Cameron O'Rourke: You know just you know good and go and then I can also access these views that i've created using external tools so maybe i'm using tablo or maybe using power bi right.
Cameron O'Rourke: Now, I just have like a little simple query tool here i'm going to come in and i'll refresh this.
Cameron O'Rourke: The views that we create a career, because we just created a couple and here they are here's the new views that we just created let's click on that and run a quick sequel query I mean just that simple right.
Cameron O'Rourke: It is orders of magnitude faster, you know, then the traditional way of doing things with big big projects and etfs and all that trying to pre build the perfect dimensional model to meet.
Cameron O'Rourke: All the possible things that we can't you know anticipate right.
Cameron O'Rourke: In addition to all that another thing we can do is is package up just analytic content like what we've been doing into something we call blueprints or data Apps and.
Cameron O'Rourke: The cover different source applications like business flows and even individual modules right.
Cameron O'Rourke: And when you install one of these blueprints for one of these data sources, you get a framework and it includes you know the schemas that we've been looking at and some views, but it also includes.
Cameron O'Rourke: You know, some sample dashboards to get you up and running quickly so basically taking all the insights and everything that come from.
Cameron O'Rourke: You know the business domains, and the users of the application and packaging that up for other users to take advantage of right.
Cameron O'Rourke: So the third self service, so a third principle right is self service right and it's about making it simple for a person in the business domain, maybe they only have baseline technical skills that.
Cameron O'Rourke: They need to set up, you know not only the infrastructure but do everything from data acquisition to publishing dashboards right well.
Cameron O'Rourke: For for infrastructure, of course, we have you covered there it's a complete data and analytics platform it's very simple to create a new cluster just give it a name and a size and where you want that to happen.
Cameron O'Rourke: Right, you can do all your config configuration here add new blueprints if you wish.
Cameron O'Rourke: configure all your security your users and everything right from here, but in the application itself, of course.
Cameron O'Rourke: You can do everything users groups roles for the security, you can set up data sources, I can connect to just about anything and fill it in you know, a forum to connect to what I need.
Cameron O'Rourke: I have local data files, I have data destinations we've seen schemas in here already, so, for example, here's here's just another one, you know that we've set up.
Cameron O'Rourke: The business views that make it easier for casual users to get in here and understand what the state is and take advantage of it, you know all the way out to dashboards.
Cameron O'Rourke: Right and and all of this, this this this content, you know helps make it an easy all in one self service self service platform right.
Cameron O'Rourke: Now the fourth and final data mesh principle would be of course federated computational governance and you know this is really about a system that it's allowing for a wide consumption of data.
Cameron O'Rourke: On but with frameworks in place for data quality and security and one example, that would be.
Cameron O'Rourke: In court is able to have centralized security that can leverage the fact that we're actually analyzing detailed data to set up fine grained data protections right.
Cameron O'Rourke: So I mean here's a rather trivial example web, so all i've done here is just I limited the visibility of the salaries in this in this some HR report to.
Cameron O'Rourke: departments that have you know five less than five employees, we just don't let them see the salary and that's down at the table level, and you can't bypass that you know if you don't have privileges right.
Cameron O'Rourke: Other use cases would include mirroring the security rules that are present in the original applications and because of this more users can analyze more of the data, because now.
Cameron O'Rourke: That data can be protected inside the analytic system, you also need.
Cameron O'Rourke: In terms of governance good metadata about your about your data and about the usage, so this is all the metadata that encarta hangs on to.
Cameron O'Rourke: pop into a couple of examples, this is like a bunch of stats about data that's being loaded over time and all the details about.
Cameron O'Rourke: about that, and where it's coming from and the sizes and here's another one about data usage, I mean, I have only me in this system so it's not terribly busy but.
Cameron O'Rourke: You know, can see the dashboards that are being used and the query every single query that gets run in the system is logged somewhere, so you can really get a sense of how the data is being used.
Cameron O'Rourke: But even better like if I drill back into the schema and let's just take a look at this invoices table again I can look at data lineage rather easy so they have this column here, I can see.
Cameron O'Rourke: All the dependencies within the physical schemas whether it's a formula column or it's used in a join, I can see if it's used in any business views.
Cameron O'Rourke: And were in any dashboards that this column is used in so get full data lineage and be able to really understand all how the data is being used, you know by all the domains everybody that's taking advantage of this data.
Cameron O'Rourke: So this is a just a quick mapping, for you have the data mesh principles to various practical capabilities.
Cameron O'Rourke: And you know you can actually if you want to try this out, you can sign up for an inquiry trial on the website I.
Cameron O'Rourke: encourage it and you can try out these principles that we've been talking about in your own environment, maybe explore different way of thinking about data engineering, it is a bit of a paradigm shift.
Cameron O'Rourke: In data pipelines and giving data to your users very incrementally you know, without a lot of the cost and time or risk of a kind of traditional big data pipeline approaches.
Cameron O'Rourke: that's all for me, just a quick look at some technology back to Mike and Craig.
Craig Colangelo: Thanks camera that's awesome yeah that makes a whole lot of sense to me, I think I might sign up for a free trial, if you don't mind.
Craig Colangelo: Alright, so I mean i'm guessing we all have questions right there's nothing super exact exacting around data mesh yet and the how to write it's definitely in its infancy there's no like kimball data warehouse toolkit sort of equivalent book.
Craig Colangelo: Except for the one that Mike mentioned that Danny just put out so it's really a journey right we're lessons are learned kind of all along the way, so.
Craig Colangelo: Margaret I don't know if you've curated any questions there that we should try to tackle but looks like we got a couple minutes there to do that.
Margaret Guarino: yeah we have a few questions, I will try to get through two of them and then again if anyone has questions, please put them in the Q amp a box of the chat box and we'll follow up with you directly.
Margaret Guarino: So this question is for Mike which one of the data mesh four principles do you find most important to implement in current infrastructures.
Mike DeGeus: wrote there's, the most important but I, I do think, as I mentioned before, data as a product is a really good place to start.
Mike DeGeus: Because that that can be sort of a philosophy that can be socialized wrench organization and without changing organizationally kind of how how it struck how your structured or implementing any new technology, new tools, you can start thinking of data as a product, you can start approaching.
Mike DeGeus: You know approaching data from the standpoint of understanding what the user problems are trying to solve those problems and delight customers.
Mike DeGeus: And you know, even if one person over here is doing that someone else over here isn't yet you can get you can still reap benefits from that so in my opinion that's a good place to start.
Margaret Guarino: And then I think you kind of alluded to this when you were saying trying in fact practice, you know, read the book look at what other companies are doing is there a single resource that lists like what data platforms were database technologies and other sources, like connectors.
Margaret Guarino: might be already like ready to support data mesh that are out there, like is there a checklist of sorts that you know that that's available to people.
Craig Colangelo: Now, so in terms of like the technology right there's the ideal and then there's the practical so.
Craig Colangelo: I think JPMorgan chase has actually done almost maybe the most in the world of data mesh exploration, thus far, and I can tell you that their entire infrastructure was on aws cloud so.
Craig Colangelo: utilizing all of the different tools, you know glue and redshift and Jupiter and Athena and canisius and all those sorts of.
Craig Colangelo: You know components to pull it together right, ideally, I think you want eventually right in that ideal world, you know didn't mesh as a proponent of you know code and and.
Craig Colangelo: data and policy and pipelines all kind of is one autonomous unit kind of like a micro service but nobody's there yet right so in the meantime you've got all these different.
Craig Colangelo: You know nodes in our world that can be interconnected in the right ways like in court in order to pull it all together.
Margaret Guarino: We probably have time for one last question, I know I don't know if we'll have enough time to answer it so this one might be a follow on, but if you're using data as a product and your example.
Margaret Guarino: Allowing users to access in quarter via queries what type of infrastructure changes would you propose.
Margaret Guarino: And or limit.
Cameron O'Rourke: hmm that's a.
Cameron O'Rourke: Sure, I completely understand the question but I mean, I think I think the philosophy moves from you know, trying to pre anticipate.
Cameron O'Rourke: You know the questions that business users in different domains are going to try to ask right, so it used to be you go gather all your requirements, you know figure out what they were then you'd go try to engineer a data pipeline to fit that.
Cameron O'Rourke: ahead of time, with all the transformations and you know you're kind of willing smashing down and aggregating and reshaping the data.
Cameron O'Rourke: As you go, you know and you're losing a lot of that fidelity so you're cutting out like you know whole groups of.
Cameron O'Rourke: Your data scientists they're not going to want to look at that they're going to want to see the original data, you know and you're maybe cutting out other domains other groups that you know want a different kind of the data so it's a it's a bit of a.
Cameron O'Rourke: philosophy shift to.
Cameron O'Rourke: Thinking about putting up that.
Cameron O'Rourke: Mostly raw original data there and then letting the different groups that want to use it access it and then begin to interactively add structure and semantics and meaning to that data right kind of like I did in my DEMO and start to bit it was kind of a mini.
Cameron O'Rourke: You know compressed time frame, but that's actually what happens, you know and a lot of our customer sites they build up these.
Cameron O'Rourke: This this understanding of the data over time and there by doing so they're able to get results very quickly very incrementally you know and and start to get value out of the data, you know, without having to wait right yeah.
Craig Colangelo: guess what in quarter loves third normal form data.
Craig Colangelo: Right so.
Craig Colangelo: To me, what a great advantage, what a great starting point.
Margaret Guarino: Well, thank you all that's all the time we have for questions today don't forget to check out the link in the chat to get a free trial and quarter, if you have any other questions you can pop them in quick and we will follow up with you and thank you all for joining us today.
Craig Colangelo: Thank you.
Hosted by:
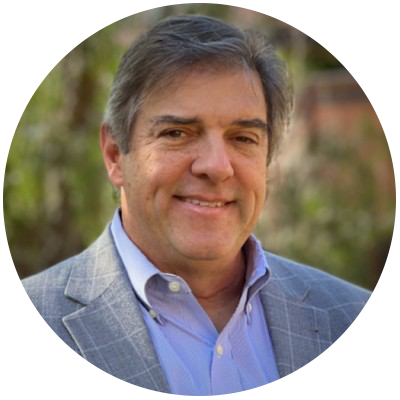
Cameron O'Rourke
Sr. Director, Technical Product Marketing
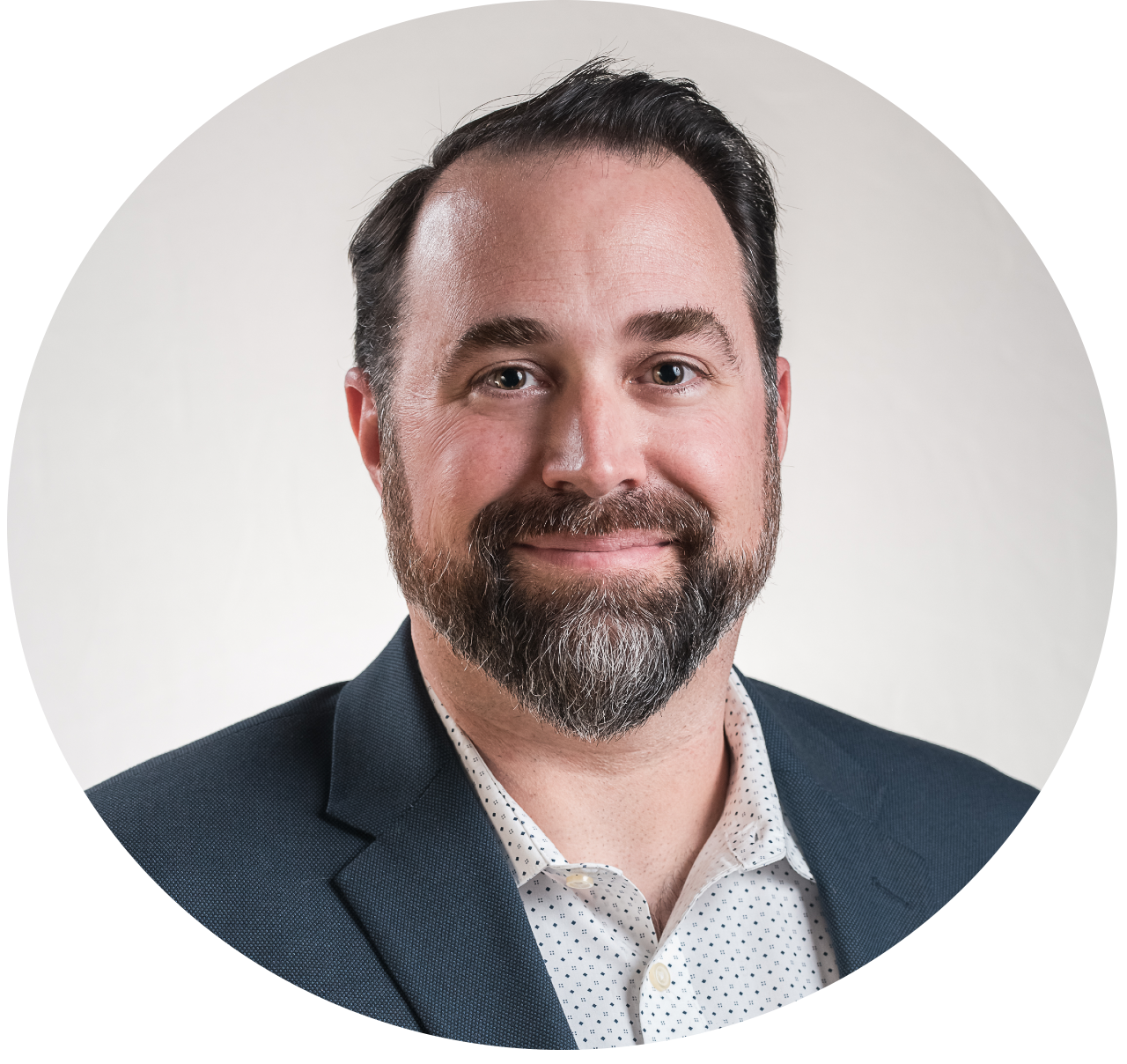
Craig Colangelo
Senior Solutions Architect
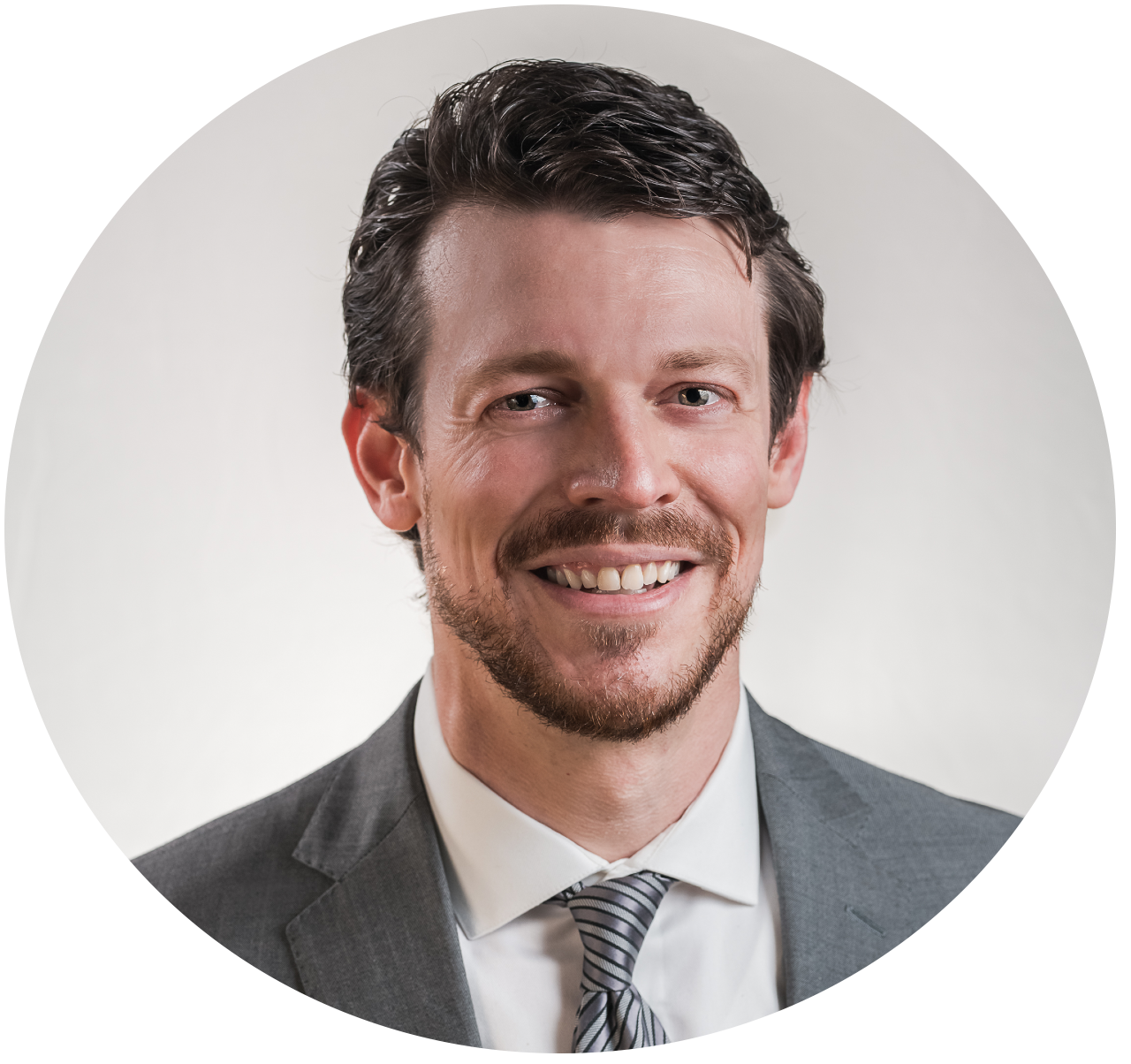
Mike DeGeus
VP of Operations