5 Key Considerations for Upgrading OBIEE to a Modern BI Solution
Creating and updating data models and reports using Oracle Business Intelligence Enterprise Edition (OBIEE) is a lengthy and resource-intensive process. Requirements gathering, schema development, and data modeling can take weeks or even months. However, million-dollar operating decisions that rely upon dynamic enterprise data from Oracle EBS, NetSuite, and other operational data sources can't be put on hold while data is made ready for analysis. It’s time for a new, modern approach to Oracle analytics.
Watch this webinar as we explore how you can unlock the full potential of all operational data in complex source systems to gain up-to-the-minute operational insights in record time.
You’ll learn how Incorta enables you to:
- Expedite your organization’s migration from OBIEE and drastically speed time to value for Oracle analytics projects.
- Directly map to Oracle data sources to eliminate traditional transformation and aggregation steps and deliver all usable data to the business in record time.
- Achieve new insights with custom dashboards featuring multiple types of interactive visuals in a single view.
- Validate insights by drilling down to the detail — from summary metrics across various data sources to transaction line details instantly.
Transcript:
Amit Kothari: Welcome everyone, we are going to let everyone trickle in and we'll go ahead and get started in two minutes.
Amit Kothari: Little housekeeping before we begin, if you have any questions during the presentation, please type them into the Q amp a box at the at any time and we'll do you know we will do our best to address them at the end during the green a green on session.
Amit Kothari: Again, but we'll get started in just a minute here, please stand by.
Amit Kothari: hi everyone.
Amit Kothari: Welcome to our webinar today the webinar is titled Oracle series session to click on five key consideration for upgrading rbi to a modern bi solution, my name is.
Amit Kothari: Terry and I am a senior director of solutions engineer in here and i'm going to be introducing our topic today and i'll also be your moderator for the q&a later on i'd also like to introduce my my co co presenter.
Amit Kothari: Alex Turk Alex dark is encoder sales engineer based out of Dallas Texas, he has nine years of experience in BA in analytics industry.
Amit Kothari: With seven of those years, being in the you know professional services capacity this on the ground knowledge helps in beta understand the data challenges outline space without further ado, I will kick us off.
Amit Kothari: Go go deep into the, let me start with.
Amit Kothari: Talking about some of the challenges which we have seen our customers are faced with toby I know he does this is one of the big four teams have of challenges which we have seen.
Amit Kothari: First of all, is difficult to achieve timely insights so what's happening is right, because obe.
Amit Kothari: For big you, you need to have a star schema kind of a model where you have to have atl and you have to have data data.
Amit Kothari: transformation lot of data transformation it actually makes the incremental refreshes with various new way of seeing multiple customers who are just able to do.
Amit Kothari: Two or three refreshes a day, and that is a big problem during closing and and basically getting timely insights so near real time is near real time, meaning like 15 minutes or 30 minutes is extremely hard to do in because of this lot of.
Amit Kothari: Data pipelines and reshaping which is going on.
Amit Kothari: The other big other big problem which we have seen is.
Amit Kothari: it's very hard to model data also so you so, you know as you might be knowing familiar with the va.
Amit Kothari: and, obviously, that we have this admin tool which is the already have to model, the metadata in that so there's a physical layer logical layer presentation layer
Amit Kothari: And it's a very heavy too so there's lots of stuff which is, which is very complex and even need a lot of developers and you know to produce even small changes so this Meta data modeling is because of this complexity.
Amit Kothari: it's very hard to to basically service request as soon as possible when they typically they're there they have a lot of sort of lag time on this.
Amit Kothari: We have also seen the dv interface, the data visually the one of the other tool is there it's very hard to do even complex models in that is just simplistic kind of modeling.
Amit Kothari: Other big problem we have seen is it's.
Amit Kothari: If you look at any customer implementation right there are multiple data sources so they have increased my data sources, they have cloud data sources, and now they want.
Amit Kothari: To basically join all this data together and surface this data in your dashboard.
Amit Kothari: So that's extreme that that is much more difficult to do with all these et and you know big bag, because you will have to know more walters data you'll have to.
Amit Kothari: model them in our PD so supporting multiple sources and Plus we have disconnected which was many of those sources are not even supported or non Oracle specific so that leads to a lot of this complex modeling problems.
Amit Kothari: The biggest other biggest problem is performance right so typically uses me so suppose the data is now you rated and stage in a data warehouse because the data warehouse is a relational database.
Amit Kothari: The the query performance is very, very sub optimal you know you have to have so for even for our data sets so typically now customers have.
Amit Kothari: In the data warehouse hundreds and millions of rows in the fact tables in a right, so when you're joining them.
Amit Kothari: We see a lot of issues with very performant the query the dashboards takes like 30 to four minute 30 to 40 minutes to render even the prom performances of a very slow, just to select the filter it takes like a minute or two minute to refresh.
Amit Kothari: So this This creates a lot of frustration with the the user right because they just have they're constantly now waiting mode so.
Amit Kothari: So this is so large volume and and performance is the key for analytics.
Amit Kothari: Adoption so.
Amit Kothari: So this so that's why what what what they have done is is to query up for fun just to make faster performance here to go on creating.
Amit Kothari: aggregate tables at multiple levels so This also leads to our data latency problem right, because now we are to go right etfs to now do more and more and more aggregation of data just to make the report perform faster, so this leads to higher higher maintenance and the CEO.
Amit Kothari: And the tech stack also is very, very complex, so we have a separate tool for atl separate tool for Meta data, the admin tool.
Amit Kothari: You know, and then, and then you have the visualization here, so all three requires expertise, you know consultants, or you know people with different expertise.
Amit Kothari: And so, and and all of all of them, so the basically the because the two landscape is so complicated with different different so now they have different different.
Amit Kothari: Problems going on with this so.
Amit Kothari: So that is one of the most important point here that the tech stack is also very complicated and complex and takes takes a lot of expertise, just to maintain that and upgrade that.
Amit Kothari: To the maintenance with in kota is where we have solved all this problem is timely insights what so basically when you when you migrate out of.
Amit Kothari: Our menu migrate to in quarter, because we don't have to do this data reshaping that's one of our biggest usp.
Amit Kothari: is basically we just get the data, as is in the third normal form, so now your earpiece has all this huge number of tables in third normal form ready just bring them, as is you just.
Amit Kothari: So you just joined them there right so so so there's no data or very, very little data reshaping so so now because of that we.
Amit Kothari: Our references are very fast, because we are not yet is writing very of we are sending very optimized extract various to the database so just.
Amit Kothari: Just like single table queries so they so that's why you have very low data latency, so now we can do 30 minutes refreshes.
Amit Kothari: And right and we all the other thing is, we also don't aggregate the data we what we do is we bring in the detailed data, and that is aggregated at runtime, so this is one of our biggest.
Amit Kothari: or USB offer in corner, where.
Amit Kothari: We have this timely insights right where we can do near real time reporting.
Amit Kothari: So that's that's the first point now.
Amit Kothari: Because because we just because we don't have this heavy admin to the only thing we have is basically basically we.
Amit Kothari: First of all, we don't have separate rooms, so all of all of our all of the thing is done in our in the browser itself, so what you do is you just bring in the tables and he just joined them.
Amit Kothari: So, and then there is just a one time thing right because, because we are not, and the only time you need to change will be when you're adding new tables so.
Amit Kothari: So it's very easy to model data, so if so typically what people do is they bring in cite the source table has a see 200 columns so they bring in all the different content they just expose a subset of it.
Amit Kothari: Right so so basically the data is already there, and you just need anything now you just need more columns you can just added directly to the dashboard.
Amit Kothari: At the high performance, so we have appropriately direct data mapping technology so that lead, that is one of the reason why we don't have to reshape the data and we can give.
Amit Kothari: Support performance, you know, without without reshaping or react aggregate data, all of the data is in memory and and basically the joins joins our pre computed and the data is loaded into memory so that's why the dashboards.
Amit Kothari: performance is in.
Amit Kothari: Sub seconds so and another thing is this grading scale scale very well so suppose, even if they're finding million rows we have customers having tables with 2 billion rows $3 million and they, and they also are in a scale very well so that's one of the biggest.
Amit Kothari: biggest.
Amit Kothari: feature features here real seamlessly so so since since we don't have this we don't have a pre aggregate data we bring in all of the detailed data aggregation is at runtime so we can now easily from from aggregated aggregated insight to detail level transaction.
Amit Kothari: So this is one of the the kind of a slide which talks about the modern day tax architecture which is, which is what is basically being.
Amit Kothari: You know the new thing now is basically I have, I have my various data rooms, so I have my bronze layer and my silver and gold and this raw data zone is basically is is basically, I suppose I have my file and it's mostly was a big in all of this raw data to my data.
Amit Kothari: Right and then I go on doing this further refinement right now, so I then we reshape the data into a data warehouse I aggregate data, and then I make specific data marts just to serve my various.
Amit Kothari: Users right, so I have finance users supply chain users, so now so by the time you read the business data zone.
Amit Kothari: The biggest problem is now you have lost lost sight of the detail transaction data, because now.
Amit Kothari: Very much aggregate level your last lineage security is a big problem, because now, you have now.
Amit Kothari: Basically, of reshape the data so and plus all this heavy ETA layers where.
Amit Kothari: You know you're basically replacing replicating the data, so you are first first replicating the source data into raw data zone, then you again replicating the data into refined and then you're further refining in your business needs zone.
Amit Kothari: Which leads to basically where where you can only only refresh like two times, or three times a day or less to other problems.
Amit Kothari: So in quarter, in contrast, right because we don't do this city shaping what we do is we bring in the data directly from the sources right, so we do pair pattern pattern.
Amit Kothari: Data extracts the map directly to source so suppose I have 50 tables in source you directly ingest the 50 tables we just joined them up so basically you are creating those those tables.
Amit Kothari: itself right so there's no data reshaping and and so you can think of it as like as and then we create this business schemas which are you can think of is like a virtual data warehouse where you can have business schema with your you know various.
Amit Kothari: dimensions and metrics right but, but all of the aggregation and refinement happens at a logical level, so we are not persisting that so, which is what gives us the power to high performance and this near real time information right so.
Amit Kothari: And the other biggest thing is customers really users really want to look at the detail transaction data, so we already have that.
Amit Kothari: Right, so now, you can easily surface that that we can control from you can aggregate hundreds of millions of rows and then you can drill to the details so that's.
Amit Kothari: that's one of one of the biggest advantage of this one this direct data mapping technology and this parallel extracts because we don't suppose.
Amit Kothari: Because we don't reshape we can go fast extraction and those extracted data goes into the park a format, which is a column new storage, which is the alluded to memory so.
Amit Kothari: So we basically skip all this.
Amit Kothari: You know there's refined data zones and businesses data zones, all of this happens to us at a logical level right.
Amit Kothari: so on.
Amit Kothari: So this one kind of gives you an idea of the difference between incorporate in your traditional.
Amit Kothari: Data warehousing So if you look at that the implementations of data versus take a long, long time right, even with the delivery solution with.
Amit Kothari: You just to customize the details to identify the new you know the various customers with.
Amit Kothari: Because the, the problem with that at Edison data warehouses right that it has his facts and dimensions have to be created.
Amit Kothari: To answer specific questions right so whenever now something changes, where you want to ask a new question.
Amit Kothari: You have to now customize and create new facts and dimensions, so that then you have to go through staging layer staging to data warehouse to do all this detail work.
Amit Kothari: So that that makes your ethically the development and time to basically produce a report for business, a long time.
Amit Kothari: But encoder right we just identify the data sources right we just get the so what So if you look at the right.
Amit Kothari: Suppose imma imma EBS customer so Kelly causes of EBS customers, but if you look at it, each one of the customers has kind of a different way.
Amit Kothari: To measure their business right metrics are different so so basically if you just bring in the tables and have, and so it becomes more of a giant right where the you don't have to do so much customizations right you because the source tables are the same.
Amit Kothari: it's only only thing what you do is you just create formula columns which are specific to your business so that's why it's much so, the implementations and time to basically surface data and your dashboard is four to seven days it's, so much so much faster.
Amit Kothari: Right and then and because we have all the all the data right, you can answer any any kind of business questions, it says, you know it's not that Eric now craft a separate solution by you know, by creating this etfs and all that.
Amit Kothari: So this is, this is what I need to return right it's basically the tend to create a business value is much, much faster.
Amit Kothari: So you know, we have seen a lot of business frustration, where they take like it takes basically six you know six to eight weeks to deliver a simple field, because there's so much things in order compared to you know basically one one day or even half a day, just to bring up.
Amit Kothari: expose that field in a quarter, so the tend to value is much faster.
Amit Kothari: So this is also kind of the things what I which kind of says, or you know what I was talking about is basically because we have all the transaction Guild leaders, we can easily drill into detail performance is great.
Amit Kothari: The adding of new data sources, because we are a unified data platform, and we have hundreds of connectors we can easily ingest the data from it and from new sources and then join them to existing sources and then enter them in the same dashboard.
Amit Kothari: Compared to you know the Oracle bi where you have to do all this etfs and you know there's so much development work just to move data and model that and getting accurate results, so you know, because we are not reshaping data no there's no data loss happening.
Amit Kothari: So other big thing which are in quarter focuses on is self service, so we have this business friendly structure called business schema, which is a presentation layer
Amit Kothari: So we have the physical schema which has the tables and then it create a nice business friendly Structural business chemo.
Amit Kothari: and basically debts and then businesses just use that to create their own dashboard so that we move.
Amit Kothari: From our manager reporting architecture to more of a self service like picture right because you know we don't want it to just create thousands and thousands of report and.
Amit Kothari: manage them, you know we it just creates some of the big gold reports, and then users can start looking at those and you know it's going to use self service to add and delete.
Amit Kothari: Things they want.
Amit Kothari: So this is just an example of one one use case which we did for a customer a big customer billion dollar company for account receivable aging So although we a solution right we had this heavy etfs and.
Amit Kothari: Basically.
Amit Kothari: If you look at the atl it was taking 70 plus minutes task he was.
Amit Kothari: And then, also the snapshot in traders lead, leading to a lot of data escalation, because they wanted as of data reporting so every day you're taking.
Amit Kothari: snapshots of millions of rows so the tables had like hundreds and hundreds of millions of rows which, which was making the report very, very slow.
Amit Kothari: So encoder comes in right where we where we do, where we you know we we have a blueprint ready vs blueprint we just deploy that which is quickly do the aging.
Amit Kothari: Because we don't have to reshape the data and we have a concept of sparse snapshot in where we don't snapshot you don't have to take daily snapshots.
Amit Kothari: So this was so another performance and data refreshes started happening extremely further ado 96 offices today, and this was important in the closing, so this is one of the most important reports for closing.
Amit Kothari: Another example is you know this this just happened a few weeks back where there was a proof of value for a big electrical contractor, so they like to build a billion dollar company with massive employees and in a big presence across the US.
Amit Kothari: So basically so they were on a.
Amit Kothari: yc on EBS data and they were having the same problem they the new reports were taking.
Amit Kothari: Close to eight plus weeks weeks.
Amit Kothari: There, to read heavy dlc data refresh was taking a long time to 18 hours for full load and three hours for incremental so you can only do so many differences, a day.
Amit Kothari: They had lot, there was a PC was very high because of so many consultants European employee.
Amit Kothari: So this was the problem and then included as a pov where within.
Amit Kothari: Two weeks effort and we could be deployed our ABS blueprint, and you know basically all of those complex reports.
Amit Kothari: You know we're basically we have the business cables and the cables and we can just build them quickly so just you know, basically, the pros and and and.
Amit Kothari: How the customer was so so impressed that they can adapt they basically sign they they were very impressed with the pov and they.
Amit Kothari: signed the contract, because now, you have just one single platform and not be shipping, the data, and I can leverage my blueprint and basically start start delivering the report, so we could deliver all this, whatever they want within four days, so it was one of the big things here.
Amit Kothari: This was another fortune 50 media company, where we completely replace their financials and supply chain, you know bi within six weeks so.
Amit Kothari: It was 96 data requests a day in a completely we you know we completely refresh their basically their etfs and star schema.
Amit Kothari: So that that was another another big.
Amit Kothari: Big win for us and and the other thing is, they are so the customer is really, really happy, in the sense that they are able to do so many faster freshness and they able to.
Amit Kothari: You know, do.
Amit Kothari: You know, with with less consultants they're able to get it we use business needs so that's one of the things.
Amit Kothari: This at a high level of product comparison of the various you know, would you know, be a yc and in quarter there which I kind of return, it is the time to inside self service unified technology and augmented analytics so we have.
Amit Kothari: One of the big thing is in documenting analytic space right we have this.
Amit Kothari: Basically, if you're organized also is being machine learning, so we have.
Amit Kothari: it's going to pull the full support of that, so we have notebooks and Python and spark that you can do ml on directly.
Amit Kothari: On top and that actually a lot of customers like that, because the 80% or any ml project is in data prep so since the data is already there, and you can easily flattened out which really easy to now do ml ml.
Amit Kothari: Work right.
Amit Kothari: So now, let me talk about the blueprints which provides this big tech stack so we have our blueprint for various sources earpiece like netsuite salesforce and then we have EBS know the IP.
Amit Kothari: Oracle cloud up ASAP so this la rebuilt analytics on various financial supply chain HR projects with.
Amit Kothari: You know, we basically rebuild physical schemas dashboards and business schemas so typically customers take those and within.
Amit Kothari: We have seen around 75 to 80% fit and then what they do is they just customize the business schemas for their own.
Amit Kothari: metrics and i'll give you our own business processes like procure to pay order to cash, so you know and they haven't built for ways customers, so they actually address the various metrics which they want.
Amit Kothari: This is kind of a high level content coverage, which we have on the blueprints and we are constantly adding to our blueprints so we have full financials coverage supply chain human resources projects sales.
Amit Kothari: Planning everything on that.
Amit Kothari: yeah this is kind of the same thing this.
Amit Kothari: Is.
Amit Kothari: The various the blueprint models here.
Amit Kothari: This one, this one is kind of shows the richness of our blueprint, so this is just one area accountable and this is just a subset of the metrics which we have.
Amit Kothari: By by each area so holds effectiveness employee supply, we have all of this matrix and what customers do is they will take those and start.
Amit Kothari: customizing their trade so basically they have they have something and then they can start adding their desktop to that.
Amit Kothari: And then we have dashboards which is going to surfaces all this data.
Amit Kothari: This one is kind of gives you a complexity of what we're talking about here, so this is one dashboard query plan for the procure to pay dashboard.
Amit Kothari: So, if you look at all those tables like on the blue tables Rachel they're all directly the source data, so, as I said right, we have no data data or via very little data reshaping like nine.
Amit Kothari: So basically 95% data is not reshaped so they're all this data we're just joined them and then because.
Amit Kothari: Now, and then what happens is this query plan is dynamic in the sense that, depending on what phase, you have added.
Amit Kothari: In the dashboard you gotta figure out the path to connect all these tables so suppose I have a subset of the tables, then the query plan will be will be totally different than this.
Amit Kothari: So, depending on what you need in quarter will bring in bring in those tables enjoys directly in the dashboard so this one report was performing in sub sections on hundreds of millions of rows and it has like more than 30 table so.
Amit Kothari: So.
Amit Kothari: So this is this is kind of another thing is, when I have two sources, this is what one of a few of our customers are doing, where they're where they're EBS and then they're moving to Oracle yet peak load but they're going waiting.
Amit Kothari: modulator time, so they are first implemented general ledger in the cloud so they want to now drill from GL data infusion to EBS to sub ledger in EBS and they all want to do it in the same dashboard so all of this can be done in our platform very easily.
Amit Kothari: So this is what I was talking about, so when you're migrating from CBS to clutter up that also produces lot of challenges, because the tech stack is totally different then now how do I.
Amit Kothari: How because because i'm going, what are your time, how do I really have a need to have surface both my legacy data and my mother or the new data, so the that property, for you know, has a lot of challenges with the Boise.
Amit Kothari: tech stack so in encoder we can have we can just get all this data, he cloudier PBS in the same platform and we can have the same dashboard we can show you can have a union of data, you can show both the legacy data, and you know, the new data so it's much more.
Amit Kothari: Your migration becomes very simple.
Amit Kothari: Okay, so I think now i'll hand it over to Alex to to give you a DEMO on that so and it's great.
Alex Stark: bye ahead and share my screen now.
Alex Stark: And it can you see that.
Amit Kothari: yeah again.
Alex Stark: awesome see i'm going to walk you guys through a kind of a high level DEMO of the Platform today not something extremely detailed but.
Alex Stark: kind of walk you through, for instance, you know how the schemas look and quarter how we would build a fresh dashboard.
Alex Stark: off of that data i'm going to show you an example of the performance of that data by building a report off of a 500 million record table.
Alex Stark: And then, lastly, I want to show you guys the Oracle blueprint and show you some of the pre built content that we've packaged for you, free of charge, so.
Alex Stark: When you first log into a quarter, you know, this is our front end you'll log in and see all the dashboards and various reports that you've built on this content page when you log in.
Alex Stark: So we are in full and then platform we don't just bring in the data and query on it, but we do have a full visualization layer where you can build very nice dashboards to visualize that data.
Alex Stark: And, and you know answer all those business questions that you have, but in order to get to this endpoint we first have to connect the data and build schemas so i'm going to go to the schema tab.
Alex Stark: You know, in this case we have many schemas already built in this DEMO environment that schema just represents all the tables that you decided to bring from that data source and how you've organized it so we've got several data sources here.
Alex Stark: i'm specifically i'd like to take a look in accounts receivable this one has a small subset of the full accounts receivable schema that we have in our Oracle blueprint.
Alex Stark: So in this case only has 14 tables and 31 joins and at the top right i've got a diagram view I can look at to really visualize what that data looks like.
Alex Stark: kind of like the slide that I showed you earlier.
Alex Stark: So this shows you all the tables, not just in this schema but also the other schemas that the data has been joined to.
Alex Stark: We support joining together separate data sources so in this case we've got our accounts receivable join to count data and opportunity data from salesforce.
Alex Stark: information from jira service desk so you're able to build a consolidator reports off of multiple sources, at the same time, but primarily we're going to focus on this accounts receivable Oracle data.
Alex Stark: So, for instance on my base table that i'm going to use for my example build is going to be customer transaction lines all.
Alex Stark: So that's kind of all the Roger was actual data we're going to build a high level summary of that information which then down the line you could also build a drill down to see full detailed information.
Alex Stark: And so, in order to start building a dashboard we can just click on explore while looking at accounts receivable and immediately began building our first inside of that information.
Alex Stark: So it's a very simple drag and drop process point click you can pick the visualizations you'd like to see the pie charts bar charts line charts.
Alex Stark: In my case i'll just do a pivot table since those can be rather a computation intensive we're going to build a lot of aggregates all have a large 500 million record table.
Alex Stark: So i'm going to do that, and then I just need to bring in rows columns and measures so i'm going to drag and drop order quantity.
Alex Stark: invoice and revenue amount.
Alex Stark: And then, from my rose, I want to see this by sales channel.
Alex Stark: As well as by another field called party type while i'm here i'd like to do some filtering so i'm going to take away a couple items I don't care to see in this particular report.
Alex Stark: And then search for my next field.
Alex Stark: And then for my columns to complete this pivot table i'm going to look at this information as a monthly breakdown of sales by you know channel in our day so i'm going to bring in month name.
Alex Stark: And then sort that on month number.
Alex Stark: And now that we've populated all the required fields, a row column and measure you can already see a preview has been generated this just uses a small subset of about 15 million records just show you what your visual will look like.
Alex Stark: And i'm going to go ahead and then save this insight, so we can pull the full 500 million records set.
Alex Stark: So i'm going to save that.
Alex Stark: The dashboard to save it into this case i'll just go anyone.
Alex Stark: And now it has started the full query and just like that it took only a second it just query the full 500 million records, plus the other tables that were part of that join and if you're curious what other tables were involved, we can always go back to edit that and say.
Alex Stark: Take a look at one of our measures and pull up the query plan.
Alex Stark: So this one's a pretty simple example not as complicated as the one i'm Michel in the slideshow, but we have five different tables involved with the base table of 500 million records and all that information was joined in queried and presented in an aggregated result in only a second.
Alex Stark: And the beauty of building those out who gets off of an aggregated you know rod transaction level detail is that we get that full visibility into abroad transaction detail information.
Alex Stark: So if I wanted to see that I could build another insight here, where I just pull in the full transaction table, and I can browse through and look at the whole entire set of records so i'm going to go ahead and build a table with everything and customer transaction lines for them.
Alex Stark: And they'll see that.
Alex Stark: And at this point we can immediately start interacting with our data, so instead of looking at all 470 million records, maybe I want to focus only on consumer.
Alex Stark: electronics, I can click on that it'll automatically filter on that information and narrow down the table at the bottom to only look at that particular information which, in our case, we have 8766 records.
Alex Stark: Now, in addition to building bash words off of schemas which represent the actual physical data that you've ingested into the importer platform.
Alex Stark: And I say that ingestion because we do make a full copy of that data we don't clear directly on the source, whenever we run our dashboards we bring in a full copy of the data.
Alex Stark: We set up incremental loads that you can then every five minutes or so update your data and have a near real time dashboard.
Alex Stark: But in addition to the schemas we also have business schemas, so this is our semantic layer
Alex Stark: where you can build curated data sets of data sets with multiple data sources in them to provide to those users, that may not understand how those joins work or how the.
Alex Stark: What the table names are what the field names are you can rename fields and, of course, build business logic formulas calculations and save this in these business schema so that you can then publish out to the users for that self service capability.
Alex Stark: So hopefully That was a good example of how easy it is to build a dashboard from scratch and important, but now, since we're talking horrible today.
Alex Stark: i'm going to switch over to my other instance where I have the full Oracle blueprint installed.
Alex Stark: So as on it said, we have a whole set of content built in here very robust content, especially in financials and supply chain.
Alex Stark: And so i'm going to start with schemas like I did before, and show you just how complex some of this data is.
Alex Stark: So, for instance, the fall accounts receivable schema which I previously showed you a subset of it actually has 33 tables and 151 joints because of all the other schemas.
Alex Stark: That are involved in join in creating for reports off of your data potentially So if I go to the diagram for this, you can see those 33 tables plus many other tables that are being pulled from the other schemas that we've created off of that EBS data.
Alex Stark: So many tables in fact that.
Alex Stark: You can't even see the names on these tables in this complex diagram until I zoom in.
Alex Stark: But there's 61 tables, as well as other objects we've created such as materialized views, where we have created.
Alex Stark: And replicated the business logic that exists at Oracle so that the reports, you get out of in court match the type of reporting that you would normally get out of you know, always see or will be it.
Alex Stark: Likewise, we have a bunch of business games built, so you know, even if you don't want to use the existing dashboards that template for customization we can use.
Alex Stark: The other you know business schemas we provided off of that Oracle data, so you can build your own custom dashboards from scratch.
Alex Stark: And finally let's just take a look at some of the content, we have here because there's a lot of good use cases that you can enable they're using our blueprint.
Alex Stark: So, for instance, we have very nice supply chain dashboards if I go here or management, I could look at this backlog booking backlog and billing dashboard.
Alex Stark: And it gives you lots of information you'll summaries of you know your booking backlog by various attributes, you know backward items specific detailed information on that on that data.
Alex Stark: And that's just the first tab you got shipping tab.
Alex Stark: where you can look at various shipping metrics and just like I showed you earlier, our dashboards are interactive So if I wanted to focus on a specific item category.
Alex Stark: Such as miscellaneous I can click on that and that particular chart now drills down to specific items within that category, and now you're looking at customers and items, you know, for that category, on the left.
Alex Stark: Another good use cases in our financials so you know we give you full drill down from GL to sub ledger.
Alex Stark: So if I wanted to go to this journals to ar and looking at a high level GL summary of my information, and then I could go down into my journal details.
Alex Stark: And I can click on the header ID and we have a drill down to another dashboard where we can then look at specific invoices and transactions underneath that particular category under that particular header ID.
Alex Stark: We also have a lot of customers using you know this dash just the blueprint to really expedite you know things like.
Alex Stark: You know, closing at the end of the quarter monthly you know we can go in and we have a reconciliation dashboard so under GL reconciliation.
Alex Stark: That they are account summary.
Alex Stark: You can see you know information from the GL and then excellent tables, you can see the variances and so people are able to drill directly into specific.
Alex Stark: journal, you know entries and specific transactions and identify what these variances are, and it really speeds up and expedites.
Alex Stark: That you know end a month or end of quarter closing process all you'd have to do if you want to see more detail on something is click on the account number.
Alex Stark: drill down to that, and now you can see, specific ar account mismatches and then identify what what information needs to be corrected as part of your closing process.
Alex Stark: So it's a very nice set of content, you know lots of value, and this is all free of charge, this is just based on our expertise and Oracle will be a lot of customers Oracle SAP salesforce workday and we build these blueprints because you know.
Alex Stark: Most of the time 80% of that those tables that data is going to match, no matter who's using Oracle right, and then we give you this blueprint to accelerate your reporting process to where.
Alex Stark: You can focus more of your energy on what makes you unique what's customer your business will customer information is in your EBS or other Snr other VIP system.
Alex Stark: So there's a lot of value here is blueprints so that concludes my DEMO i'm going to hand it back over to emit for the Q amp a we have any questions we'll go ahead and answer them.
Amit Kothari: Thanks Alex.
Amit Kothari: As a reminder, please type your questions into the Q amp a box and we'll address them live.
Amit Kothari: So the questions we have is how do we support data snapshots so we have full support of a snapshot in data we have a dense snapshots and Spar snapshots.
Amit Kothari: So dense snapshot is your regular snapshot, but I can do a daily weekly quarterly you know snapshots of the data and then I can surface them and then it's.
Amit Kothari: very, very simple to create snapshots and then so we, we have a Community article on that, and then you can surface that data in.
Amit Kothari: Our dashboard and the spot snapshot is where I can recreate that picture as of a certain date, so we have special functions called last version, so I don't have to take daily snapshot so.
Amit Kothari: sparse optional it's a really great solution for when you have the data size is huge right, so you don't want it escalation, so I can recreate the data as of a certain day and we can see the snapshot.
Amit Kothari: The other is how do we support Oracle EBS data in constellation between GL and the sub ledger, so I think that was one DEMO which Alex showed, so we have the GL and the sub ledger is it is sub ledger is like API projects.
Amit Kothari: And we can go from journals that we have on all the dashboards and content to support that so and then you can do the reconciliation between your your sub ledger tables and GL and you know you can find out where the discrepancies are.
Amit Kothari: Any other questions you guys, have you can just type it here and we can.
Amit Kothari: You know, we can try to answer those questions.
Amit Kothari: Okay.
Amit Kothari: Any more questions.
Amit Kothari: A couple of minutes to ask any questions.
Amit Kothari: that's all the questions we have today oh there's one question they caught, can we have the recorded session okay yeah they're.
Amit Kothari: Different lighting the recorded session will be posted on our Community right.
Alex Stark: yep and I think everyone will also be the email with that link sometime after the webinar ends.
Amit Kothari: yeah.
Amit Kothari: Yes.
Amit Kothari: Yes, right Okay, so any any other questions.
Amit Kothari: Okay, I think that's all we had so please join me in thanking Alex for.
Amit Kothari: sharing their time and expertise with us and don't forget to check out in kota cloud during quarter.com you can just go there and basically.
Amit Kothari: I just have a trial trial cluster and then you can even have the blueprints also there you can start basically creating a blueprint and basically looking at the blueprint and loading the data, so please check us out there.
Amit Kothari: The link is in the chat thanks for joining us and have a fantastic day.
Hosted by:
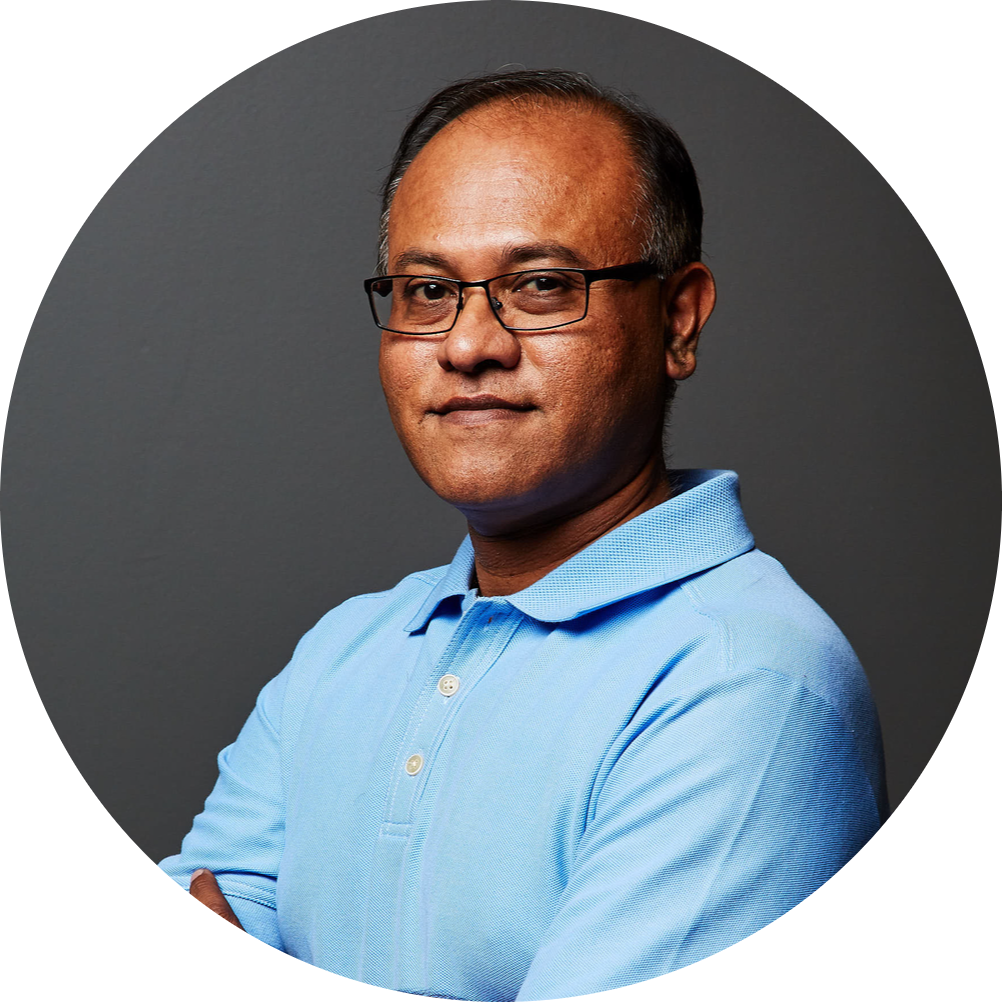
Amit Kothari
Senior Director, Solutions Engineering
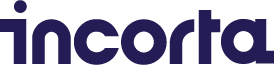
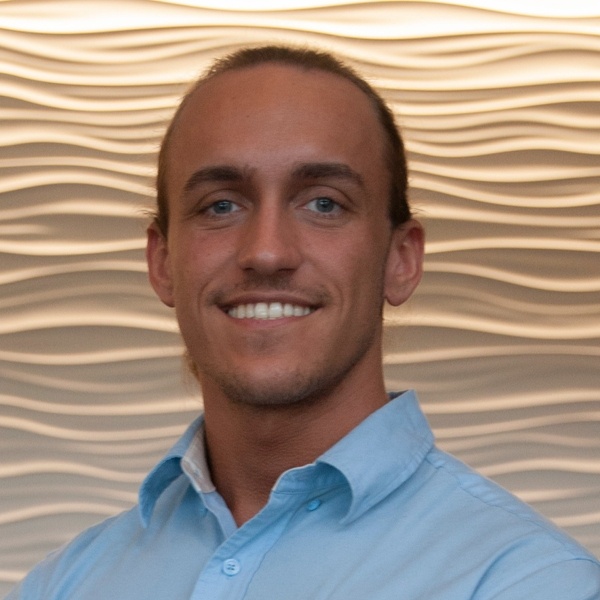
Alex Stark
Senior Sales Engineer