How to Implement a Virtual Star Schema: Use Virtual Star Schemas to Eliminate Complex Data Pipelines Forever
Virtual star schemas are quickly disrupting competing data architectures like data warehouses, data vaults and data virtualization. That’s because virtual star schemas let BI teams finally deliver high-performance, self-service analytics without needing to build and maintain expensive, time-consuming data pipelines to support physical star schemas.
Watch this webinar to hear industry experts Claudia Imhoff and Awny Al-Omari explain what’s behind the virtual star schema’s meteoric rise and how you can put it to good use in your own data architecture.
Watch this webinar to learn:
- How virtual star schemas uniquely curate and deliver complex, transactional data for business analysis and collaboration
- Why advances in data storage and in-memory analysis are key enablers of virtual star schemas
- How you can use virtual star schemas to transform your own analytics projects while simplifying your technology stack
Nick Jewell: Fantastic welcome everyone we're going to let a few folks trickle in and we'll go ahead and get started in about two minutes time.
Nick Jewell: For those of you early join us a little housekeeping before we begin if you've got any questions during the presentation, please type them into the Q amp a box at anytime you like we'll do our best to address them at the end of today's session thanks very much we'll start in two minutes.
Nick Jewell: So again, welcome to everybody on today's webinar we're going to let a few more folks trickle in we're going to go ahead and start in about one minutes time.
Nick Jewell: And again just a little housekeeping message for those that have just joined, if you have any questions during today's webinar please feel free to type them into the Q amp a box.
Nick Jewell: And we'll do our best to answer them towards the end of today's session so once again, starting in about two minutes time.
Nick Jewell: Alright let's get the show on the road so again a little housekeeping just before we get started questions throughout today's session feel free to use the Q amp a function here in zoom.
Nick Jewell: we'll do our best to get to those questions, towards the end of the webinar today.
Nick Jewell: But let's get underway so welcome to today's webinar, this is all about how to use virtual star schemas to eliminate complex data pipelines forever.
Nick Jewell: My name is Nick jewel i'm in quarter senior director of product marketing i'm going to be your moderator and DEMO show man today i'd like to introduce my co speakers.
Nick Jewell: So, first of all, Claudia imhoff internationally recognized expert on analytics business intelligence and all their corresponding architectures.
Nick Jewell: Dr imhoff has co authored five books and more than 150 articles for technical and business magazines.
Nick Jewell: she's also the founder of the boulder bi brain trust, which is a consortium of internationally recognized independent analysts and experts welcome Claudia.
Nick Jewell: Also, with us today is only elementary chief architect of in quarter with 25 plus years experience in building high performance database and data warehousing systems and of course analytics platforms so without further ado i'll hand over to Claudia to kick us off.
Claudia Imhoff: Thank you for the kind introduction Nick and also thanks so much to all my to in court as we're putting on yet another of these wonderful seminars.
Claudia Imhoff: All right, let me get to my first slide is the genesis of the star schema we're going to we're going to.
Claudia Imhoff: Put the wayback machine up and we're going to say it's the 1980s, again, who knows we're heady days and rock and roll you know Queen and led Zeppelin talking head.
Claudia Imhoff: We even had bon jovi bangles ooh and if you're into it, you know AC DC tests and a few of those good things, but it was also.
Claudia Imhoff: a wonderful time for the data warehousing era, there was a time when gosh it was just starting out, it was so exciting.
Claudia Imhoff: We had the Ai the concept, the idea of an integrated database taking data from multiple sources and somehow integrating it into this database, the sole purpose of decision support.
Claudia Imhoff: The relational db MS is working right the technology with us these with a date and cod rules for design normalization for a second, third, fourth, fifth normalization if you can get that high.
Claudia Imhoff: It was the most efficient way of course to store the data in these relational databases, the least efficient, though, for performance in terms of multi joint queries That was a problem for the relational database.
Claudia Imhoff: So that was the big change in analytics in the 80s, the entry of the star schema brilliant database design by Ralph kimball and his and his crew.
Claudia Imhoff: The idea was to create a physical star schema and it was a multi dimensional type of deal dimensions in fact table.
Claudia Imhoff: It was taking the relational design and going ahead and putting all the join together getting reasonable, in fact, very good response time.
Claudia Imhoff: If we look at the next slide you'll see that this is an example of a star schema the dimension tables sitting on the outside and then the Center is the fact table.
Claudia Imhoff: that's the physical instantiate of a star schema it allowed multi dimensional query.
Claudia Imhoff: Significant data do normalization, as you can imagine, it did not follow the David carr rules in fact it violated them quite violently.
Claudia Imhoff: But it was excellent, in terms of performance for these multi dimensional types of queries.
Claudia Imhoff: The fact table surrounded by the dimensions great way to to get these multi dimensional analysis it basically pre joined the data for you.
Claudia Imhoff: And as long as the analytical processes or the data never changed or you didn't change the questions that you wanted to ask the star seed schema was a fabulous design, however let's go to the next slide.
Claudia Imhoff: Difficulties did develop.
Claudia Imhoff: Like I said as long as the analytical processes, where the data never changed and life is good everything's great.
Claudia Imhoff: But you know as well as I do that an analytics environment is very fluid, it is constantly changing.
Claudia Imhoff: It is unpredictable We may want to look at these sets of dimensions today, but then tomorrow we're going to look at a whole different group of.
Claudia Imhoff: The analytical environments became kind of nightmarish in terms of complexity maintenance was what became difficult if you had to add a dimension to a star schema.
Claudia Imhoff: More than likely it didn't tear down the old star schema as a dimension and then rebuild it you just build another one with a new set of dimensions around it.
Claudia Imhoff: Slowly changing dimensional data was also problematic, it was really hard to keep that up to date and make sure everybody was on board with that.
Claudia Imhoff: And like I said always new needs for four dimensions different dimensions different ways of kind of slicing and dicing and cutting it all up.
Claudia Imhoff: And the need for new star schemas and they were mostly redundant in their basis, but they weren't knew a little bit different from the old one, and that means a loss of flexibility and a loss of agility.
Claudia Imhoff: As I stay down my slides the business community was not amused.
Claudia Imhoff: All right, let's go to the next slide and take a look at what what has happened over the intervening years over the 35 or so years that we have.
Claudia Imhoff: There is a better way today yay technological progress, technological advances i'm going to talk about three of them and then we're going to go into a deeper dive.
Claudia Imhoff: With awning when we talk about the in just a moment cloud storage of data great change we no longer have to have it in our in our data.
Claudia Imhoff: centers we could put it into the cloud, and that gives us a tremendous amount of flexibility in terms of splitting it up to handle more queries or data or.
Claudia Imhoff: Less storage wins or less performance when we're not using it quite as much, and then, of course, in memory wow memory used to be so expensive that we would do everything we could to keep it out of memory.
Claudia Imhoff: memory is dropped like a rock and fortunately now, we can put things in them read that we're not possible, before and then, of course, new query engine wow new things coming along the new query engines are absolutely remarkable in what they could do.
Claudia Imhoff: Okay next slide let's talk a little bit about cloud yeah gave us a lot of benefits here.
Claudia Imhoff: much reduced costs the elasticity of the cloud, which was really a tremendous boon, especially in environments where we have.
Claudia Imhoff: Massive queries one day, and maybe a trickle of them on another day.
Claudia Imhoff: The data storage orchestration over the different storage format itself whether it's Ram the random access memory or ssd the solid state drive.
Claudia Imhoff: Or the hdd the spinning disk itself, we had a choice we could pick where do we store our data really hot data goes in one of these caches really cold data us in frequently neko in a different one.
Claudia Imhoff: optimization that improves the performance by that better io usage of the query engine and the columnar memory, storage and we'll talk about that in a moment as well.
Claudia Imhoff: again next slide The next thing that we looked at in memory, as I mentioned wow and it's been dropping like a rock.
Claudia Imhoff: Most recently, they reduce costs of memory mean that data can can reside, either on disk or in memory it optimizes performance for queries by eliminating.
Claudia Imhoff: A lot of requests that dictate the disk storage data it does improve scalability with the decrease cost of memory it's been a great boon to the analytical environment that we now have.
Claudia Imhoff: And then, my last slide on the the query engine themselves.
Claudia Imhoff: wow just take a look at what we've got today what makes star schemas in some in some situations.
Claudia Imhoff: irrelevant, the physical start speeding up keep in mind, I am not talking about a virtual star schema i'm not talking about.
Claudia Imhoff: The design of this scheme itself, but the actual physical instantiate of the star schema may not be necessary anymore, and that solves so many of those earlier problems.
Claudia Imhoff: But the query engine now provide real time join between the complex data tables themselves and other it's we can have a virtual star schema instead of a physical one.
Claudia Imhoff: They do create the needed aggregations that we all know and love of some of the data that we have.
Claudia Imhoff: That it also yields a tremendous amount of flexibility, after all, it's much easier to change a virtual star schema than it is a physical one and that's important and will become even more important in the future, as we bring in more and more and more data.
Claudia Imhoff: Alright, so with that i'm going to bring him on and he's a smart cookie so listen to this guy.
Claudia Imhoff: we're going to talk a little bit more.
Claudia Imhoff: about the changes with the column their storage and how it's really different.
Claudia Imhoff: from traditional relational databases, we also want to talk a little bit about the changes in terms of storing the data and analyzing the data within memory analytics on is going to talk a bit about that.
Claudia Imhoff: And then, also the changes that that in coordinate is bringing to the table the data direct data mapping.
Claudia Imhoff: So only Let me turn it over to you, I want to hear more about the more technical aspects of what we're going to do today.
Awny Al-Omari: So, technically, thank you very much.
Awny Al-Omari: So, so in the following two minutes, we will be talking mostly about query performance and how in court is capable of executing complex many ways join mapping to.
Awny Al-Omari: Highly normalized three three and a half schema in in a blazing speed and the reason we talk about query performance in the context.
Awny Al-Omari: In the context of just checking point, this is my video and audio fine.
Nick Jewell: Okay yeah.
Awny Al-Omari: Thank you, this is talking about pretty performance in the context of diversity is you could see the new building that up.
Awny Al-Omari: So just to get to play for us now and and how how important solve the problem of query performance.
Awny Al-Omari: You can simplify the problem of query execution cost into two parts, the first part is the data access, and the second part is the data data access can define it as the.
Awny Al-Omari: Cost of reading the data from the data storage, to put it into a data processor in a processing.
Awny Al-Omari: ready format, this would include both that search apart, to find basically the part of the data that you need to do need a data set.
Awny Al-Omari: The read part from the from the media, the moving it then copying copying it to the right format and the other space so technology is driven to that our storage format column are partitioning indexing etc storage media ssd is memory.
Awny Al-Omari: envy me cetera and storage location specific locality versus remoteness, the second part is the data processing and we could see that in the case of bi.
Awny Al-Omari: The the overwhelming warming overwhelming part of a data person goes for the joint joint are the most.
Awny Al-Omari: Common type I mean typically quitting will find one group by but typically you could find multiple multiple we're joined drones are the most expensive and the most common part of the way grouping and sorting come come next and next slide please.
Awny Al-Omari: So let's talk about how in quarter adversity, to access a problem on what to do, I said, imagine in court and the format side our data is stored in a column are.
Awny Al-Omari: Really out as good as a deck and this is the the the the whole idea of column format using laying out your data column by column, rather than traditional.
Awny Al-Omari: row by row and give you two main advantages, the first advantage is that now, I can only read the columns that are relevant to my query and others to the table.
Awny Al-Omari: And the second one, which is probably even more important allow you to do compression very smart compression on that column data, because the same time, same frequency, etc, etc.
Awny Al-Omari: And incorruptible form, I want a quality pristine class in coding and compression because its balance between good compression rate useless of the memory space, but also.
Awny Al-Omari: in court, they can act when the compressed data, all the way up in the in the stack and there's something on a mission here we're talking about kumar and compression because there's.
Awny Al-Omari: been a trend and people have been claiming it for the last 1015 years.
Awny Al-Omari: In court is one of the few system that not only store data in column aren't compressed format, but act on the idea to all the way up into execution second That gives you tremendous performance advantage, for example, if you.
Awny Al-Omari: If you grouping or or or filter on ego so over included values, you have simple integers versus strings that's that's an obvious advantage when the medium side.
Awny Al-Omari: encoder store is data on memory so they'd have the data is is a memory facet more over, we have to do a lot of work to do very smart.
Awny Al-Omari: taken advantage of cpu caching and one or two and three so many for each repetitive and consecutive will only hit the.
Awny Al-Omari: nanosecond versus the Syrian and, second, or so and when the equation side, the data is stored at the processing note, not only that, in the same data.
Awny Al-Omari: processing the memory of the processor processor so there's actually no data transformation between or inter process communication.
Awny Al-Omari: or remote calls, so a few months of my definition for the data access coast, being in the course of taking the data from the from the storage.
Awny Al-Omari: or the way to the data processor address space in a consumable format, well, we already have it right there in in kota it can't get any better than that let's move to the next slide.
Awny Al-Omari: let's get to the joint so since the beginning of time three joints dominated industry.
Awny Al-Omari: The value and variation for this john's The first one is that is, that is to join.
Awny Al-Omari: This is where you pick the the the outer a new product, a new article.
Awny Al-Omari: Basically, the matching on the on the inner unless you were required with indexing from the from the inner table this is Kingdom on TV when you only access bureaus and certain type of DSS queries.
Awny Al-Omari: tactical level, we are only small and workflows but in when you go through the VI word has john is working and some some.
Awny Al-Omari: Some additional support systems and database systems only only support has joined when they're targeting via the.
Awny Al-Omari: API world and that's because it's very versatile that we have shown what is it read it before the table, usually the smaller.
Awny Al-Omari: read it and memory built in memory hash table and then use the entrepreneurial to prove and look up look up the attached able to find to find the joint.
Awny Al-Omari: it's very as you would get a summary it's very memory intensive because build attached table.
Awny Al-Omari: In memory certain region is a is a theater when you're going to go into it, this is about women that yet for completeness, is when you sort both sides and where's the result very robust but slower obviously that has shown let's go to the next slide and talk about.
Awny Al-Omari: How does include those are jobs.
Awny Al-Omari: That are created at the loading stage Okay, and we take that the June results that we pre compute and we use it to build an in memory.
Awny Al-Omari: Light data structure that will allow us to not from one table with one another, so basically what we have an extremely efficient in memory optimize optimize join.
Awny Al-Omari: If there's sort of this query time i'm not building any hash tables or, and this is, if you think is is really for you and you will equate will execute without the need to visit a new story structure at one time.
Awny Al-Omari: One one other thing to point is that because we're using this in memory built in memory structure.
Awny Al-Omari: i'm getting a note that the audio is breaking.
Awny Al-Omari: yeah so i'm going to have to slow down a little bit so because we are we're building this in memory structure ahead of time adequate time you don't build any joint and this this lead to a counter intuitive.
Awny Al-Omari: point is that it turns out that, while in memory are are are famous for using a lot of memory, because you started it and nobody, which is true for data storage.
Awny Al-Omari: At the query execution part they actually use this number three in the case of a hash join that we mentioned before, for example, if a concurrent query would have its own version of the hash table one in the case of the.
Awny Al-Omari: In memory system built right like the encoder is a execute its own its own joins we you will not need to build your own copy of the hash table do you have actually and in the city and this index is being used by the multiple concurrent queries.
Awny Al-Omari: We don't store any intermediate results, except the final result that region for the final.
Awny Al-Omari: Final retrieve data and it's like this.
Awny Al-Omari: So how but most high table john so see I know I wanna I want to join between I want to get the.
Awny Al-Omari: My total revenue per country and error per category, so the inquiry user would pick the measure from the sales being the revenue and.
Awny Al-Omari: And the dimension from the category and country and incorporate under under underneath will build with figure out what the joint if you're here, I need to join seals to productivity.
Awny Al-Omari: To country at the execution time this was are connected through this in memory hubs which I, which I wasn't index index joins.
Awny Al-Omari: And then technically execute through here record in few in memory helps this let's Compare that to see the advantage against traditional in the next slide traditional.
Awny Al-Omari: system, how do you compute which will have you on the right hand side that has joined for the case of the hash run the same query will be executed as well.
Awny Al-Omari: Each one of these tables I assume it to join.
Awny Al-Omari: Sales to product to continue to collect category.
Awny Al-Omari: So, first we scan product country category is a concurrently or or different steps that depend on the system, and you build a hash table for each of these.
Awny Al-Omari: In our in our relationship, and this is an expensive part and memory intensive.
Awny Al-Omari: And then you you take that out of the oven, which is this case here's the larger largest table and you approach each of these tables looking for.
Awny Al-Omari: For a match this This includes the computation of the hash value for for for each of the jockeys.
Awny Al-Omari: And then be with a case of a match look up into the hash table and compare the final result, to make sure you actually have equality here, this is very expensive.
Awny Al-Omari: compared to what you have between the Left and the Right we're talking nanoseconds versus microsecond good looking for those nuggets and that shows why why input, the can execute complex Jones at the high speed, the next slide and my last slide.
Awny Al-Omari: So what do I have in practice in somebody I have right now my data in highly optimized the process processing really format column format in memory.
Awny Al-Omari: Do soggy stables, that we have here in these circles and then between the tables, I have a index join mapping joint index that's really it and the joint the related tables together, which means in totality you could look at this.
Awny Al-Omari: column our presentation, as well as the joint index as the legal steps that together will for me this schema level composite.
Awny Al-Omari: index, or what I would like to call it a joint superhighway because right now just pick your your your measure from any of these tables and that people become basically the the fact.
Awny Al-Omari: The fact in a virtual start and you pick the dimensions and or filter from the other tables and that makes him.
Awny Al-Omari: The dimensions and what are you have basically a build a virtual will start on the query will execute when it's super super fast in.
Awny Al-Omari: In real schema schema is much more complex than, why should here and it worked out actually if you do the math this astronomical number of ways that you can organize.
Awny Al-Omari: A tic TAC tables with permission tables living for grants one of those are available under your fingertips, without any materialisation aside from these pre computed join, so instead of having lock yourself to one or two physical.
Awny Al-Omari: stars, you have under under under your fingertip and literally a galaxy of stars, and this is where I hand it back doing.
Nick Jewell: Fantastic Thank you very much, only a galaxy of virtual stars indeed so i'm just going to stop sharing the presentation for a moment jump over.
Nick Jewell: For a short demonstration really to introduce the in quarter platform to you all show you how we can build powerful virtual star schemas.
Nick Jewell: and start to deliver insights really without lengthy or complex data pipelines slowing us down.
Nick Jewell: The endgame, of course, is to show how we can go for some raw source data as only talked about so these kind of rich data insights with an absolute minimum of data transformation modeling or specialist IT skills.
Nick Jewell: But i'm going to start at the very beginning and i'm going to start by talking about our data now in quarter has a vast range of data connectors.
Nick Jewell: Out of the box, these include databases business applications file systems data lakes API connectivity.
Nick Jewell: Even streaming data, so we can build a virtual star schemas from over 240 connectors.
Nick Jewell: At the last count now for the DEMO today i'm going to be working with some predefined data sources, this is going to include a complex application database for a national retailer.
Nick Jewell: Now, typically working with real world application data means a lengthy IT project right data architects modelers other folks who coax that data from its original transactional form.
Nick Jewell: into a structure more typically suited to analysis, maybe, that means our traditional star schema maybe even mean something simpler like a flattened view.
Nick Jewell: Well, within quarter none of those steps are required we're going to get that data insights ready without needing to remodel or transform the data from its original structure so.
Nick Jewell: The DEMO today is all about saving time and resources along the way, but making sure that we make the most of our skills to present the data to the business users in the most effective way.
Nick Jewell: So i'm going to kick off by going to the schema tab where we're going to get in quarter to understand the structure of our retail data.
Nick Jewell: i'm going to click new and we're going to use our schema wizard to make things really easy, so let me give our schema a name.
Nick Jewell: going to drop in and pick out online store as a data source click next down here at the bottom.
Nick Jewell: and basically over on the left hand side, we can now start to select the tables and the assets within.
Nick Jewell: Our data structure bring them into in quarter for analysis and here I can explore these tables so, for example, I can see how in quarter is going to treat the data.
Nick Jewell: Whether that's as a key a dimension of measure very common terms i'm sure to everyone on the webinar today.
Nick Jewell: They use the drop down if I want to change behavior we can re label our fields, if we want to add more meaning to these attributes, we can even change data types themselves.
Nick Jewell: Lots of flexibility, but we're just going to make sure that we're selecting all the tables we're going to click next.
Nick Jewell: And then on the next screen i'm going to click create schema to start this process.
Nick Jewell: But basically, our wizard will ensure that any metadata structural information from the database gets brought into play so, especially the connections, or the joins between tables in the raw data itself.
Nick Jewell: Now point to note we haven't actually ingested any data into in quarter yet, but we have laid the groundwork, so if we click on the diagram button up here at the top.
Nick Jewell: we're going to get to see a complete picture of all of our source data with all the connectivity between those tables basically ready to go.
Nick Jewell: And remember in traditional data architecture projects, this is the it heavy part of the Program.
Nick Jewell: Data gets moved transformed remodeled until it's often in quite a different format for analysis not here we're going to work with that data directly in this source format.
Nick Jewell: Without any costly data transformations and here we can see some of the normalized application tables.
Nick Jewell: That we'd usually try to bring together as dimensions, maybe in our model, so we might take product subcategory and category.
Nick Jewell: And roll that into a single product dimension, we might take the sales order itself and say, this would be another good dimensional candidate from address to state to country even salesperson and sales territory but.
Nick Jewell: So far, all of the objects that we've loaded from our data source have been simple mappings to tables themselves within quarter, we can also bring in.
Nick Jewell: table aliases derived tables whether that's from sequel or using in quarters data is tools we can also embrace code more completely and bring in a materialized view, and this is where we can work directly with.
Nick Jewell: sequel Python are or scholar against in quarters Apache spark service so really to use this to create and serve up some more advanced analytics for our users.
Nick Jewell: When we're ready, we can kick off either a full load, or we can use incremental loading to bring back recent changes depending on our situation so.
Nick Jewell: This can really help us present and track historical data back to our users, much like the slowly changing dimensions but Claudia mentioned earlier on in traditional dimensional modeling.
Nick Jewell: In this case, i'm going to click full load we'll get that started we're absolutely looking with real application volumes in this DEMO so that will take a second or two to run.
Nick Jewell: In the meantime i'm going to jump back to my schema menu and i'll drop into a pre loaded schema to give you an idea of something a little more complex.
Nick Jewell: So let's open up the diagram view you can see here we're dealing with e rp data financial data in fact we've got over 2 billion rows of data loaded and compressed inside in quarter now.
Nick Jewell: At this point, we can see data from financial closes, we can see some fema simulations we can even see data brought in from our CRM system.
Nick Jewell: down here at the bottom, so a great way to visualize and bring together disparate data sources for analysis if we click the explore button up here at the top.
Nick Jewell: were taken into in quarters built in data visualization studio we can immediately get to work with all the tables in the schema from the panel on the left.
Nick Jewell: or choose from a really wide range of data, this styles from the drop down basically everything you'd expect from a modern bi tool.
Nick Jewell: possibly more since this aspect of the platform is completely eXtensible via our SDK so let me quickly build an aggregated table or bring in a couple of grouping dimensions, at this point let's drop them in here i'll select a couple of measures as well.
Nick Jewell: And we can see instantly that direct data mapping that only talked about giving us instant results off the source data with no transformation no weeks of waiting.
Nick Jewell: For a technical project to deliver the data in a format for analysis now Ralph kimball used to talk about a data warehouse dining experience.
Nick Jewell: Where raw ingredients got processed in the kitchen got sent out to customers in a dining room kind of with the implication that diners shouldn't really be wondering loose in the kitchen.
Nick Jewell: The view we see here is a bit like a chef's table so valuable access to the raw source data for ad hoc queries not the polished dining experience but i'm going to show you what that looks like next i'm going to jump out of this view, right here.
Nick Jewell: And we'll quickly have a look back at our schema let's see if I load has finished at this point.
Nick Jewell: So it was a DEMO there it is so it's completed it's loaded 25 million rows in about a minute and a half, not too shabby but now we're going to curate.
Nick Jewell: The content of this business application into an in quarter business schema a view for self service analysis.
Nick Jewell: Now, this is a really simple process we'll head over to the in quarter business schema tab will open one that i've created a little bit earlier and remember.
Nick Jewell: Traditional data projects, this is the stage where you get your data teams your bi development teams to shape and transform the data they've applied all kinds of data engineering decisions within quarter, we can get to work here with absolutely minimal intervention.
Nick Jewell: Between that source system and the insights So here we see virtual dimensions, like the retail location.
Nick Jewell: Or we can easily bring together products and orders into a collected view so that they're easily accessible.
Nick Jewell: We can enrich our data you'll notice here i've created a formula using some pretty intuitive formula functions to present data that wasn't available in the source system.
Nick Jewell: And we can explore this data in much the same way, but now, with a beautifully curated virtual star schema.
Nick Jewell: And at this point, maybe we'd like to explore the data, very quickly, a little further i'll bring in a geo spatial analysis i'll use a bubble map.
Nick Jewell: i'll drop in my state and province name lots of control over the visualization so here, maybe i'll just simplify the name, make sure my map is pointing at the state level.
Nick Jewell: And i'll drop in that revenue field that we just created and again almost instantly we get the visualization Washington California New York or big hitters when it comes to revenue.
Nick Jewell: I can save this insight over to a dashboard at this point so i've got a folder pre created here i'll drop that into my dashboard.
Nick Jewell: And now I can start to interactively analyze the dashboard or create new content let's very quickly add another listing table and at this point i'll drop in my sales order ID.
Nick Jewell: they'll drop in Category subcategory may bring in revenue once more.
Nick Jewell: The results changing instantly maybe I don't like the formatting when it comes to sales order idea kind of a fussy when it comes to number format so i'll get rid of the comma.
Nick Jewell: And again very quickly updated will save this will drop this into our dashboard as well, and now we start to interact, so I can hover over a particular state such as Oregon.
Nick Jewell: I can very quickly, see the data change down at the bottom all of the revenue down at that most transactional level if I want to go further and make additional selections maybe i'll select road bikes in Oregon.
Nick Jewell: We can see the number change both at the top and the bottom and, of course, very flexible to apply filters and explore the data in your own way.
Nick Jewell: So basically we've done no no pre aggregations no industrial data engineering just a seamless virtual star schema that can be refined further connected to other data sources and developed as the business sees fit.
Nick Jewell: So Claudia virtual star schemas harness I think many of the forces that you mentioned, and only some amazing technical innovation behind the scenes back to you.
Claudia Imhoff: All right, well let's get started with the next section of our of our talk, and that is the Roundtable and the Roundtable I hope will be most interesting to people.
Claudia Imhoff: it's a compilation of a lot of the questions, but this is our third webcast on this on this topic, so this is a compilation of many of the questions that we received in the first two, and just as a reminder to everybody.
Claudia Imhoff: Please do submit your questions we've already got a handful of listeners, I hope we get a handful more, we would like to get to your questions, after the Roundtable as well, so let me kick it off a little bit here.
Claudia Imhoff: The first one, we got whereas with all about the best practices and i'm just going to read it off so pardon me.
Claudia Imhoff: What best practices carry over with virtual star schemas so Nick i'd like to start with you, and if you don't mind i'm going to call in on an answer from at the end of after you finish.
Nick Jewell: You you've got it so i've got two answers one sensible one, a little flip and i'm going to say first of all, something from my experience working with star schemas and kimball methodology.
Nick Jewell: Is the idea of a dimensional bus matrix it really helps understand which dimensions apply to which facts it's a very good way of organizing what it is you're about to build.
Nick Jewell: But honestly what practices carry over it's about listening to the business it's all about that conversation to understand whether you're modeling a business process in a way that will drive insights.
Claudia Imhoff: And what's your flip it one.
Nick Jewell: That was the slipping well it's like simplest things listening to the business it's just one for an analyst right.
Claudia Imhoff: I just wanted to emphasize that because that's part of what my answer is going to be, and that is for heaven's sake, the design is still needed must, you must listen to the business people.
Claudia Imhoff: The design is what carries through to the ultimate design in the database itself, it may not look like the the on paper design.
Claudia Imhoff: But certainly that's how we get all of the requirements that we need and that's listening listening to the people listening to the business.
Claudia Imhoff: Okay second one what gets left behind from legacy Paul at practices and other if we if we follow your advice and we're doing this new world order that gets left behind what don't we need more.
Nick Jewell: Sustainable clothing i'll start off again if you don't mind, so I went through my big library and I found a book from.
Nick Jewell: called the business intelligence roadmap and he kind of laid out this itemized project plan for star schemas and bi.
Nick Jewell: There was 500 plus line items and i'm going to argue that complexity is long gone and I probably say it never would have delivered in such a rigid format anyway, so I think what you see with in quarter.
Nick Jewell: Is that we're really preaching agile iterations build test refactor based on the results that you find it's leaner it's me now you have a much smaller development and delivery team it doesn't need as much heavy involvement from it, or data engineering.
Claudia Imhoff: yeah I would add also that, on the maintenance and it's a much simpler process as well.
Claudia Imhoff: Yes, certainly getting it built is is easier you're absolutely right about that, but even maintaining it is simpler if we don't have the physical nature of the star schema is.
Claudia Imhoff: If we can just maintain these virtual stars life is so much nicer I have to admit, on the let me bring you into the conversation.
Claudia Imhoff: Because there's I think there is confusion about a virtual star versus virtualization we've seen that question come up more than once, so i'm going to turn it over to you what's the difference between a virtual star, if you will, and virtualization itself.
Awny Al-Omari: So the question is what is virtualization virtualization is basically an obstruction providing an abstraction layer between.
Awny Al-Omari: Reality or or or or physical design that is complex, somewhat undesirable, just like to build a view on it that says view is.
Awny Al-Omari: is most user friendly and more unified more agile, I guess changes under underneath.
Awny Al-Omari: So, in that sense, and then purchase any record two components, one would be this mapping between mimic what is the physical layer for the record.
Awny Al-Omari: button for now, and the logical layer for the view and also the ability to the sufficiency, otherwise you don't have a viable solution so in data virtualization usually is concerned about.
Awny Al-Omari: abstracting the location of the data, the source of the data, the format of the data, so my data would look like a logical table, you know could be on my sequel table or MARQuIS the file or or or or Cassandra.
Awny Al-Omari: So it will hide all of that, on the other, on the other hand, start virtualization or virtual star is concerned sort of certain but this abstracting.
Awny Al-Omari: The schema if I say boudicca the schema with the complexity, to which is three accomplished in.
Awny Al-Omari: Providing to you and NASA looking virtual data set or or dimensional dimensional look and giving you an additional capability of executing.
Awny Al-Omari: Without looking yourself to one one physical.
Awny Al-Omari: design, so I would say this, the conceptually the same in the sense that there are both of Section abstracting trying to simplify and unified.
Awny Al-Omari: Complex layer underneath but one is concerned, more about the data source and the data and the part of the DNA from seo with others about the team or the GL, which is the digital transformation that transformation.
Claudia Imhoff: All right, well done.
Claudia Imhoff: I don't have to take another one, least i'll take a stab at it first either one of you jump on in there, if you have more to contribute.
Claudia Imhoff: What should star schema practitioners be thinking about before they make the transition to the virtual star.
Claudia Imhoff: Do they have to throw away dimensional modeling no no we've already established with dimensional modeling is an absolute requirement.
Claudia Imhoff: got to get the the requirements from the business people, but I think one of the things that you should pay attention to.
Claudia Imhoff: And this is has not changed at all, and that is the metadata behind what you're modeling.
Claudia Imhoff: All of that metadata collecting as much as you can about where the data came from what happened to it, whether it's aliases on and on and on.
Claudia Imhoff: Other other formats, that it may show up in and so forth, because that's all going to feed into.
Claudia Imhoff: some kind of catalog or some way of actually fighting you saw Nikki is pulling aliases who's pulling all kinds of things.
Claudia Imhoff: So you've got to bring all of that metadata forward into your analytical environment, and that also includes the data quality itself how good is the David you know, do we give it a thumbs up we've got it certified whatever it is.
Claudia Imhoff: So please don't forget about the metadata in your rush to go build a virtual star schema the metadata is still very much needed any comments from either one of you.
Nick Jewell: Know 100% agree floater I think the importance of the semantic layer is now paramount because.
Nick Jewell: You don't need to build a physical layer that now models that pre semantic layer, so I think everything's got simpler, but the discipline of building something that the business can understand can work with.
Nick Jewell: And then can ask additional questions that you can quickly serve up absolutely critical.
Claudia Imhoff: Wonderful alrighty next one do in quarter developed virtual star schema is only work against bra transactional data set What if my company already has analytics.
Claudia Imhoff: Data architects that that they include stars or materialized views, so let me start with you, Nick and then audio i'd like to hear your comments about this one.
Nick Jewell: that's perfect Thank you and also this this touches on a question in the Q amp a from Lorenzo where he says can in quarter combine more than one connection two different data sources so, for example, can we combine.
Nick Jewell: SAP Oracle sequel server and bring them all together absolutely and in the very short DEMO that I showed today.
Nick Jewell: We had an example where we had a rp data CRM data, we also had data that was generated through data science methodology as well, so the idea of bringing in Python them to calculate customer churn.
Nick Jewell: All of these things brought together and from my brief time it in quarter so far.
Nick Jewell: i've definitely seen this works on anything where you have knowledge joins were previous systems have just struggled to bring those data sources together the direct data mapping in in quarter is a phenomenal way to unlock that data.
Awny Al-Omari: Thinking Okay, I want to add to the grid answer than account is that we, there is a number, there is a value actually also pointing to that to the three enough data So yes, will the encoder will be able to carry all of executing you're already pretty much analyzed star Jones.
Awny Al-Omari: It but he was paralyzed something from a three enough to Saskia you lose a lot of information, which is the rest of the that connectivity, so you would be able to execute that but without pointing to the three and a half.
Awny Al-Omari: original origin origin, the schema in some form, you would lose a lot of the.
Awny Al-Omari: ability to do valuable discounts, you are virtual start that you can enter your your suck a limited to the model that you're presenting.
Awny Al-Omari: to incorporate that if you if you only you only present to it, the the Saskia you will work on that star schema but you want to you want to go back to the origin of it enough to be able to live ideation and have the additional visual stuff.
Claudia Imhoff: All right, let me get to a question that we have number of times in that we focus on this.
Claudia Imhoff: up in these last three focused on schemas, but there are other data modeling types out there, for example, data volt we've heard about that you know that type of thing is it possible to incorporate a particular data mining techniques.
Claudia Imhoff: Virtual star schema for from a date or anything else i'll start with you.
Claudia Imhoff: answer to that question.
Awny Al-Omari: Okay i'm city, this is the sound was breaking click your mouse you just to repeat it again.
Claudia Imhoff: The idea is that we focused on the schema, but there are other data modeling.
Claudia Imhoff: Like default, for example.
Claudia Imhoff: How did How does that work in in court.
Awny Al-Omari: So yeah i'm gonna I give a kid full answer to this one, so I would say, in principle, yes, you could you could utilize in court, I guess, other.
Awny Al-Omari: Data models and this example of the data vault the and, as you said, it's it's we really provide you a way to map and I think an actual some example of this mapping and to execute that mapping at high speed between Heidi normalized format to a higher.
Awny Al-Omari: dimensional level modern again, even in in a data vault data both is a great modeling paradigm to track basically your history of the data warehouse and guess changes with these changes being.
Awny Al-Omari: In the underlying data models or the slowing moving down their missions, but it's that that's an expensive Heidi normalizing that data, so you have the hubs and the satellites.
Awny Al-Omari: The and that's what we need so much to do a dimensional view of that, so I think in quarter in principle may work that I don't know if you have a customer who's done that but.
Awny Al-Omari: I think that should work I would use, probably the the the the business of view to obstruct the the.
Awny Al-Omari: The hubs and the satellite as as a dimension and then use the links as a as a fact and would be I would love to have a concrete example where this word, but I think it possible that you don't.
Claudia Imhoff: A comment from you.
Nick Jewell: know, I was gonna say only has opened the doors the invitation is there anybody on the webinar if you have that particular setup come and talk to us.
Claudia Imhoff: we'll do.
Claudia Imhoff: The last few anything computed with a virtual.
Nick Jewell: So only we might have some issues with claudia's audio for a second i'll just i'll take that question for a second, so the question was for you is there anything pre computed.
Nick Jewell: With a virtual star schema that we're seeing today.
Awny Al-Omari: Absolutely yeah that's a very good question and any any I think I mentioned that in the in the slides no baby will go we're going fast, but.
Awny Al-Omari: We do pre compute the two way joins between between the common tables that have relationship.
Awny Al-Omari: Between between them to sell the common joins or the tables that they just er relationship between the stables move to compute this john saw this is.
Awny Al-Omari: Sometimes a joke, we do have it here we have ej ella's and we conclude this joins, but these shows right now become building block okay for a combined tutorial possibilities of how you construct.
Awny Al-Omari: Your your your star you started actual virtual stuff so it in a way to it's a Compostable components that allow you to do a combination of multitude of visual such.
Claudia Imhoff: Fantastic thanks also to.
Nick Jewell: yeah how's your audio now.
Claudia Imhoff: turned off my video So hopefully it's okay we'll get you give me a thumbs up if it's okay.
Awny Al-Omari: Just.
Claudia Imhoff: Okay, this is an interesting question and I thought it was a good question where does the virtual star schema go in quotes with it's not being used.
Awny Al-Omari: It goes nowhere.
Awny Al-Omari: Because never existed.
Awny Al-Omari: They.
Awny Al-Omari: submitted it wouldn't Actually, I think, maybe the question come from the thinking is that hey do we.
Awny Al-Omari: Do we a cashier such that for for performance so cash the results and you could do this, but we execute the square so fast that we didn't find even the need for caching D it's think of it as a as a as a query level Meta data.
Awny Al-Omari: We don't dematerialize anything we were the only thing we need to realize is these.
Awny Al-Omari: tables and computed the vegetables and the computers and the completed joins and we will try everything from there.
Claudia Imhoff: Alright sounds good to me.
Claudia Imhoff: Next last question how would we have operational modeling with virtual star schema things like data cleanup data across courses or handling chasing labeled or relationship Nick this sounds like one right in your wheelhouse.
Nick Jewell: Absolutely i'm gonna go back to my my kimball's restaurants analogy, which will be trending on Twitter after this talk i'm sure so basically the operational modeling is all the backroom steps that happened in the kitchen.
Nick Jewell: All that stuff you need to do before you present data for analysis is in the dining room.
Nick Jewell: And i'm going to say that within quarter, you can of course conform data against your master data sources, to make sure.
Nick Jewell: That you're using approved cleaned elements, or even to clean and process data directly using things like formulas that we saw in the DEMO.
Nick Jewell: or even sequel or code in Python are or scholar, so there are lots of options, I think, to understand that we can actually make many, many changes in that back end before we take the data through to the virtual star schema and presented to the end users.
Claudia Imhoff: Excellent How does virtual 30 might fit into a modern data includes either a cloud data warehouse or a data lake so let me see Nick why don't I start you on that one.
Nick Jewell: yeah perfect and then i'd love to hear only on his view on this one as well, so.
Nick Jewell: work that I do it in quarter, we often see that with both cloud data warehouses and data leaks.
Nick Jewell: We see data models, I guess, in several different zones now whether they're called bronze silver or gold or raw muddled or presentation, depending on the vendor that you're talking to.
Nick Jewell: The goals are basically the same it's to transform it's to coax data from that original complex data format into a simpler analytical one.
Nick Jewell: Now within quarter, we can work directly with the raw or the Bronze data from all of those sources and kind of treat it as a final analytics presentation layer so you've seen the virtual star schema.
Nick Jewell: Without the need to invest in all of that heavy transformation or creating multiple copies of the data really is it's a huge game changer when it comes to things like delivery costs and performance, what do you think Tony.
Awny Al-Omari: I think I think you nailed it big in could provide end to end from the extraction loading analysis, but if if a customer or user already have their data loaded into a party format, we can take it from there from the.
Awny Al-Omari: From the data lake and execute.
Nick Jewell: Well, thank you, Claudia Thank you only for you, if your conversations in the round table i'm going to hit this one over now to some audience q&a for the last few minutes so.
Nick Jewell: We still do have time, if you want to get a question answered, please drop it into the Q amp a panel, the first one that I see is from ganesh and I think this is for you only is there a limit in the size of data to build a virtual star schema.
Awny Al-Omari: Okay i'm glad someone asked that question because I knew that the memory issue could be could be troubling for some so.
Awny Al-Omari: Okay, so there is no hard limit, I would say, be there, we didn't get into into the details so even though, is this holiday in memory part, but we also disrespect, which means we do we do a.
Awny Al-Omari: swap basically at the column level in a in a in a very high efficient we some of the data this little last last last just simply use to disk and read and read it.
Awny Al-Omari: on demand, so what it turned out to be is not the What matters is not the size of your entire dataset what what does matter is the size of the working set, which is the heart most.
Awny Al-Omari: 90 plus percent of the of the queries that come Come on, if we if you if you as long as you're working set fits in memory and you can we can we can we have a very intelligent algorithm for for swapping you, you can execute very well i'm i'm.
Awny Al-Omari: i'm amused by the size of the data that serve our customers have um it's it's it's scary event Natalia what city you're talking billions and billions of rows in the second excuse missing a lot.
Nick Jewell: Absolutely.
Awny Al-Omari: yeah well, if you want to click stream data or iot are so important from probably not the right platform for you.
Awny Al-Omari: know.
Nick Jewell: That makes sense, I was just gonna say in terms of the DEMO when you load 25 million records in a minute when you load 2 billion records.
Nick Jewell: In not much longer it almost looks trivial at that point, but really it's doing a lot of heavy lifting behind the scenes to make that as elegant as it is.
Nick Jewell: Thanks arnie another one for you and for in quarter joins does it require to define query attributes up front that's from what about anonymous attendees.
Awny Al-Omari: No, no, we have to define the the the joint quaid is, what are we joining on which is usually the primary key foreign key relationship, but until all the table, all the columns in both tables are game for.
Awny Al-Omari: You do need.
Nick Jewell: testing and we have another anonymous question so are virtual schemas shared across users and i'll take the first step of this one, if you like, so.
Nick Jewell: When we connect to an oracle EBS or an SAP you know these big complex business applications.
Nick Jewell: there's a lot of security metadata available in those systems and we can bring that across as well as part of what's known as an in quarter blueprint.
Nick Jewell: And that can make that security data that row level security application level security available to users who need to access those virtual schemas so rather again.
Nick Jewell: Then redefining and reinventing the wheel when it comes to a star schema we can reuse what you already have in place in the business application so.
Nick Jewell: it's a really great way of saying you're allowed to see this level of data in the source, you can see exactly the same level of data in the virtual store as well anything you want to add on you.
Awny Al-Omari: know I think you know.
Nick Jewell: Perfect okay um well another anonymous one this time again for you only and Claudia you might want to chime in as well, how is this different from snowflake.
Awny Al-Omari: world outside of your question, how is that similar.
Awny Al-Omari: I mean.
Awny Al-Omari: it's not like is a is a data warehouse system on the cloud.
Awny Al-Omari: They have done a great job on on on scalability is if us, and so we incorporated, that is a totally different experience, where we are where we're focused on on.
Awny Al-Omari: The problem for this thing, how do we execute queries with high performance that on the third number for most of data and the data warehouse has already been curated in a in a in a more.
Awny Al-Omari: Multi dimensional type of type of model link it's also distributed great for handling huge large amount of data or omissions example if you were to click stream data also probably.
Awny Al-Omari: better off with data bricks and stuff yet because i'm not get as it is larger core message to the security, but if you're dealing with high value data like CRM corp is financial data with a pistol.
Nick Jewell: Fantastic and Claudia from your experiences sort of a an industry thought leader, how do you see in quarter and snowflake positioned.
Claudia Imhoff: you're putting me on the spot here, but I will say, but let me put it simply, the goals are the same both companies want to deliver the best experience in terms of analytics and.
Claudia Imhoff: performance and so forth, is the cloud how you get there, how in court it gets there and how snowflake gets very different so.
Nick Jewell: Fantastic Okay, I think we have time for a couple more questions I see, there are many questions now arriving in the Q amp a will do our best to reach out to you after today's session and get you the answers that you need.
Nick Jewell: The first one i'm going to take is from Steve said simon's he says, what is the back end database and can we read from it so.
Nick Jewell: Only you've shown in your presentation slides were using columnar park a format, as the backend not strictly a database, but more of a data repository or a data lake.
Nick Jewell: However, from an end user perspective if you're in tablo or power bi or, indeed, other bi tools, you can access and query and read from in quarter, as though it was a postgres database right only.
Awny Al-Omari: That is correct, yes, we will provide a sequel interface that you can access our data processing engine and and execute your sequel query with suppose Kristen purpose.
Nick Jewell: Fantastic Okay, so I think Claudia as well you're you're you're letting me know there's another question about does it include to just access your data and make it available or does it store the data so.
Nick Jewell: It ingests the data and transforms it into this park a storage layer and then all of the great technical innovations that only talked about in his segment can be applied to the data in that format So hopefully that answers that question.
Nick Jewell: And i've got time I think for one more right now.
Nick Jewell: Let me just check out and find it so Joseph asks what's the process that we use with clients to size our deployment of in quarter, well, we have a number of enterprise partnerships, one of which is with wipro.
Nick Jewell: And we pro have developed a really interesting T shirt sizing approach when it comes to in quarter sizing.
Nick Jewell: Particularly when we're working with large business applications like Oracle EBS and their T shirt sizes are often based on the number of rows so whether we're talking about hundreds of millions billions of rows.
Nick Jewell: The number of obe or Ob AIA applications that might need to be migrated or modernized as part of that process.
Nick Jewell: And we can make sure that those details are available to you, and anybody else who's interested as well, but we very much have a definite framework for helping clients deploying quarter different sizes.
Nick Jewell: So with that, thank you very much that's all the time we have for questions today, please join me in thanking Claudia and only for their time.
Nick Jewell: and sharing their experience with us today all left me to say, really is to say check out in quarter cloud.in quarter.com i'll post, the link in the chat Thank you very much for joining us today and have a fantastic day.
Nick Jewell: Thank you.
Hosted by:
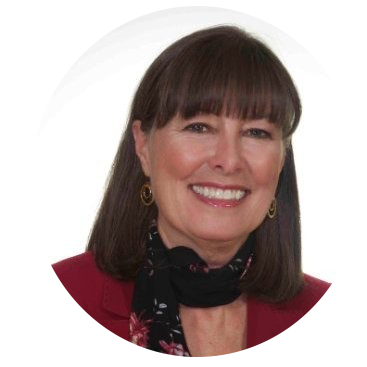
Claudia Imhoff
CEO Intelligent Solutions, Inc.

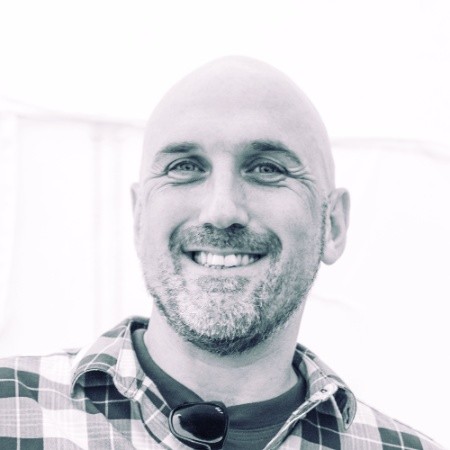
Nick Jewell
Senior Director, Product Marketing
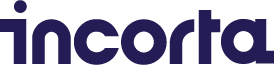
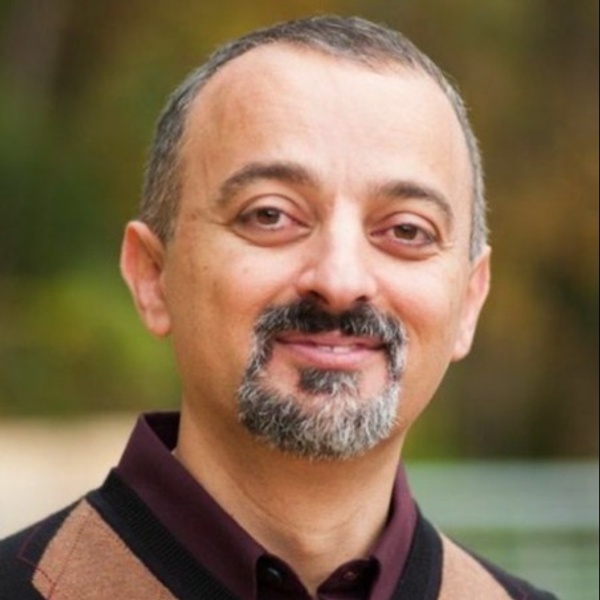
Awny Al-Omari
Technical Fellow and Chief Architect
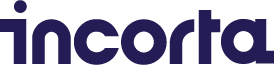